Leveraging Dynamic and Heterogeneous Workload Knowledge to Boost the Performance of Index Advisors
Proceedings of the VLDB Endowment(2024)
摘要
Current index advisors often struggle to balance efficiency and effectiveness when dealing with workload shifts. This arises from ignorance of the continual similarity and distant variety in workloads. This paper proposes a novel learning-based index advisor called BALANCE, which boosts indexing performance by leveraging knowledge obtained from dynamic and heterogeneous workloads. Our approach consists of three components. First, we build separate Lightweight Index Advisors (LIAs) on sequential chunks of similar workloads, where each LIA is trained with a small batch of workloads drawn from the chunk, and it provides direct index recommendations for all workloads in the same chunk. Second, we perform a policy transfer mechanism by adapting the LIA's index selection strategy from historical knowledge, substantially reducing the training overhead. Third, we employ a self-supervised contrastive learning method to provide an off-the-shelf workload representation, enabling the LIA to generate more accurate index recommendations. Extensive experiments across various benchmarks demonstrate that BALANCE improves the state-of-the-art learning-based index advisor, SWIRL, by 10.03% while reducing training overhead by 35.70% on average.
更多查看译文
AI 理解论文
溯源树
样例
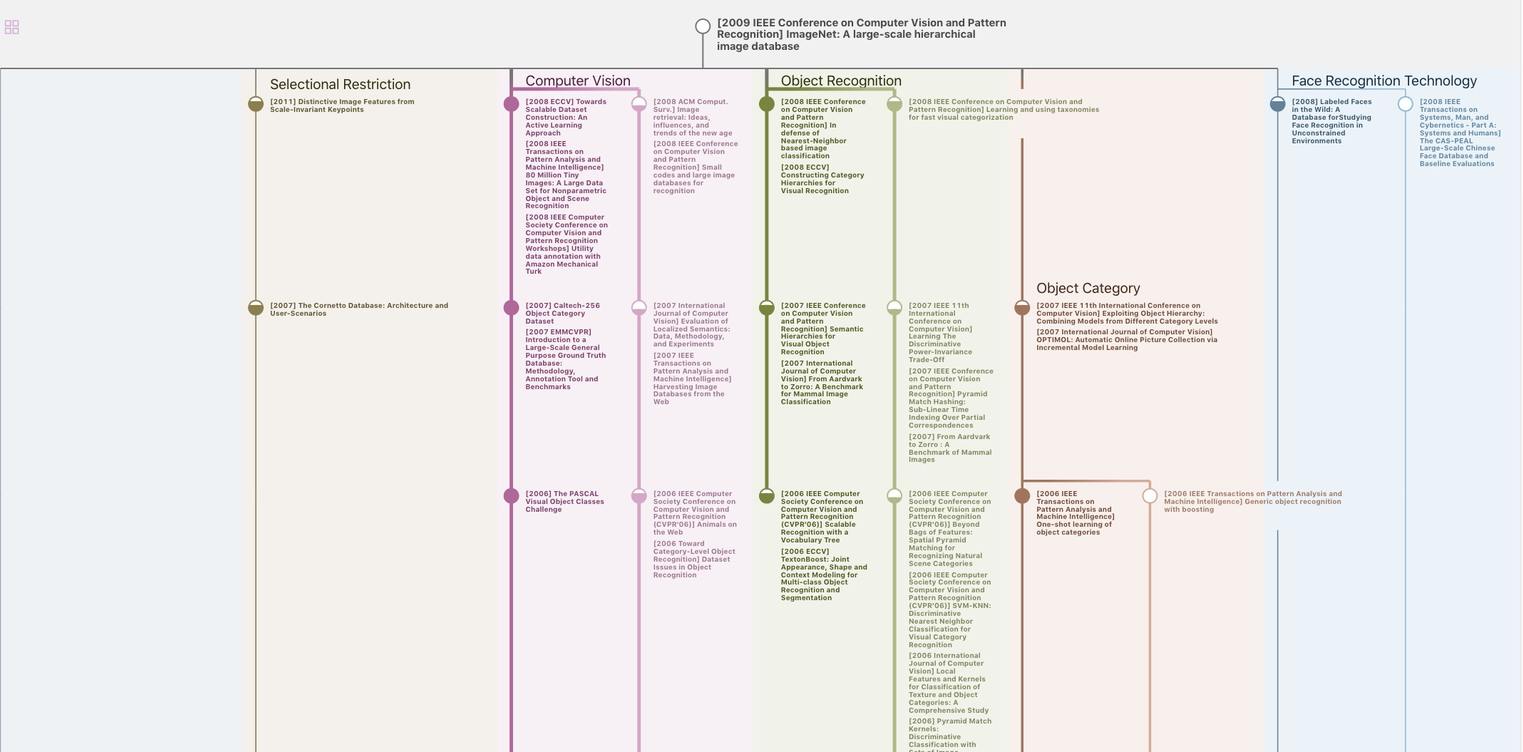
生成溯源树,研究论文发展脉络
Chat Paper
正在生成论文摘要