Development and validation of a symbolic regression-based machine learning method to predict COVID-19 in-hospital mortality among vaccinated patients
Health and Technology(2024)
摘要
The continuous evolution of SARS-CoV-2 and possible future pandemics have risen concerns relevant to the effectiveness of the vaccines which are currently available. To this direction, new computational tools based on artificial intelligence (AI) and machine learning (ML) methods are incorporated, focusing on revealing hidden patterns and behaviors from, oftentimes, a great number of parameters that may affect (or not) the evolution of the pandemic. In this study, we developed and validated prediction models of COVID-19 in-hospital mortality among vaccinated patients by applying Symbolic Regression (SR)-based, ML algorithms. Considering the key role of cytokines and chemokines in the modulation of the immune response, we employed a dataset combining several of the aforementioned biomolecules with commonly used laboratory markers as well as demographic and clinical data. Starting from a forty-four features dataset, we managed to restrict the total number of employed variables between 6–8 and ended up in four possible equations accurately predicting data behavior. The feature ‘Days with symptoms from onset until admission’ appeared in every equation, while interleukins (ILs)-17A and -6 in 3 out of 4 models. The parameters ‘IL-6’ and ‘IL-17A’, wherever combined led in a different survival effect on patients, compared to those cases where they solely appeared in an equation. Our method is presented for the first time and aims to be part of a broader computational and statistical framework that could aid in medical decision-making applications.
更多查看译文
关键词
COVID-19,Cytokines,In-hospital mortality,Machine learning,Symbolic regression,Vaccination
AI 理解论文
溯源树
样例
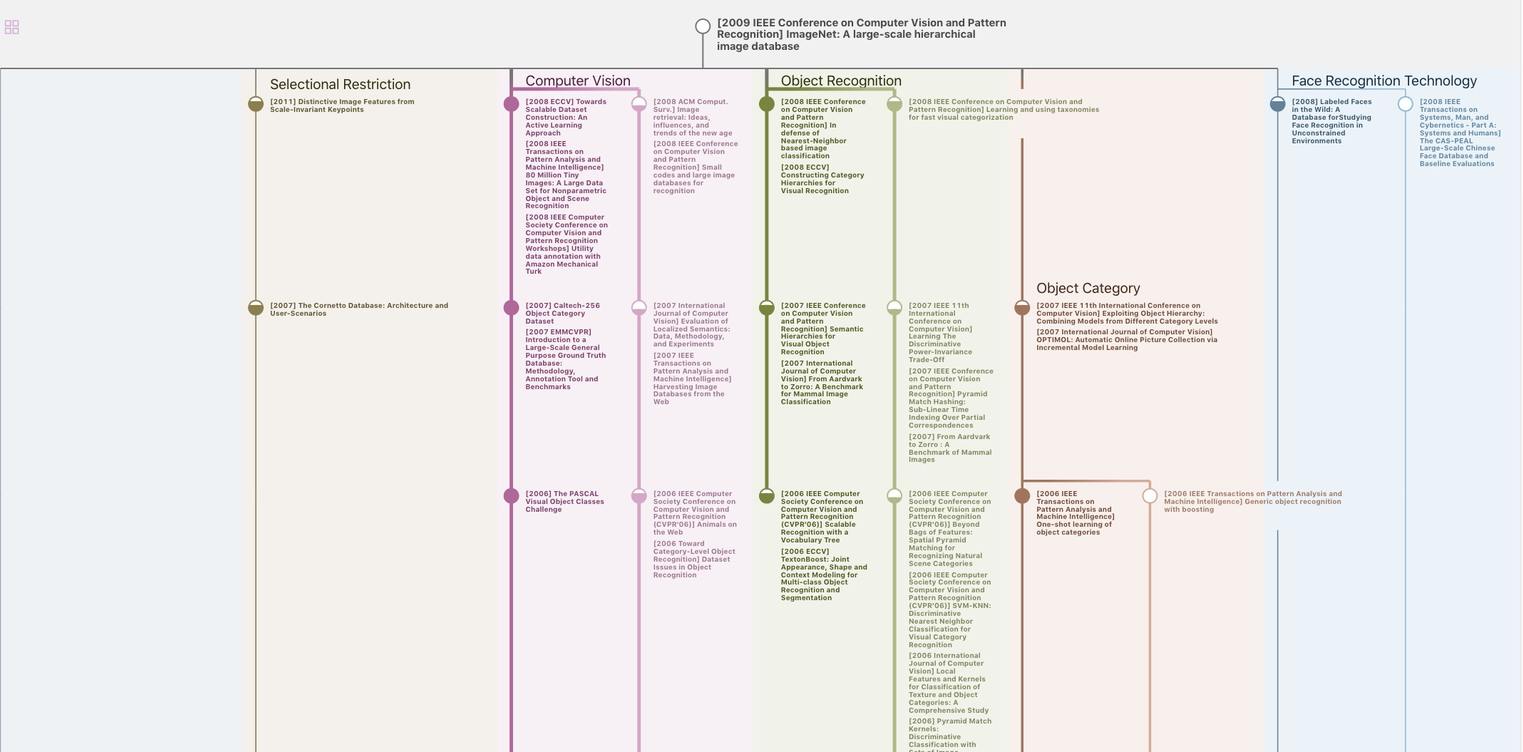
生成溯源树,研究论文发展脉络
Chat Paper
正在生成论文摘要