ChronosLex: Time-aware Incremental Training for Temporal Generalization of Legal Classification Tasks
Proceedings of the 62nd Annual Meeting of the Association for Computational Linguistics (Volume 1 Long Papers)(2024)
摘要
This study investigates the challenges posed by the dynamic nature of legalmulti-label text classification tasks, where legal concepts evolve over time.Existing models often overlook the temporal dimension in their trainingprocess, leading to suboptimal performance of those models over time, as theytreat training data as a single homogeneous block. To address this, weintroduce ChronosLex, an incremental training paradigm that trains models onchronological splits, preserving the temporal order of the data. However, thisincremental approach raises concerns about overfitting to recent data,prompting an assessment of mitigation strategies using continual learning andtemporal invariant methods. Our experimental results over six legal multi-labeltext classification datasets reveal that continual learning methods proveeffective in preventing overfitting thereby enhancing temporalgeneralizability, while temporal invariant methods struggle to capture thesedynamics of temporal shifts.
更多查看译文
AI 理解论文
溯源树
样例
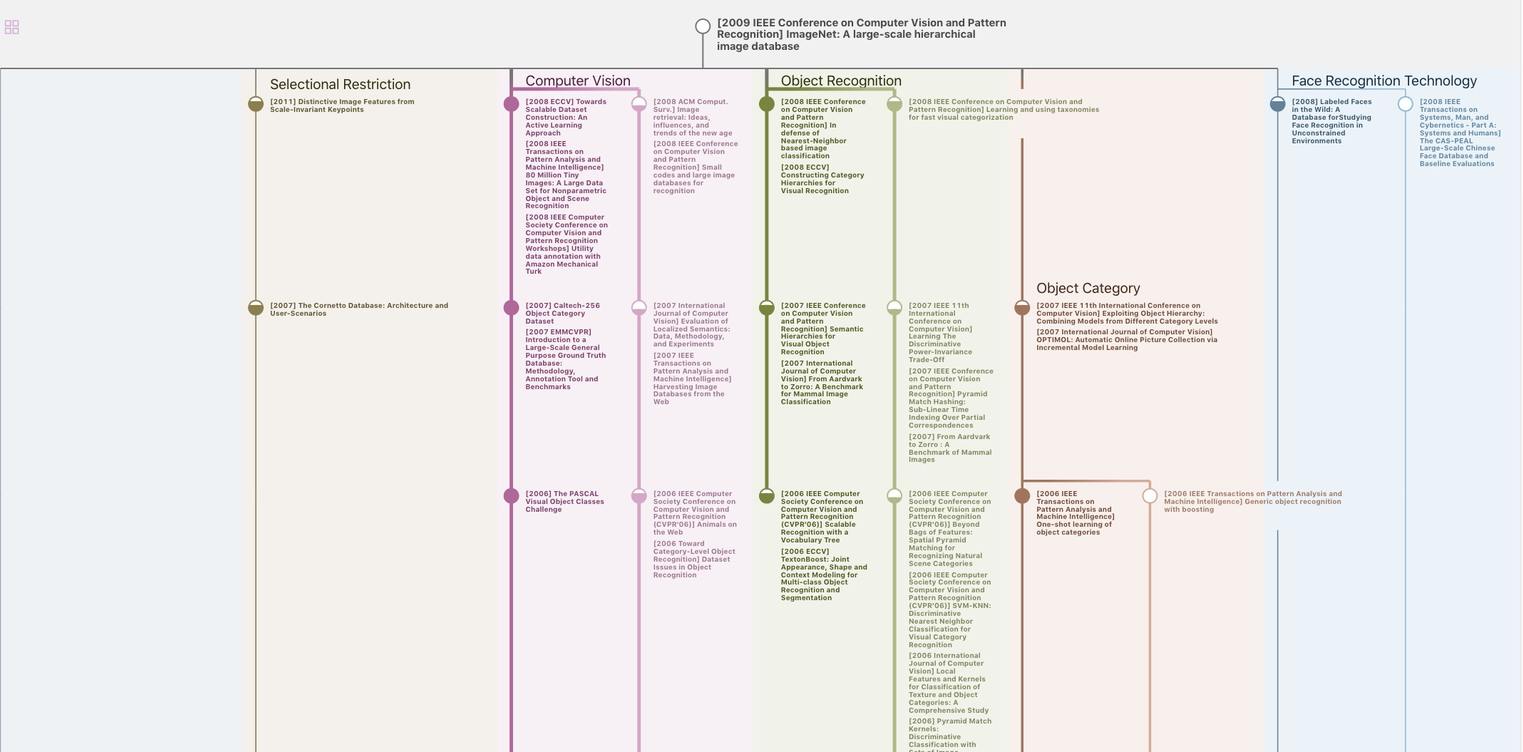
生成溯源树,研究论文发展脉络
Chat Paper
正在生成论文摘要