Enhancing Robustness to Novel Visual Defects Through StyleGAN Latent Space Navigation: a Manufacturing Use Case
Journal of intelligent manufacturing(2024)
Abstract
Visual Quality Inspection is an integral part of the manufacturing process that is becoming increasingly automated with the advent of Industry 4.0. While very beneficial, AI-driven Computer Vision Algorithms and Deep Neural Networks face several issues that may impede their adoption in practical real-life settings such as a manufacturing shop floor. One such issue arising during an AI classifier’s continuous operation is the frequent lack of robustness to novel defects appearing for the first time. Such unanticipated inputs can pose a significant risk to cyber-physical applications as a resulting out-of-context decision could compromise the integrity of the production process. While recent Machine Learning methods can theoretically tackle this problem from different angles (e.g., open-set recognition, semi-supervised learning, intelligent data augmentation), applying them to a real-life setting with a small, imbalanced dataset and high inter-class similarity can be challenging. This paper confronts such a use case aiming at the automation of the visual quality inspection of shaver shell brand prints from the electronics industry and characterized by data scarcity and the existence of small local defects. To that end, we introduce a novel data augmentation approach based on the latent space manipulation of StyleGAN, where defect data is intentionally synthesized to simulate novel inputs that can help form a boundary of the model’s knowledge. Our approach shows promising results compared to well-established open-set recognition and semi-supervised methods applied to the same problem, while its consistent performance across classifier embeddings indicates lower coupling to the final classifier.
MoreTranslated text
Key words
Open-set Recognition,Defect Recognition,Data Augmentation,Deep Learning,Generative Adversarial Networks
AI Read Science
Must-Reading Tree
Example
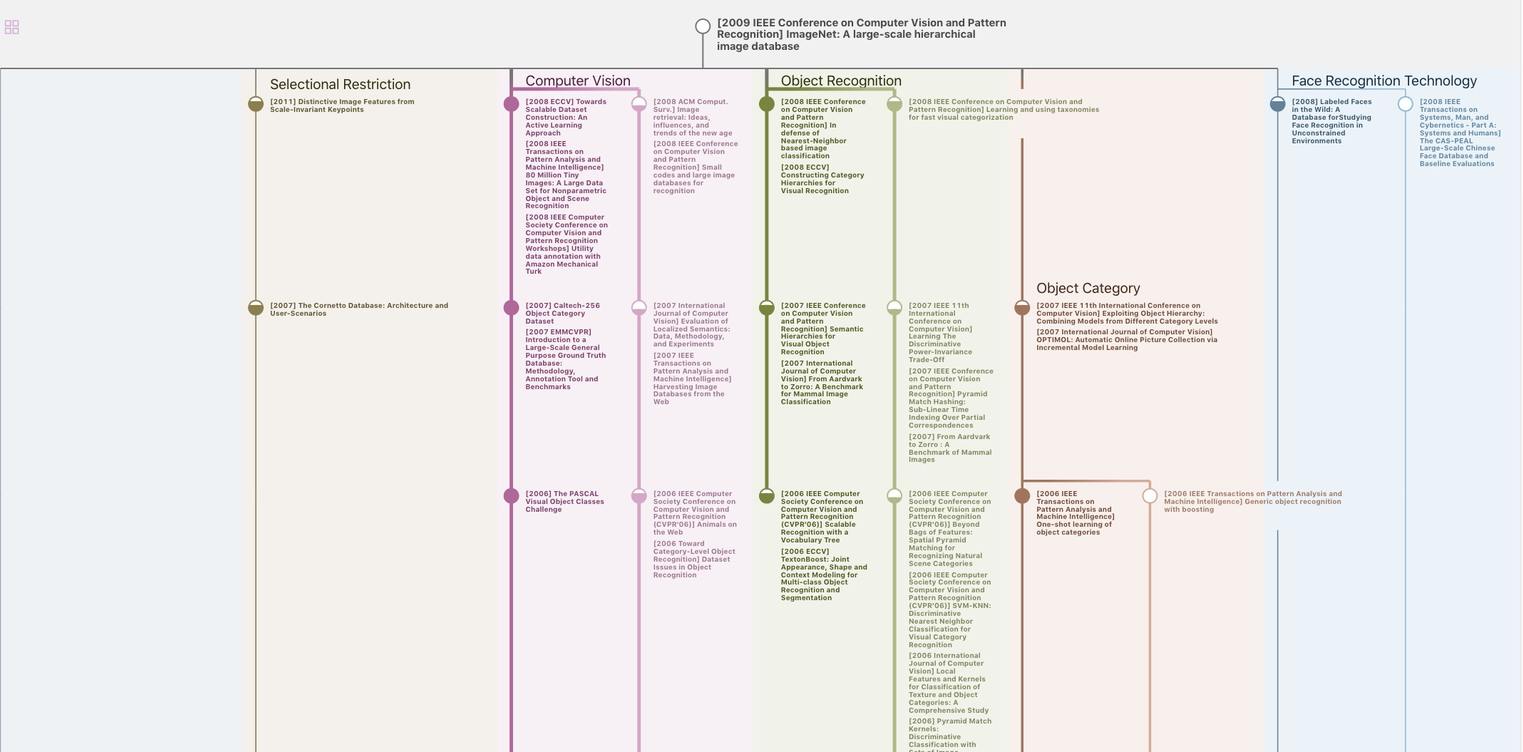
Generate MRT to find the research sequence of this paper
Chat Paper
Summary is being generated by the instructions you defined