Optimal Scheduling Strategy of Electricity and Thermal Energy Storage Based on Soft Actor-Critic Reinforcement Learning Approach
JOURNAL OF ENERGY STORAGE(2024)
关键词
Energy storage management,Reinforcement learning,Soft actor-critic,Community-scale microgrids,Optimal schedule
AI 理解论文
溯源树
样例
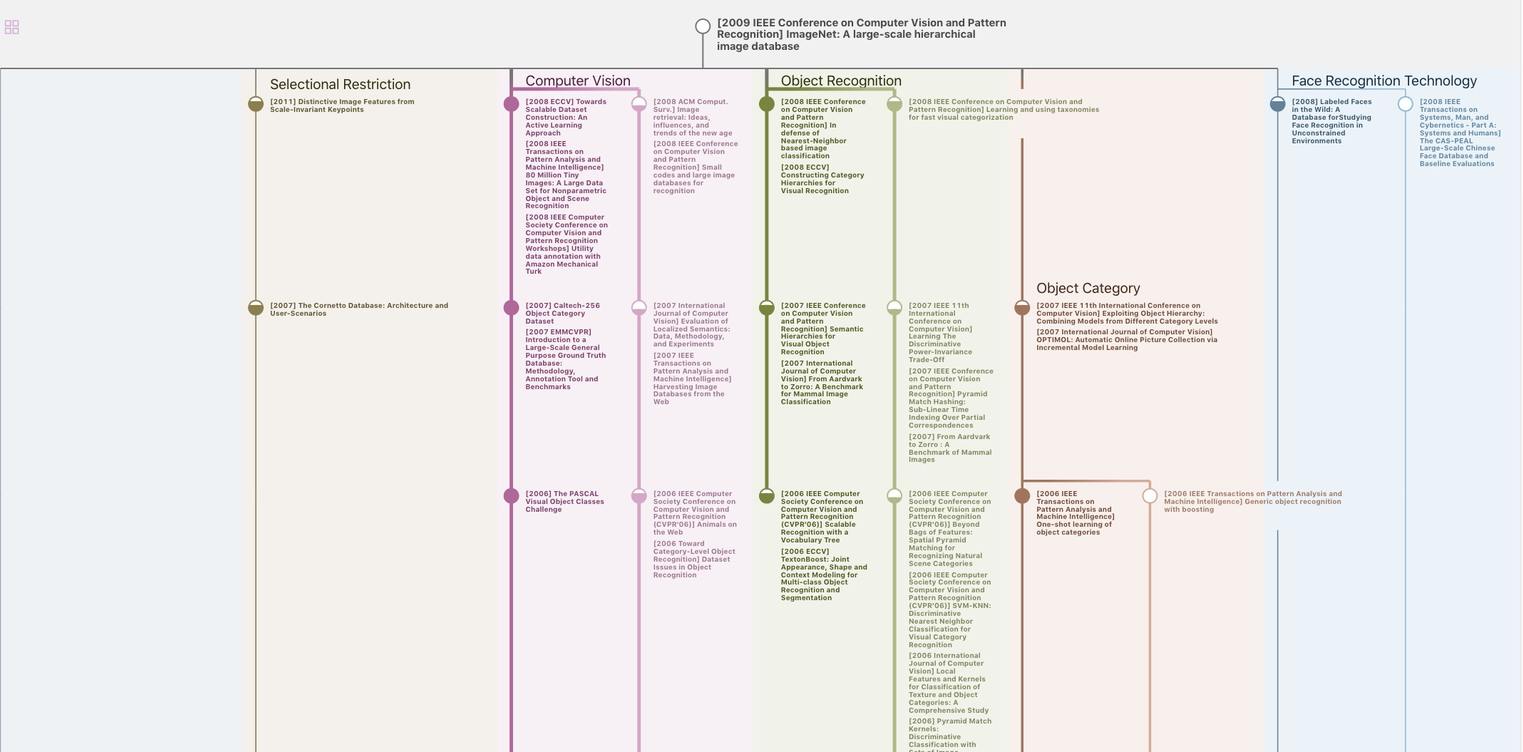
生成溯源树,研究论文发展脉络
Chat Paper
正在生成论文摘要