Stagewise Training with Exponentially Growing Training Sets
IEEE Transactions on Neural Networks and Learning Systems(2024)
Key words
Training,Optimization,Computational complexity,Upper bound,Approximation algorithms,Training data,Risk minimization,Gradient hard thresholding (GHT) algorithms,proximal algorithms,stagewise training strategy,stochastic optimization
AI Read Science
Must-Reading Tree
Example
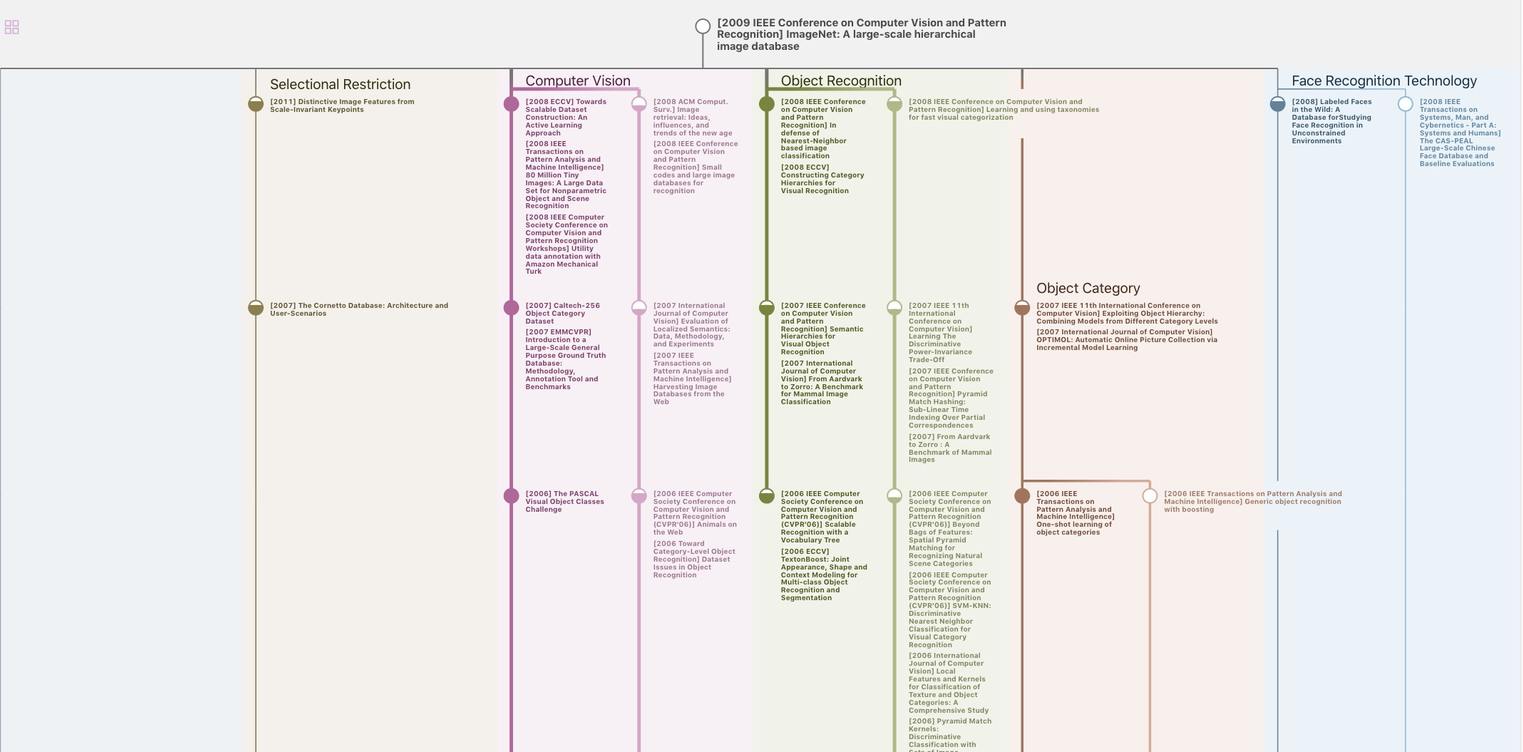
Generate MRT to find the research sequence of this paper
Chat Paper
Summary is being generated by the instructions you defined