Reliable Edge Machine Learning Hardware for Scientific Applications
2024 IEEE 42ND VLSI TEST SYMPOSIUM, VTS 2024(2024)
摘要
Extreme data rate scientific experiments create massive amounts of data that require efficient ML edge processing. This leads to unique validation challenges for VLSI implementations of ML algorithms: enabling bit-accurate functional simulations for performance validation in experimental software frameworks, verifying those ML models are robust under extreme quantization and pruning, and enabling ultra-fine-grained model inspection for efficient fault tolerance. We discuss approaches to developing and validating reliable algorithms at the scientific edge under such strict latency, resource, power, and area requirements in extreme experimental environments. We study metrics for developing robust algorithms, present preliminary results and mitigation strategies, and conclude with an outlook of these and future directions of research towards the longer-term goal of developing autonomous scientific experimentation methods for accelerated scientific discovery.
更多查看译文
关键词
real-time systems,neural networks,digital simulation,reliability assesment,VLSI,VLSI testing
AI 理解论文
溯源树
样例
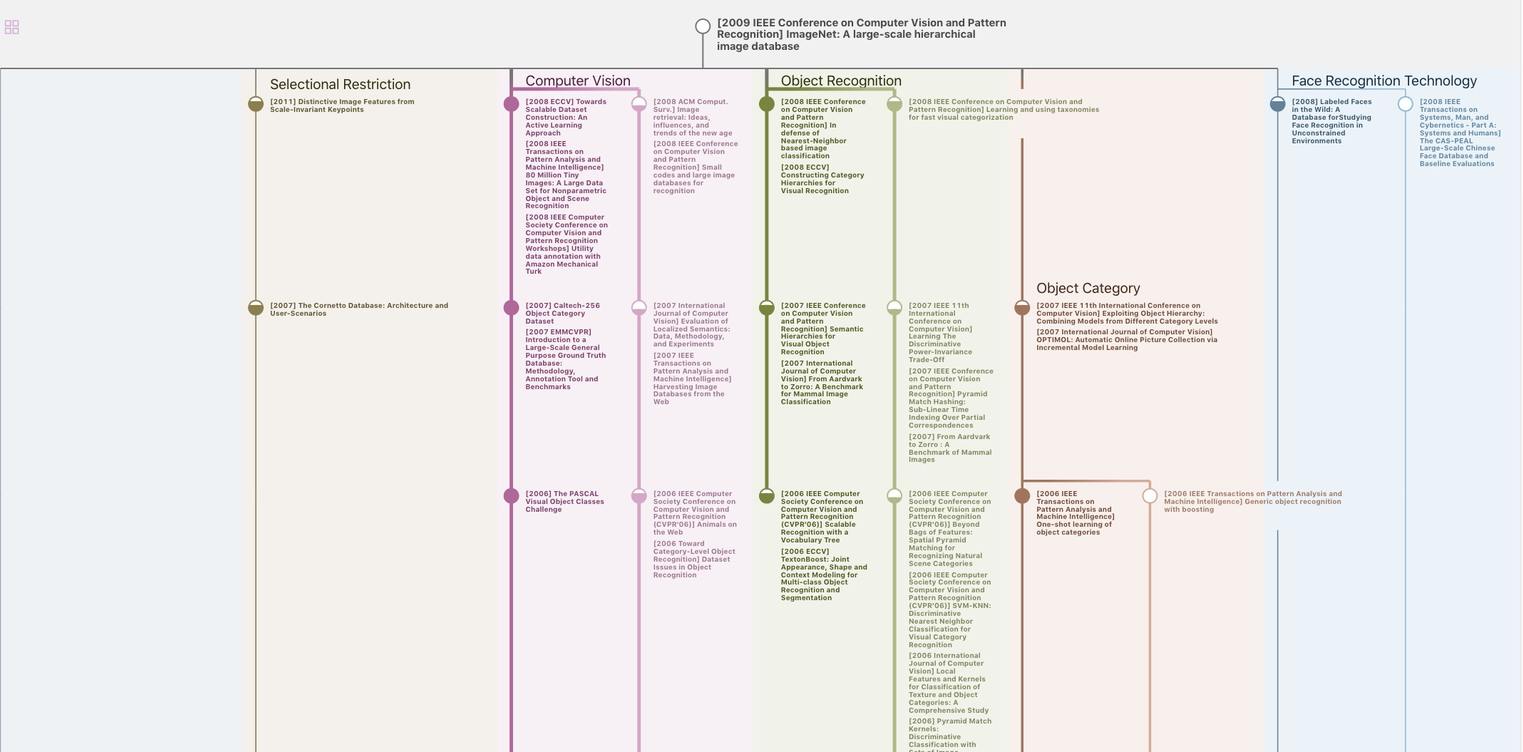
生成溯源树,研究论文发展脉络
Chat Paper
正在生成论文摘要