FeatureLTE: Learning to Estimate Feature Importance
Proceedings of the ACM on Management of Data(2024)
摘要
Feature importance scores (FIS) estimation is an important problem in many data-intensive applications. Traditional approaches can be divided into two types; model-specific methods and model-agnostic methods. In this work, we present FeatureLTE, a novel learning-based approach to FIS estimation. For the first time, as we demonstrate through extensive experiments, it is possible to build general-purpose pre-trained models for FIS estimation. Therefore, FIS estimation reduces to prediction outputs from a pre-trained FeatureLTE model. Pre-trained FeatureLTE models enjoy several desired advantages, including accuracy, robustness, efficiency, and evolvability, and FeatureLTE models really begin to shine on large datasets where traditional methods often find themselves unable to scale. We build our pre-trained models for binary classification and regression problems using observations from nearly 1,000 public datasets. We systematically evaluate various design choices of FeatureLTE model construction and carefully design meta features to make sure that they are computationally lightweight. Based on our evaluation, FeatureLTE is on par with the best existing FIS estimators in terms of FIS quality, and achieves up to 339.48x speedup without sacrificing the quality of FIS estimates on large-scale datasets. Finally, we release two pre-trained FeatureLTE models for binary classification and regression problems that are ready to use on almost all tabular datasets, along with the repository of 701 binary classification datasets and 256 regression datasets with pre-computed feature importance scores to promote future research along this direction.
更多查看译文
关键词
feature importance estimation,feature selection,meta learning
AI 理解论文
溯源树
样例
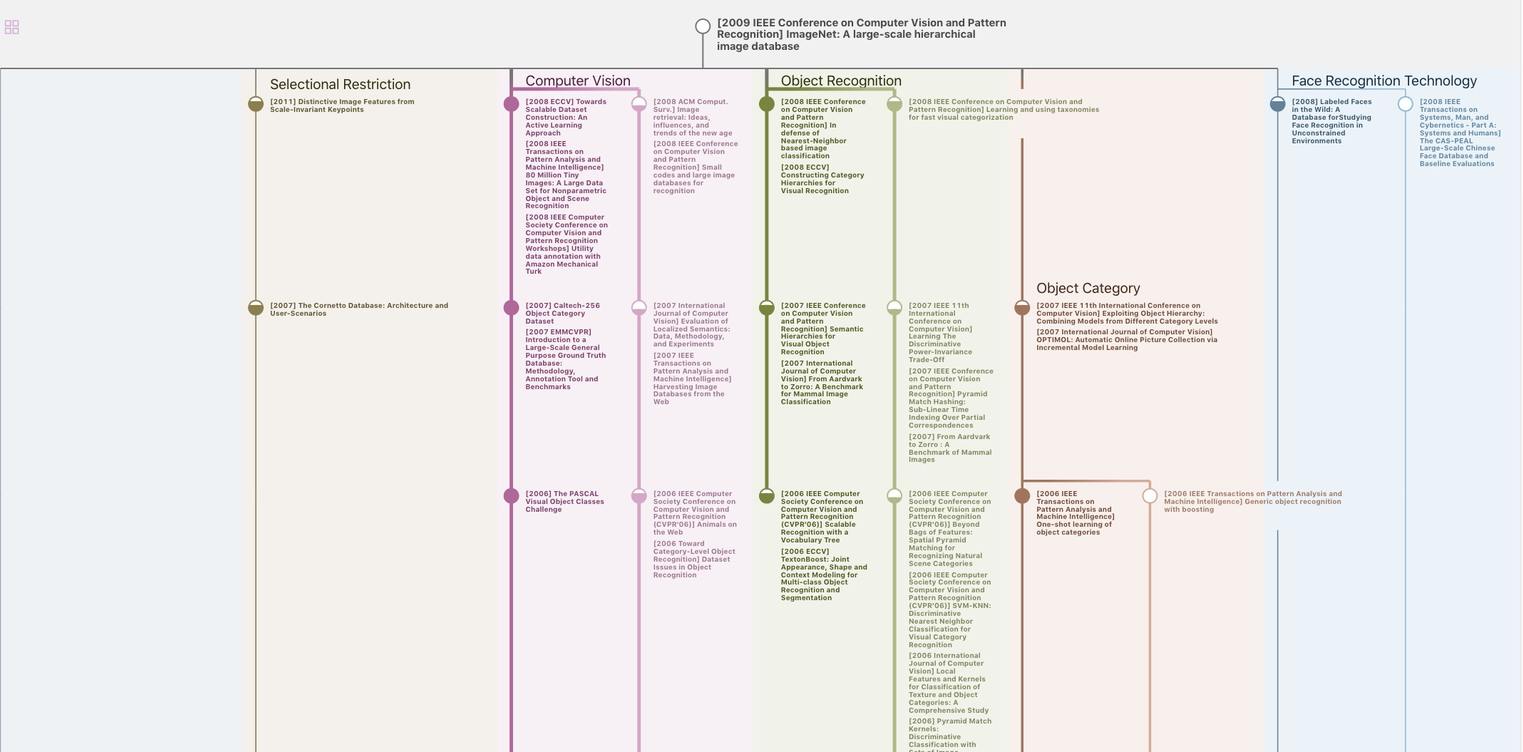
生成溯源树,研究论文发展脉络
Chat Paper
正在生成论文摘要