Single and multi-tasked neural networks: selection and deployment.
International Conference on Autonomous Robot Systems and Competitions(2024)
摘要
Efficient perception is a fundamental requirement for ADAS and ADS, with implications for safety, accuracy, and speed. The choice between multi-tasked and single-tasked deep learning networks can significantly impact the performance of these systems and their ability to understand and respond to the complex driving environment. This paper explores the comparison between multi-tasked neural networks and multiple single-tasked networks. To make the comparisons possible and also to implement different kinds of models in an inference unit, a versatile application designed to seamlessly run multiple deeplearning models with distinct tasks was developed. With this application, the single-tasked networks YOLOv8, SegFormer, and Ultrafast lane detection v2 were evaluated while running individually and simultaneously. The evaluated multi-tasked networks were the YOLOPv2 and TiwnLiteNet. This study concluded that both the multi-tasked YOLOPv2 and the combination of the single-tasked YOLOv8 with multi-tasked TiwnLiteNet are the best solutions.
更多查看译文
关键词
deep-learning,inference,driving assistance,multiple models,multi-task
AI 理解论文
溯源树
样例
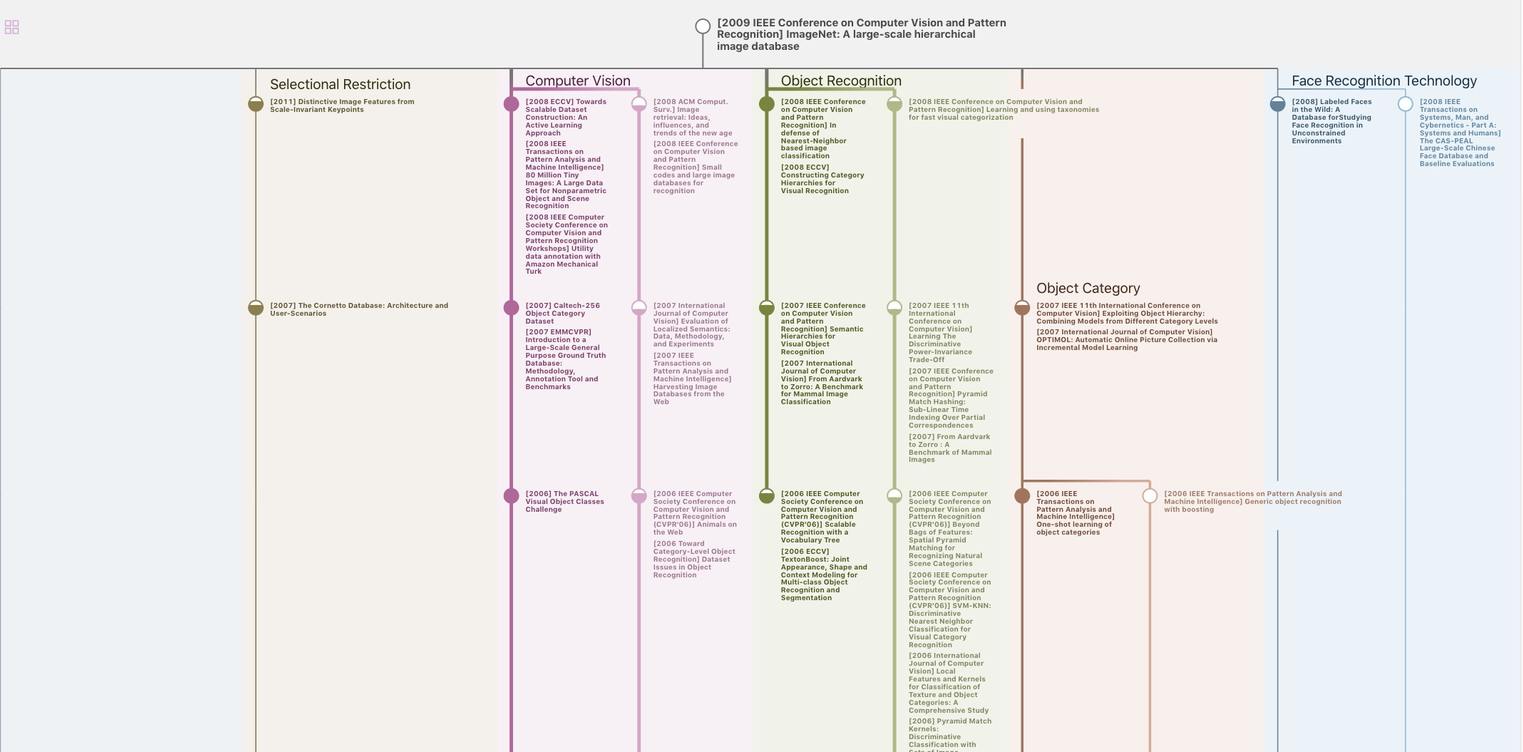
生成溯源树,研究论文发展脉络
Chat Paper
正在生成论文摘要