Bayesian Game-Driven Incentive Mechanism for Blockchain-Enabled Secure Federated Learning in 6 G Wireless Networks
IEEE Transactions on Network Science and Engineering(2024)
摘要
The sixth-generation (6 G) wireless networks are envisioned to build a data-driven digital world with widespread Artificial Intelligence (AI). Federated learning (FL) is a distributed AI paradigm that coordinates different data owners to train shared AI models cooperatively. However, traditional FL faces challenges in practically deploying in 6 G networks: (i) the central server becomes the bottleneck and fails to identify clients' malicious behaviors, and (ii) the lack of incentive mechanisms makes heterogeneous nodes hard to collaborate when considering unilateral returns. To address the above challenges, we first propose a blockchain-enabled FL (BFL) framework where clients' malicious behaviors could be identified without a central server. Then we propose a Bayesian game-driven incentive mechanism to encourage honest nodes to provide valid models while hindering the training interference from malicious clients. Moreover, we propose a dynamic data contribution scheme to schedule data resources equitably while ensuring model performance. Finally, a Proof-of-Incentive consensus mechanism is designed as benign impetuses to guide the system toward the direction of more secure model aggregation and higher incentives. Experimental results show that our proposed schemes can obtain high-precision models even with malicious clients and effectively motivate honest nodes to join FL in 6 G networks.
更多查看译文
AI 理解论文
溯源树
样例
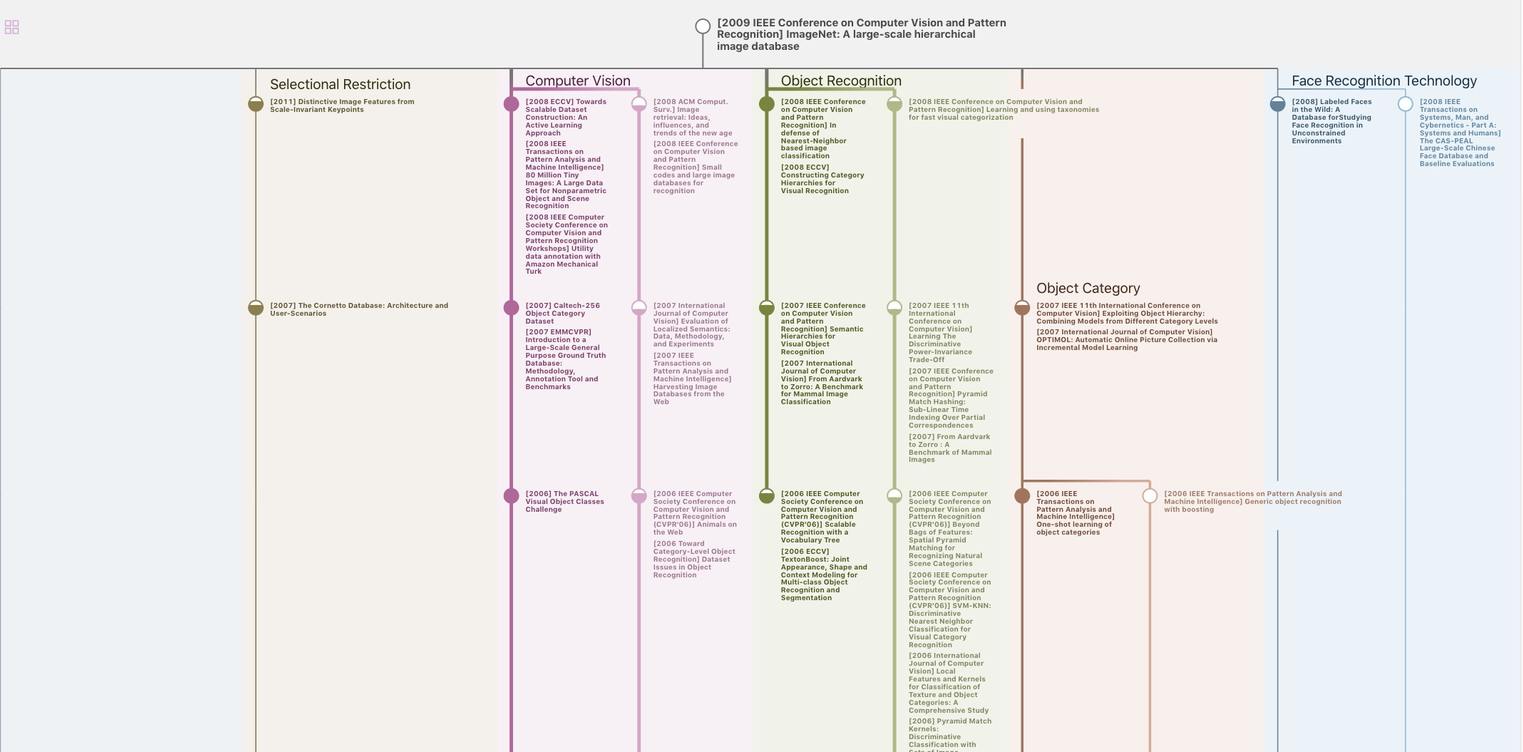
生成溯源树,研究论文发展脉络
Chat Paper
正在生成论文摘要