AI-generated synthetic clinical-genomic data for precision oncology research: Validation using a case study on lung adenocarcinoma.
Journal of Clinical Oncology(2024)
摘要
e13627 Background: The analysis of genomic variants is crucial in precision oncology research, offering insights into cancer risks and progression, especially in diverse types such as lung adenocarcinoma (LUAD). However, such research often grapples with balancing patient privacy with the need for comprehensive, high-quality genomic datasets. Our project addresses this by creating synthetic clinical-genomic data, which maintains patient confidentiality and provides a rich resource for genomic cancer research. Methods: Leveraging the GuardantINFORM database, which includes anonymized genomic data and structured payer claims, we focused on generating synthetic data for LUAD patient cohorts. This approach involves processing real patient data into a format compatible with Medisyn’s generative AI models, ensuring the synthetic data retains the original's statistical properties, and processing the output back into the original database structure and format. This method plays a crucial role in maintaining patient privacy and serves as a valuable tool for research by enabling the generation of realistic patients with desired properties on demand. Results: Our synthetic data closely mirrors real-world genomic and claims variable distributions, evidenced by a 0.994 R2 correlation between real and synthetic data along with comparable Oncoprints. Importantly, privacy tests show that patient confidentiality is effectively maintained despite this effective performance. The synthetic data's utility was then demonstrated in a study replicating real-world findings: LUAD patients with KRAS G12C in combination with STK11 mutations showed a significantly higher risk of early mortality. This underscores the potential of synthetic data in advancing cancer research. Conclusions: This research offers a promising avenue for the cancer research community. By providing a method to share privatized, synthetic genomic data, which can be combined and generated on demand, we enable broader, more responsible data sharing. This approach protects patient privacy and offers a rich dataset for groundbreaking research, potentially accelerating advances in cancer diagnosis and treatment. [Table: see text]
更多查看译文
AI 理解论文
溯源树
样例
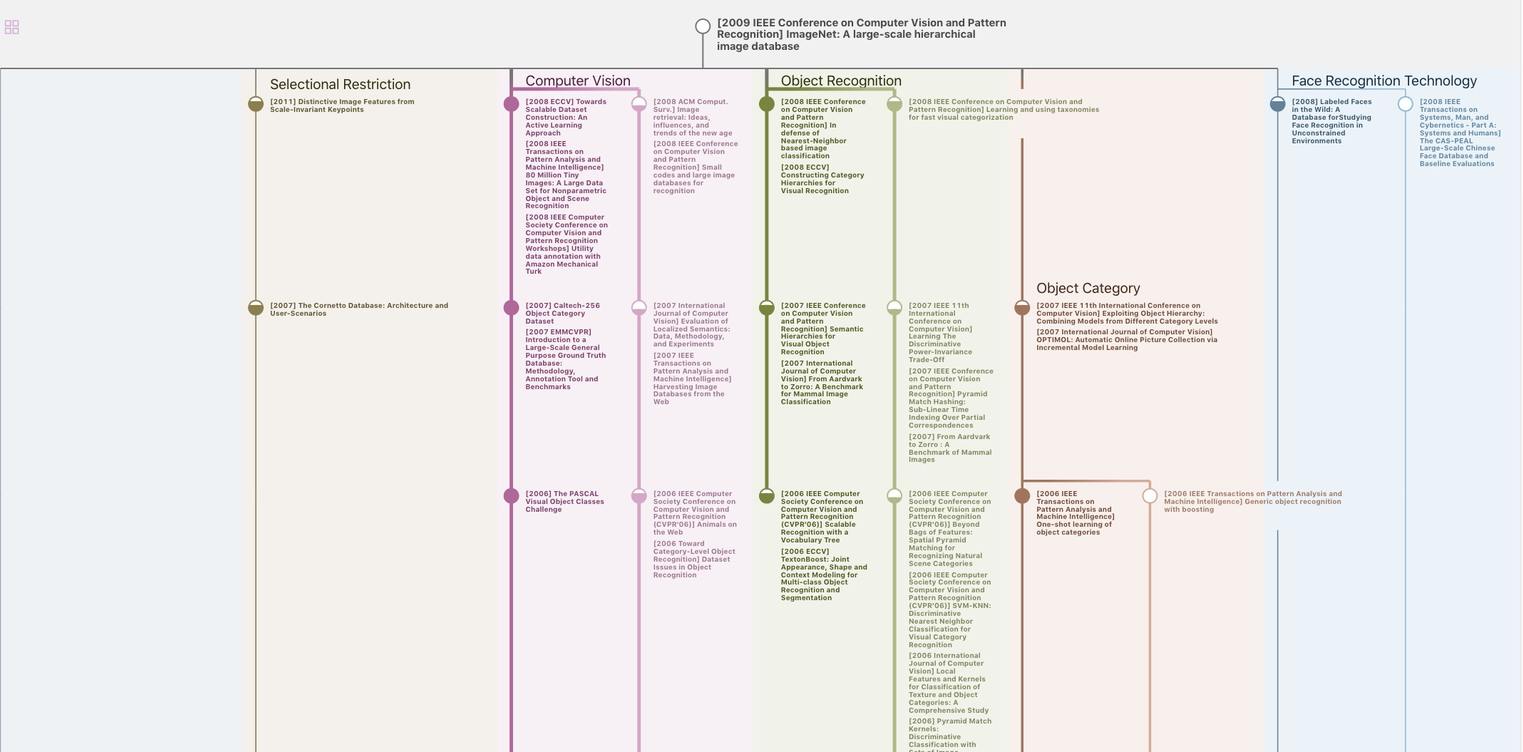
生成溯源树,研究论文发展脉络
Chat Paper
正在生成论文摘要