Geometric Deep Learning-Guided Suzuki Reaction Conditions Assessment for Applications in Medicinal Chemistry
RSC medicinal chemistry(2024)
摘要
Suzuki cross-coupling reactions are considered a valuable tool for constructing carbon-carbon bonds in small molecule drug discovery. However, the synthesis of chemical matter often represents a time-consuming and labour-intensive bottleneck. We demonstrate how machine learning methods trained on high-throughput experimentation (HTE) data can be leveraged to enable fast reaction condition selection for novel coupling partners. We show that the trained models support chemists in determining suitable catalyst-solvent-base combinations for individual transformations including an evaluation of the need for HTE screening. We introduce an algorithm for designing 96-well plates optimized towards reaction yields and discuss the model performance of zero- and few-shot machine learning. The best-performing machine learning model achieved a three-category classification accuracy of 76.3% (+/- 0.2%) and an F1-score for a binary classification of 79.1% (+/- 0.9%). Validation on eight reactions revealed a receiver operating characteristic (ROC) curve (AUC) value of 0.82 (+/- 0.07) for few-shot machine learning. On the other hand, zero-shot machine learning models achieved a mean ROC-AUC value of 0.63 (+/- 0.16). This study positively advocates the application of few-shot machine learning-guided reaction condition selection for HTE campaigns in medicinal chemistry and highlights practical applications as well as challenges associated with zero-shot machine learning. Machine learning-predicted screening plate.
更多查看译文
AI 理解论文
溯源树
样例
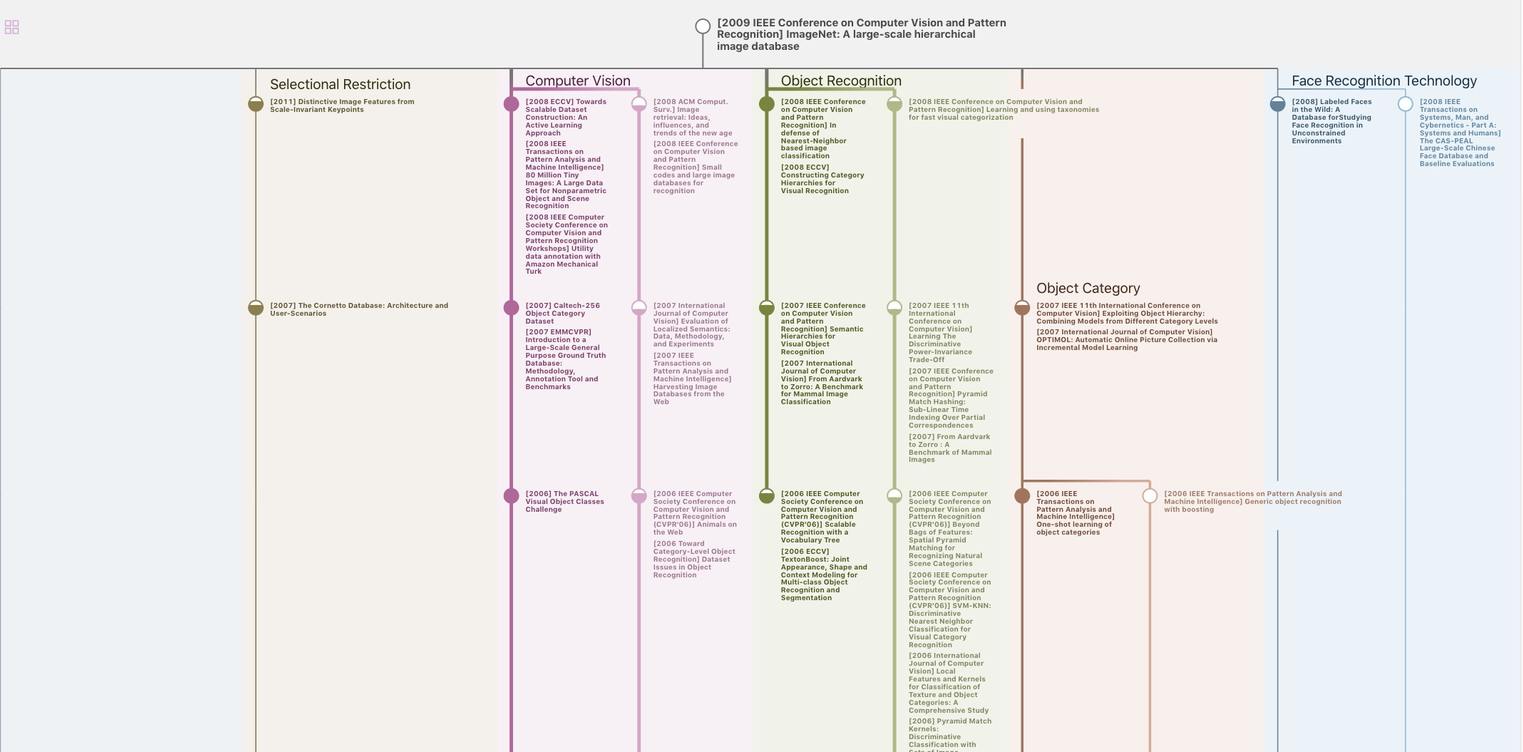
生成溯源树,研究论文发展脉络
Chat Paper
正在生成论文摘要