Open-Vocabulary SAM3D: Towards Training-free Open-Vocabulary 3D Scene Understanding
arxiv(2024)
摘要
Open-vocabulary 3D scene understanding presents a significant challenge in the field. Recent works have sought to transfer knowledge embedded in vision-language models from 2D to 3D domains. However, these approaches often require prior knowledge from specific 3D scene datasets, limiting their applicability in open-world scenarios. The Segment Anything Model (SAM) has demonstrated remarkable zero-shot segmentation capabilities, prompting us to investigate its potential for comprehending 3D scenes without training. In this paper, we introduce OV-SAM3D, a training-free method that contains a universal framework for understanding open-vocabulary 3D scenes. This framework is designed to perform understanding tasks for any 3D scene without requiring prior knowledge of the scene. Specifically, our method is composed of two key sub-modules: First, we initiate the process by generating superpoints as the initial 3D prompts and refine these prompts using segment masks derived from SAM. Moreover, we then integrate a specially designed overlapping score table with open tags from the Recognize Anything Model (RAM) to produce final 3D instances with open-world labels. Empirical evaluations on the ScanNet200 and nuScenes datasets demonstrate that our approach surpasses existing open-vocabulary methods in unknown open-world environments.
更多查看译文
AI 理解论文
溯源树
样例
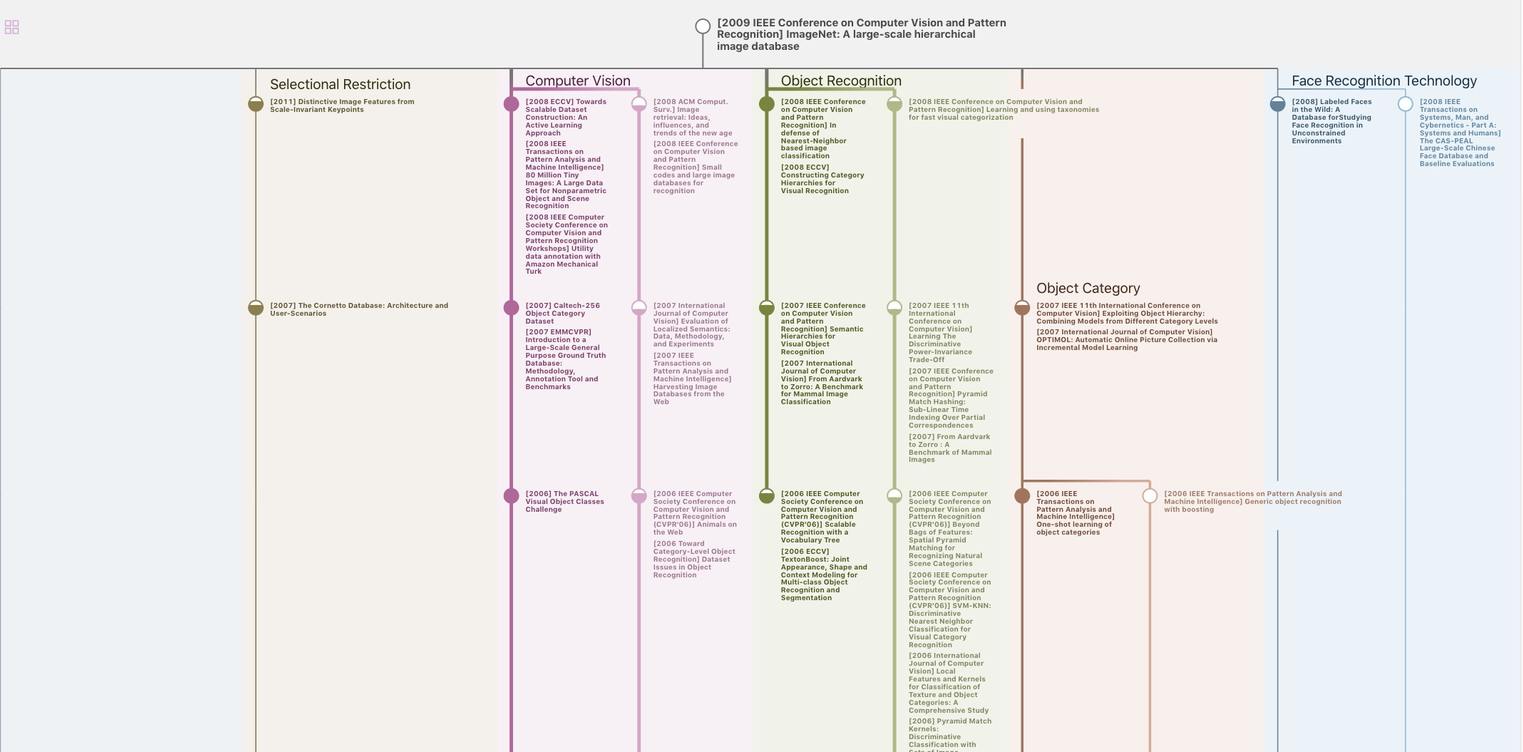
生成溯源树,研究论文发展脉络
Chat Paper
正在生成论文摘要