Efficient Visual Fault Detection for Freight Train Via Neural Architecture Search with Data Volume Robustness
IEEE TRANSACTIONS ON INDUSTRIAL INFORMATICS(2024)
摘要
Deep learning-based fault detection methods have achieved significantsuccess. In visual fault detection of freight trains, there exists a largecharacteristic difference between inter-class components (scale variance) butintra-class on the contrary, which entails scale-awareness for detectors.Moreover, the design of task-specific networks heavily relies on humanexpertise. As a consequence, neural architecture search (NAS) that automatesthe model design process gains considerable attention because of its promisingperformance. However, NAS is computationally intensive due to the large searchspace and huge data volume. In this work, we propose an efficient NAS-basedframework for visual fault detection of freight trains to search for thetask-specific detection head with capacities of multi-scale representation.First, we design a scale-aware search space for discovering an effectivereceptive field in the head. Second, we explore the robustness of data volumeto reduce search costs based on the specifically designed search space, and anovel sharing strategy is proposed to reduce memory and further improve searchefficiency. Extensive experimental results demonstrate the effectiveness of ourmethod with data volume robustness, which achieves 46.8 and 47.9 mAP on theBottom View and Side View datasets, respectively. Our framework outperforms thestate-of-the-art approaches and linearly decreases the search costs withreduced data volumes.
更多查看译文
关键词
Date volume robustness,fault detection,freight trains,neural architecture search (NAS),sharing
AI 理解论文
溯源树
样例
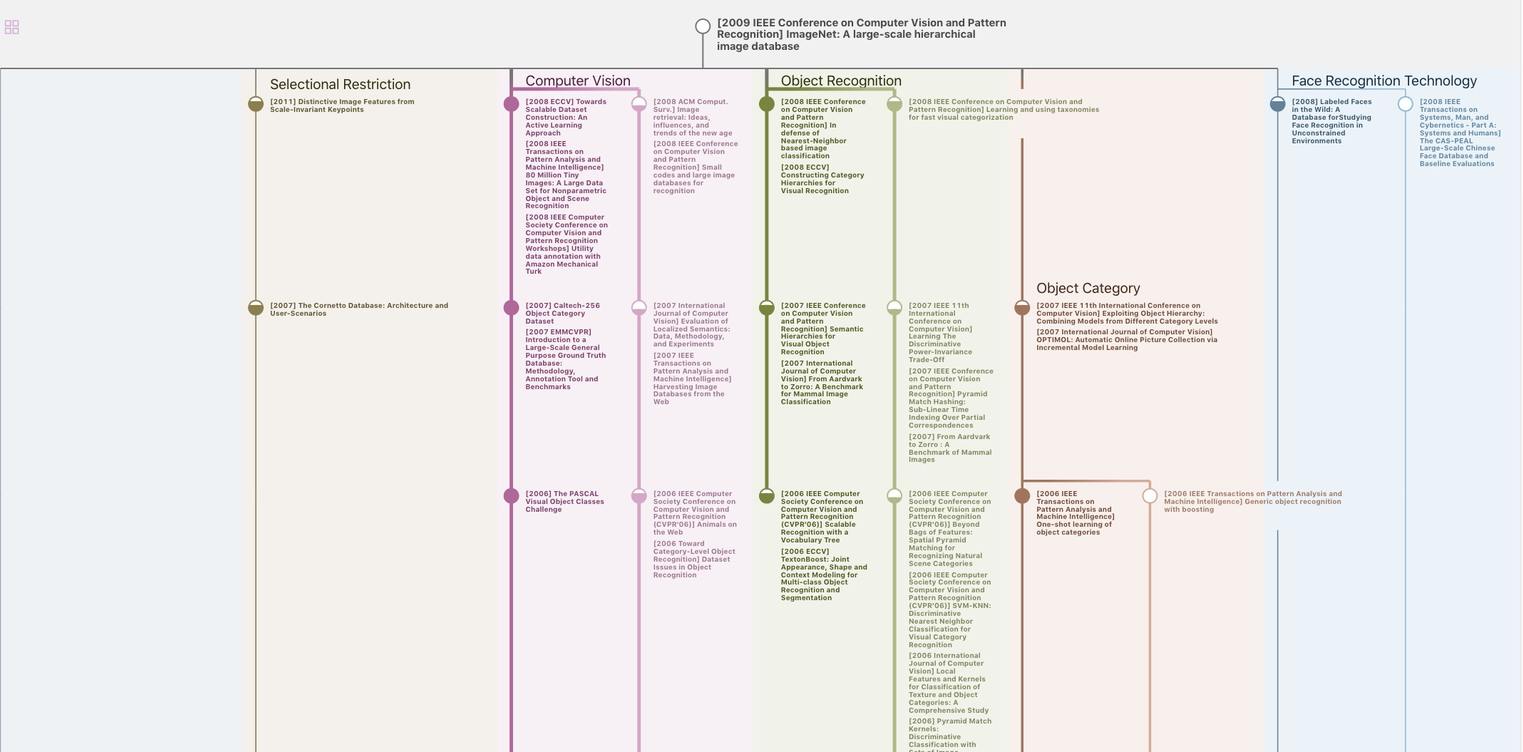
生成溯源树,研究论文发展脉络
Chat Paper
正在生成论文摘要