Detection of Venous Thromboembolism Using Recurrent Neural Networks with Time-Series Data
Asia Conference on Algorithms, Computing and Machine Learning(2024)
摘要
Machine Learning (ML) has been widely applied to medical science for decades. As common knowledge, the progress of many diseases is often chronic and dynamic. Longitudinal data, or time-series data, has better descriptions of certain diseases, as it naturally captures the dynamic over time. Venous thromboembolism (VTE), as a regular complication of cancer tumour treatments, has been emphasized in many studies. Recent research has shown that ML algorithms incredibly contribute to VTE risk estimation and prediction. However, existing methods rarely consider the dynamic changes during the development of VTE, and often make decisions based on time-point data, such as the latest blood test results of patients. In this paper, we propose a VTE detection model that takes advantage of capturing dynamic information using time-series data. The model consists of a Recurrent Neural Network (RNN) based framework with time embedding and Attention mechanism to encode longitudinal features of patients. We test our proposed model on a real-world dataset that contains multiple times of blood test results of patients together with other clinical features. The network is compared with several commonly used ML models in previous VTE studies. The results show that our model outperforms all baseline models, and achieved a state-of-art performance with 80.0% accuracy and 0.881 AUC.
更多查看译文
AI 理解论文
溯源树
样例
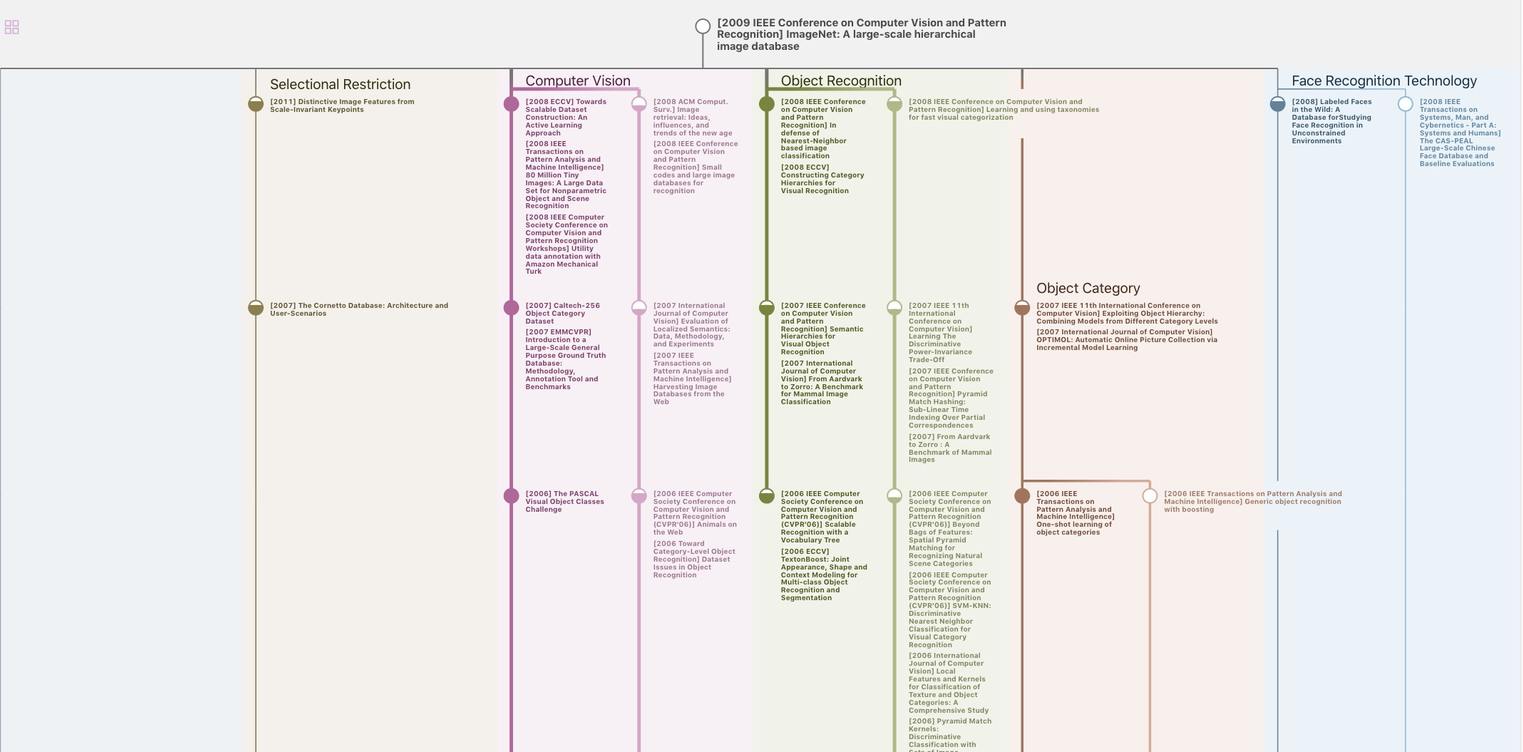
生成溯源树,研究论文发展脉络
Chat Paper
正在生成论文摘要