Fault Diagnosis of Industrial Robot Based on Multi-Source Data Fusion and Channel Attention Convolutional Neural Networks
IEEE access(2024)
摘要
Industrial robots are prone to failure due to harsh working environments, which affects movement accuracy. The fault diagnosis of industrial robots has become an indispensable part of robot collaborative maintenance in intelligent manufacturing. Most existing diagnostic methods only use a single data source, and the diagnostic accuracy will be affected due to signal acquisition errors and noise interference. This paper proposes a multi-source data fusion and channel attention convolutional neural network (MD-CA-CNN) for fault diagnosis of multi-joint industrial robots. The network takes the time domain data and time-frequency domain data of the vibration signal, torque signal, and current signal of the six joints of the robot as input. Then, we realize the diagnosis of the faults by using a Softmax Classifier layer after the two parts of feature extraction and feature fusion. In addition, a channel attention mechanism is developed. It acts on the two parts of feature extraction and feature fusion, respectively. It assigns weights to different source data and weights to time-domain and time-frequency domain features. Finally, we established a test bench to compare the proposed method with the deep learning algorithm that only uses multi-data source fusion, the deep learning algorithm that only uses a single data source, and the commonly used machine learning algorithm. The results show that the MD-CA-CNN model proposed in this paper has the highest accuracy and stability, reaching 95.8% +/- similar to 0.39 %, which verifies the method's effectiveness.
更多查看译文
关键词
Industrial robots,fault diagnosis,multi-source data fusion,convolutional neural networks,channel attention mechanisms
AI 理解论文
溯源树
样例
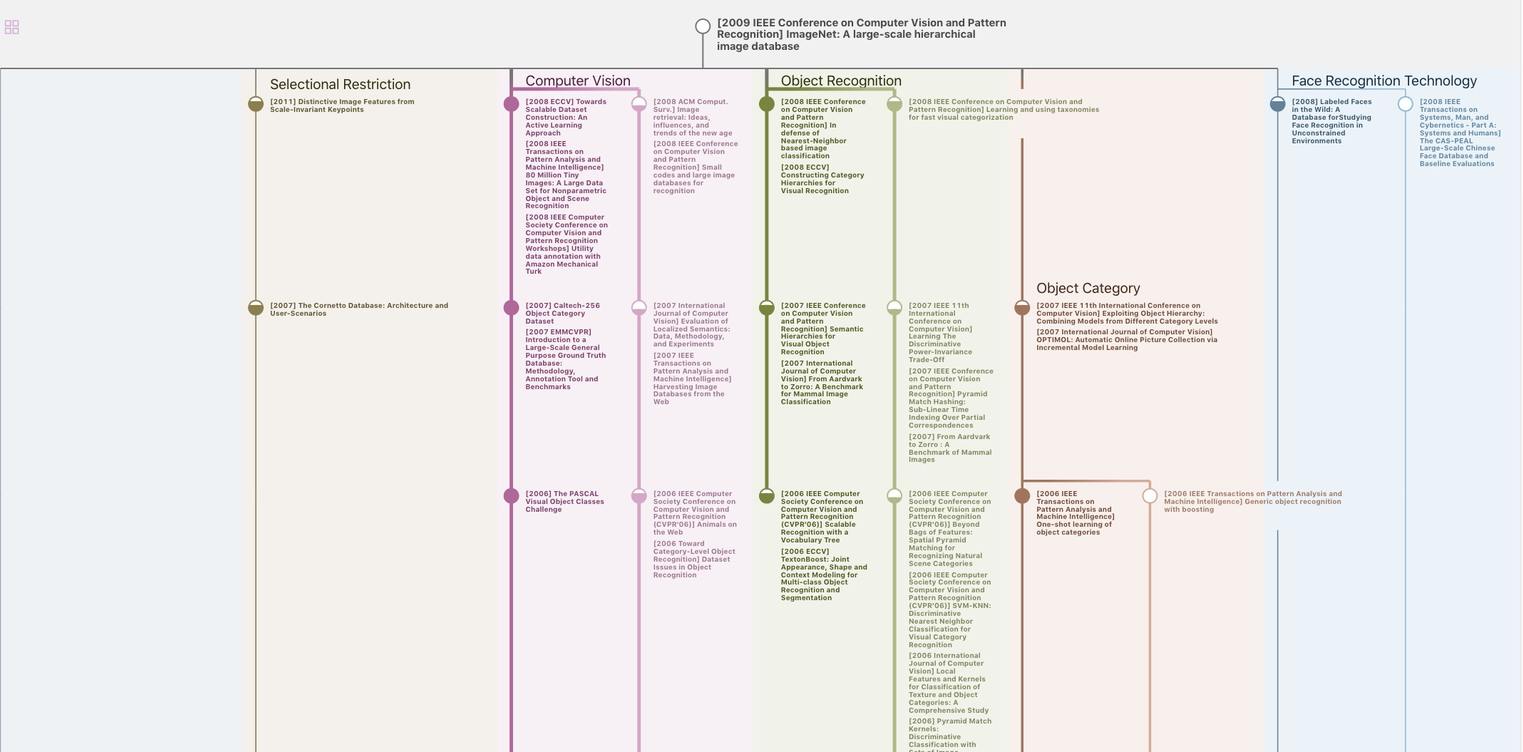
生成溯源树,研究论文发展脉络
Chat Paper
正在生成论文摘要