Quantitative and Qualitative Assessment of Left Atrial Cardiomyopathy As Well As Prediction of Atrial Fibrillation Recurrences Upon PVI Using Machine Learning
Europace(2024)
摘要
Abstract Introduction Atrial fibrillation (AF) is one of the most common heart pathologies in daily clinical practice and confers significant mortality and morbidity. However, AF ablation often proves ineffective - even after successful pulmonary vein isolation (PVI). The prediction of PVI failure for the long-term treatment of AF as well as the improved assessment of the underlying substrate appears pivotal. We hypothesize, that ML can be applied to better characterize the substrate and recurrence in AF patients upon PVI. Methods & Results We retrospectively evaluated data from 205 patients that underwent AF ablation between 2019 and 2023, including high-density 3D electroanatomic maps (sinus rhythm), LA rotational angiography, invasive LA hemodynamic measurements (after transseptal passage), echocardiography, 12-lead ECG and laboratory testing. A neuronal net was trained on selected features extracted from those datasets using supervised learning and employed to predict measures of atrial cardiomyopathy and AF recurrence. Patients were 66±1 years old and received first or second pulmonary vein isolation (PVI) for paroxysmal atrial fibrillation with 18% documented recurrences upon a mean follow-up of 23±1months. First, we extensively refined or developed tools for automated P-wave analysis from 12-lead ECGs as well as local LA voltage and volume quantification. Next, LA hemodynamics and volumes were assessed: Mean LA volume as derived from rotational angiography significantly correlated with LA volume index as obtained using echocardiography. Interestingly, LA size showed no correlation with NT-proBNP, LA low voltage or LA hemodynamics. In addition, global (25±2% of total LA area) and local (i.e. anterior, posterior, roof) LA voltage was determined and shown to be an independent predictor of AF recurrence. In our first unselected subset of 136 patients with 2nd PVI for atrial fibrillation, our ML models predicted LA fibrosis (i.e. low voltage regions; Utah stage) from 12-lead ECGs with an accuracy of 90%. Accuracy was 87% for the prediction of AF recurrences from LA pressure and low-voltage regions. In addition, the algorithm predicted AF recurrences from 12-lead SR ECGs obtained before PVI with an accuracy of 91%. Conclusion Artificial neural networks allow to quantitatively and qualitatively predict measures of atrial cardiomyopathy and atrial fibrillation recurrence probability upon complete PVI with high accuracy.Figures
更多查看译文
AI 理解论文
溯源树
样例
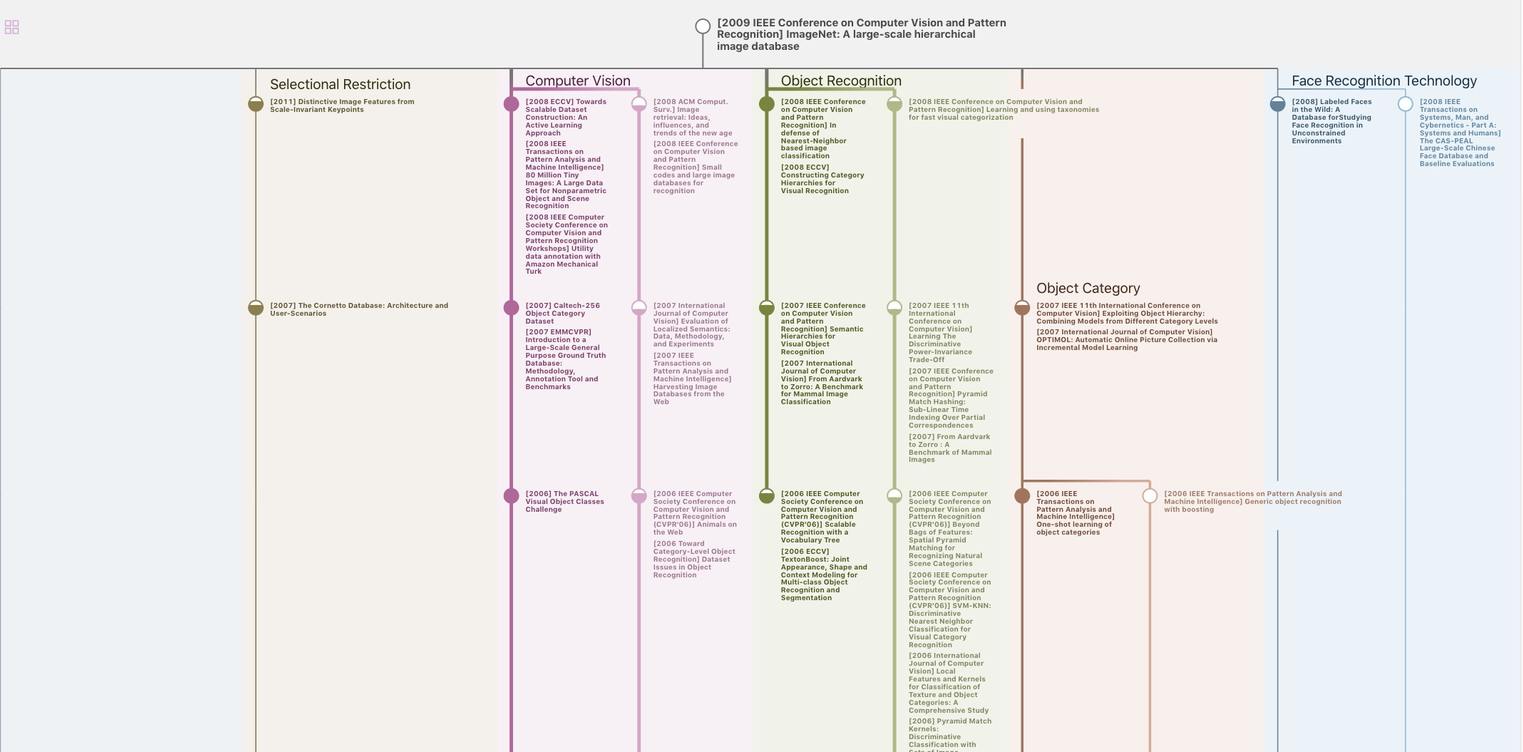
生成溯源树,研究论文发展脉络
Chat Paper
正在生成论文摘要