Beyond Accuracy and Robustness Metrics for Large Language Models for Code
ICSE-Companion '24 Proceedings of the 2024 IEEE/ACM 46th International Conference on Software Engineering Companion Proceedings(2024)
摘要
In recent years, Large Language Models for code (LLMc) have transformed the landscape of software engineering (SE), demonstrating significant efficacy in tasks such as code completion, summarization, review, tracing, translation, test case generation, clone detection, and bug fixing. Notably, GitHub Copilot [31] and Google's CodeBot [21] exemplify how LLMc contributes to substantial time and effort savings in software development. However, despite their widespread use, there is a growing need to thoroughly assess LLMc, as current evaluation processes heavily rely on accuracy and robustness metrics, lacking consensus on additional influential factors in code generation. This gap hinders a holistic understanding of LLMc performance, impacting interpretability, efficiency, bias, fair-ness, and robustness. The challenges in benchmarking and data maintenance compound this issue, underscoring the necessity for a comprehensive evaluation approach. To address these issues, this dissertation proposes the development of a benchmarking infras-tructure, named HolBench, aimed at overcoming gaps in evaluating LLMc quality. The goal is to standardize testing scenarios, facilitate meaningful comparisons across LLMc, and provide multi-metric measurements beyond a sole focus on accuracy. This approach aims to decrease the costs associated with advancing LLMc research, en-hancing their reliability for adoption in academia and industry.
更多查看译文
关键词
deep learning,code generation,interpretability,transformers
AI 理解论文
溯源树
样例
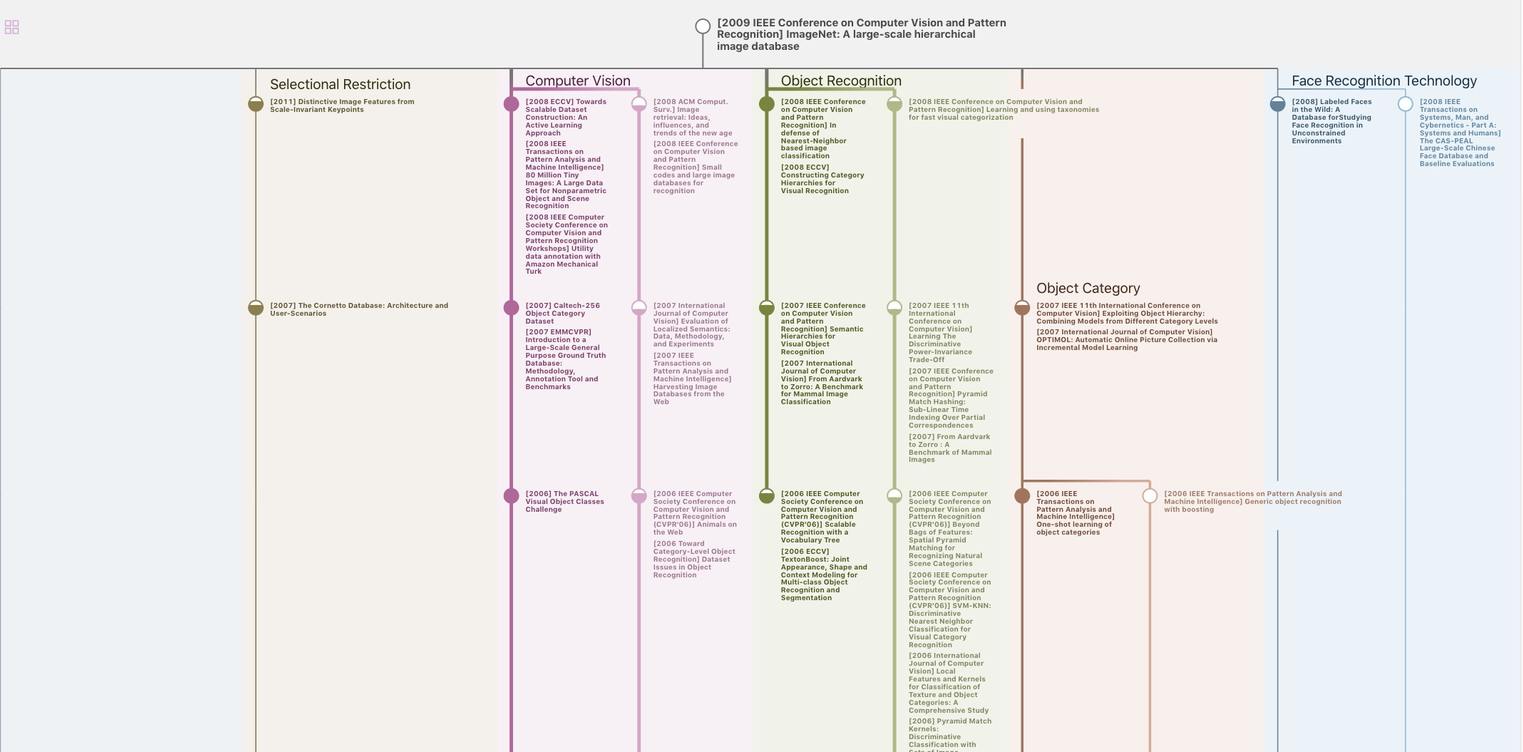
生成溯源树,研究论文发展脉络
Chat Paper
正在生成论文摘要