Multi-source Connectivity As the Driver of Solar Wind Variability in the Heliosphere
Stephanie L. Yardley,David H. Brooks,Raffaella D'Amicis,Christopher J. Owen,David M. Long, Deb Baker,Pascal Demoulin,Mathew J. Owens,Mike Lockwood,Teodora Mihailescu,Jesse T. Coburn,Ryan M. Dewey,Daniel Muller,Gabriel H. H. Suen,Nawin Ngampoopun,Philippe Louarn,Stefano Livi, Sue Lepri,Andrzej Fludra,Margit Haberreiter,Udo Schuhle NATURE ASTRONOMY(2024)
AI 理解论文
溯源树
样例
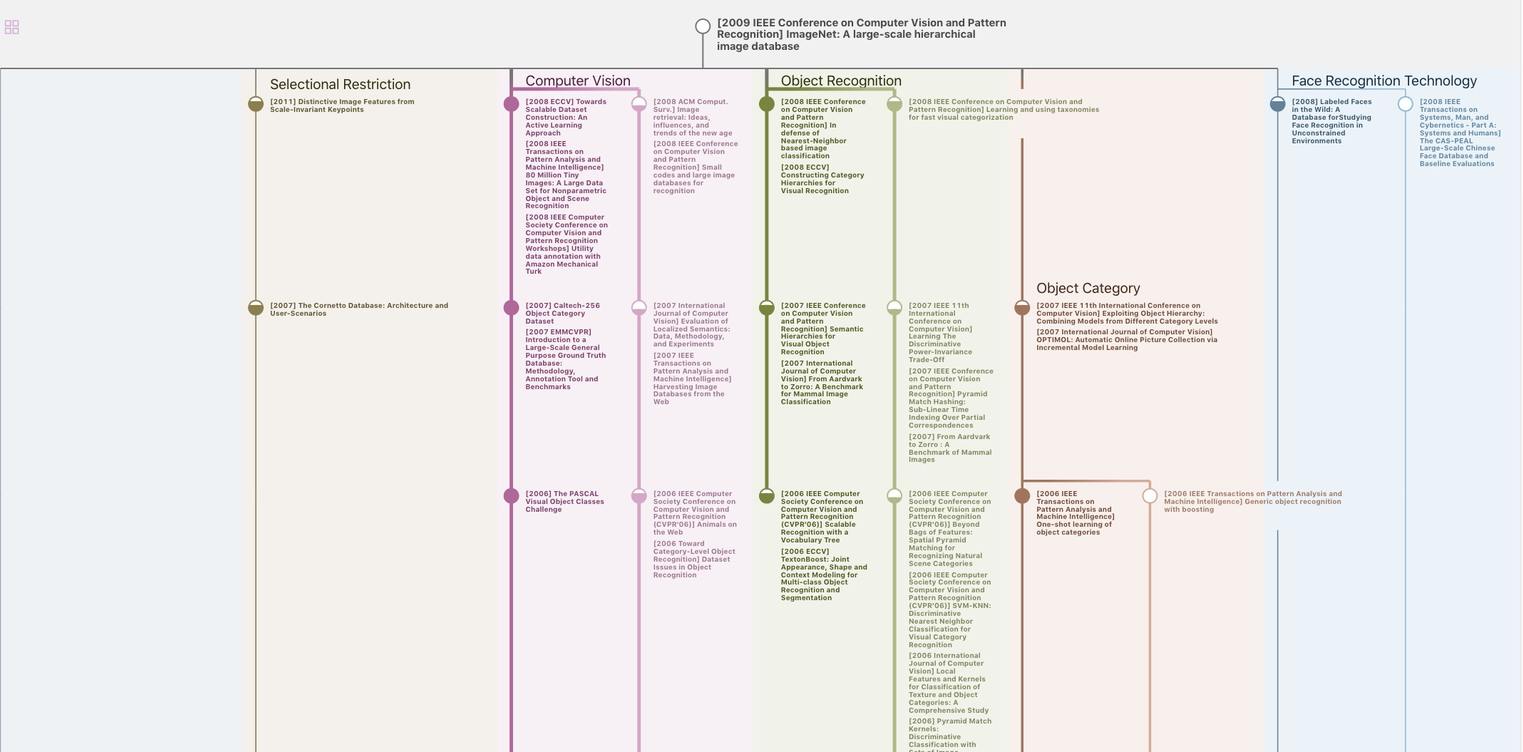