Feature Aggregation with Latent Generative Replay for Federated Continual Learning of Socially Appropriate Robot Behaviours
CoRR(2024)
摘要
For widespread real-world applications, it is beneficial for robots to
explore Federated Learning (FL) settings where several robots, deployed in
parallel, can learn independently while also sharing their learning with each
other. This work explores a simulated living room environment where robots need
to learn the social appropriateness of their actions. We propose Federated Root
(FedRoot), a novel weight aggregation strategy which disentangles feature
learning across clients from individual task-based learning. Adapting popular
FL strategies to use FedRoot instead, we present a novel FL benchmark for
learning the social appropriateness of different robot actions in diverse
social configurations. FedRoot-based methods offer competitive performance
compared to others while offering sizeable (up to 86
72
interactions require social robots to dynamically adapt to changing
environmental and task settings. To facilitate this, we propose Federated
Latent Generative Replay (FedLGR), a novel Federated Continual Learning (FCL)
strategy that uses FedRoot-based weight aggregation and embeds each client with
a generator model for pseudo-rehearsal of learnt feature embeddings to mitigate
forgetting in a resource-efficient manner. Our benchmark results demonstrate
that FedRoot-based FCL methods outperform other methods while also offering
sizeable (up to 84
resource consumption, with FedLGR providing the best results across
evaluations.
更多查看译文
AI 理解论文
溯源树
样例
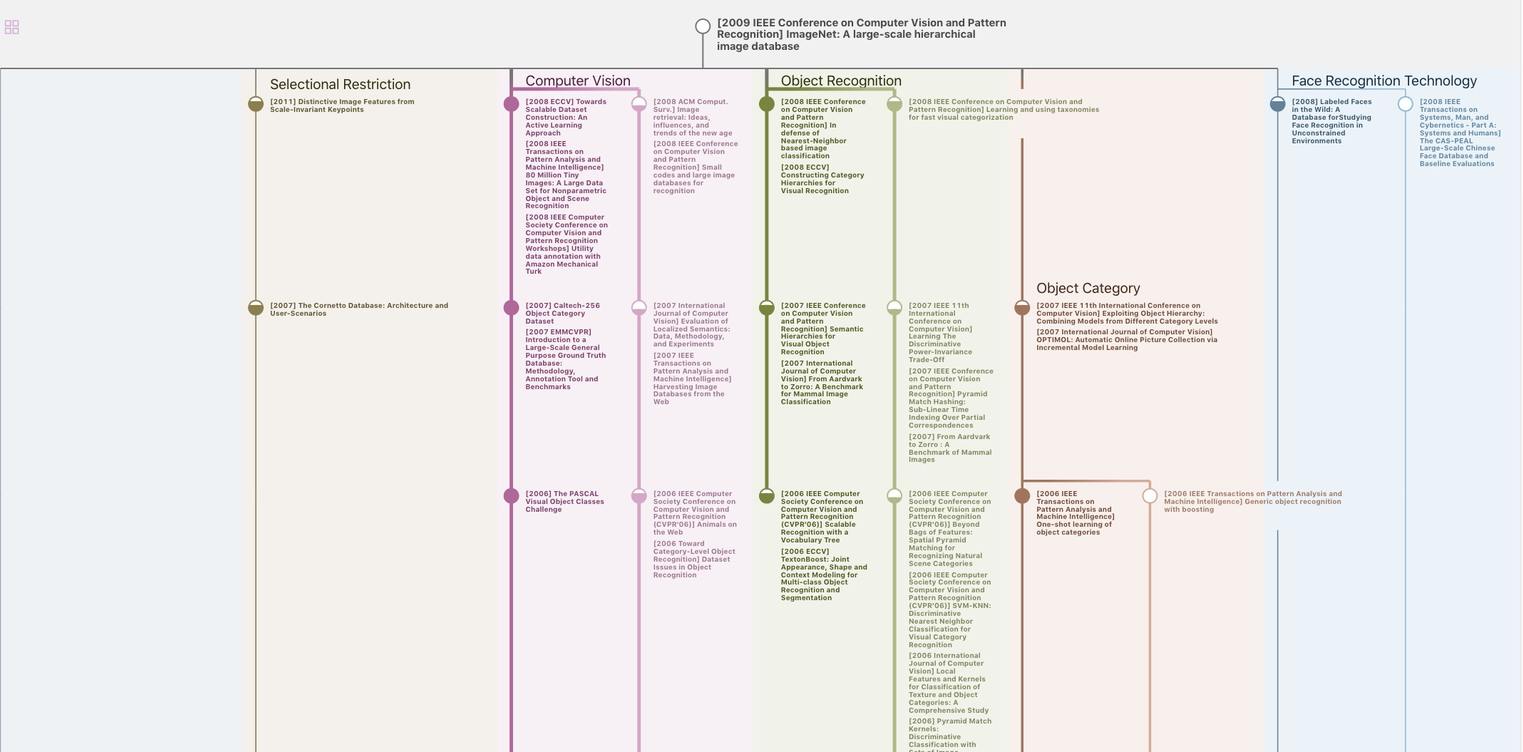
生成溯源树,研究论文发展脉络
Chat Paper
正在生成论文摘要