Complexity of Zeroth- and First-order Stochastic Trust-Region Algorithms
CoRR(2024)
摘要
Model update (MU) and candidate evaluation (CE) are classical steps
incorporated inside many stochastic trust-region (TR) algorithms. The sampling
effort exerted within these steps, often decided with the aim of controlling
model error, largely determines a stochastic TR algorithm's sample complexity.
Given that MU and CE are amenable to variance reduction, we investigate the
effect of incorporating common random numbers (CRN) within MU and CE on
complexity. Using ASTRO and ASTRO-DF as prototype first-order and zeroth-order
families of algorithms, we demonstrate that CRN's effectiveness leads to a
range of complexities depending on sample-path regularity and the oracle order.
For instance, we find that in first-order oracle settings with smooth sample
paths, CRN's effect is pronounced – ASTRO with CRN achieves
Õ(ϵ^-2) a.s. sample complexity compared to
Õ(ϵ^-6) a.s. in the generic no-CRN setting. By contrast,
CRN's effect is muted when the sample paths are not Lipschitz, with the sample
complexity improving from Õ(ϵ^-6) a.s. to
Õ(ϵ^-5) and Õ(ϵ^-4) a.s. in the zeroth-
and first-order settings, respectively. Since our results imply that
improvements in complexity are largely inherited from generic aspects of
variance reduction, e.g., finite-differencing for zeroth-order settings and
sample-path smoothness for first-order settings within MU, we anticipate
similar trends in other contexts.
更多查看译文
AI 理解论文
溯源树
样例
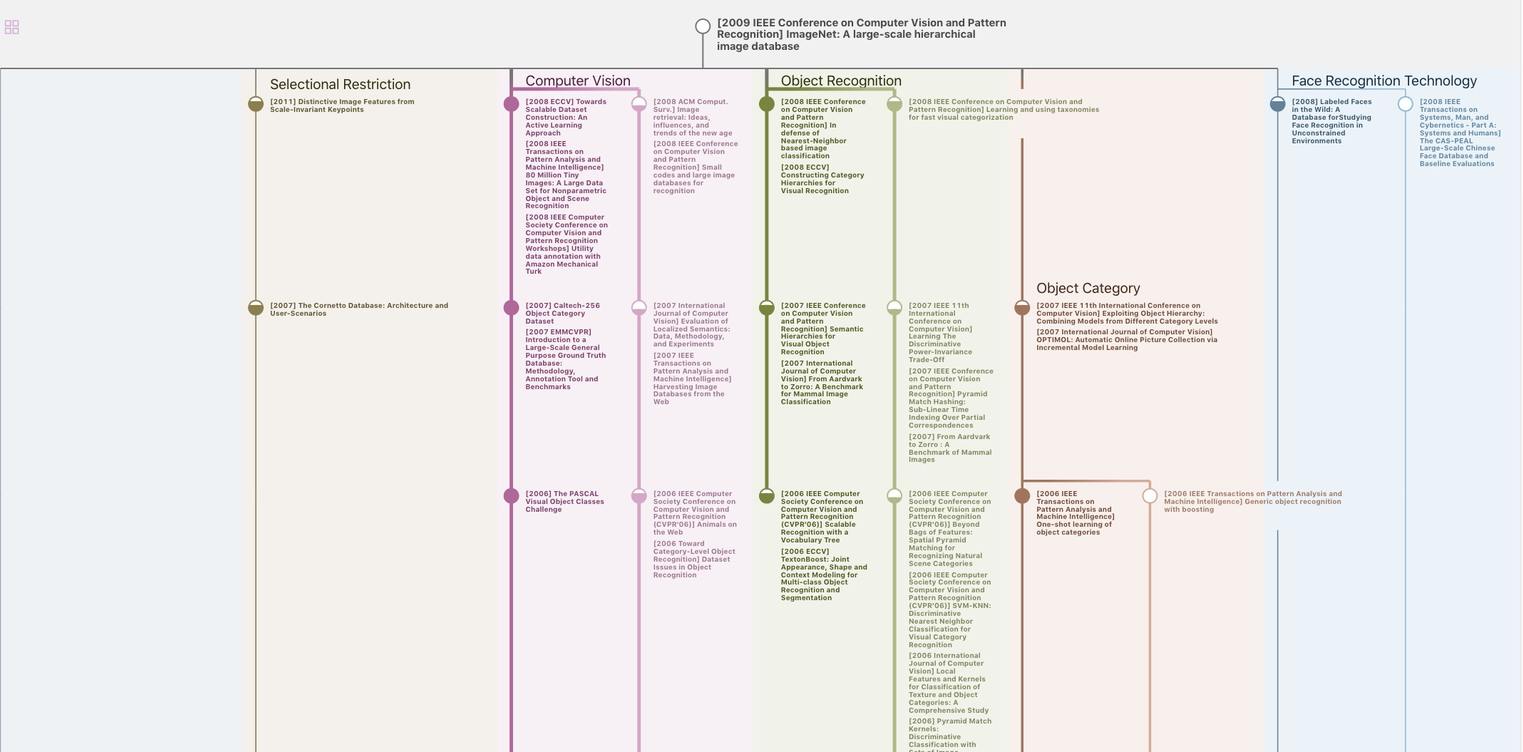
生成溯源树,研究论文发展脉络
Chat Paper
正在生成论文摘要