THE RELATIONSHIP BETWEEN CIRCADIAN BLOOD PRESSURE PROFILE AND LEFT ATRIAL MECHANICS, ARTERIAL STIFFNESS AND LEFT VENTRICULAR PERFORMANCE IN HYPERTENSIVE PATIENTS
JOURNAL OF HYPERTENSION(2024)
Jagiellonian Univ | Univ Hosp
Abstract
Objective: Background: The left atrium plays a crucial role in managing left ventricular filling and contractility through its functions as a reservoir, conduit, and boost pump. Consequently, the interaction between the left atrium(LA), left ventricle(LV), and the arterial system appears as a critical determinant of comprehensive cardiovascular performance. Non-dipping profile of blood pressure(BP) in 24-hr monitoring(ABPM) is associated with increased cardiovascular mortality and morbidity, including atrial fibrillation and stroke. We aimed to investigate the association between LA mechanics, LV performance, arterial stiffness and circadian rhythm of BP in essential hypertension. Design and method: Hypertensive patients without overt cardiovascular disease were recruited. In all subjects 24-hr ABPM and extended echocardiographic image acquisition protocol were performed(Vivid E95, GE). Carotid-femoral pulse wave velocity(PWV) was measured with the SphygmoCor device(Atcor Medical). All analyses, including LV global longitudinal strain(GLS) and LA reservoir strain(LAR), LA conduit strain(LACD), LA booster pump strain(LACT) were performed off-line. The related factors were evaluated by multivariate linear regression analysis to find the independent factors. Statistical analysis was conducted using SPSS statistics software Results: Study group included 219 patients(age 55.3±13.5 years, 55% females). According to BP patterns patients were divided into two groups: non-dippers(n=53), presenting with <10% reduction in both systolic and diastolic BP at nighttime, and dippers(n=166). Non-dippers were older(61.3±11.3 vs 54.2±13.7 years, p<0.01) and exhibited higher BMI(30.2±4.9 vs 28.6±4.5 kg/m2 p<0.01). There were no differences between groups in office BP(non-dippers 128.3±13.3/72.9±9.5 vs 128.1±14.6/75.8±10.8 mmHg in dippers), neither 24hr BP(124.2±13.3/73.5±9.6 vs 121.4±11.5/74.2±7.1 mmHg, respectively). After adjustments for age and BMI, in the non-dipper group higher PWV(8.3±1.2 vs 7.9±1.2, p=0.05) and LACD(-14.7±5.7 vs -17.0±6.7, p<0.001) were observed, with no-differences in LV GLS(-20.7±3.3 vs -19.8±3.9; NS). Multivariate linear regression analysis revealed that age(p<0.001), office(p<0.001) and nighttime SBP(p=0.008)(but not dipping status, p=0.50), diabetes(p=0.02) and creatinine level(p=0.02) were independently corelated with PWV. LACD was independently corelated with age(p<0.001), LV mass(p=0.04) and LV GLS(p=0.03) Conclusions: Arterial stiffness and left atrial performance are associated with circadian rhythm of BP. Nocturnal systolic BP but not dipping profile is the independent risk factor of worse arterial and left atrial performance.
MoreTranslated text
求助PDF
上传PDF
View via Publisher
AI Read Science
AI Summary
AI Summary is the key point extracted automatically understanding the full text of the paper, including the background, methods, results, conclusions, icons and other key content, so that you can get the outline of the paper at a glance.
Example
Background
Key content
Introduction
Methods
Results
Related work
Fund
Key content
- Pretraining has recently greatly promoted the development of natural language processing (NLP)
- We show that M6 outperforms the baselines in multimodal downstream tasks, and the large M6 with 10 parameters can reach a better performance
- We propose a method called M6 that is able to process information of multiple modalities and perform both single-modal and cross-modal understanding and generation
- The model is scaled to large model with 10 billion parameters with sophisticated deployment, and the 10 -parameter M6-large is the largest pretrained model in Chinese
- Experimental results show that our proposed M6 outperforms the baseline in a number of downstream tasks concerning both single modality and multiple modalities We will continue the pretraining of extremely large models by increasing data to explore the limit of its performance
Upload PDF to Generate Summary
Must-Reading Tree
Example
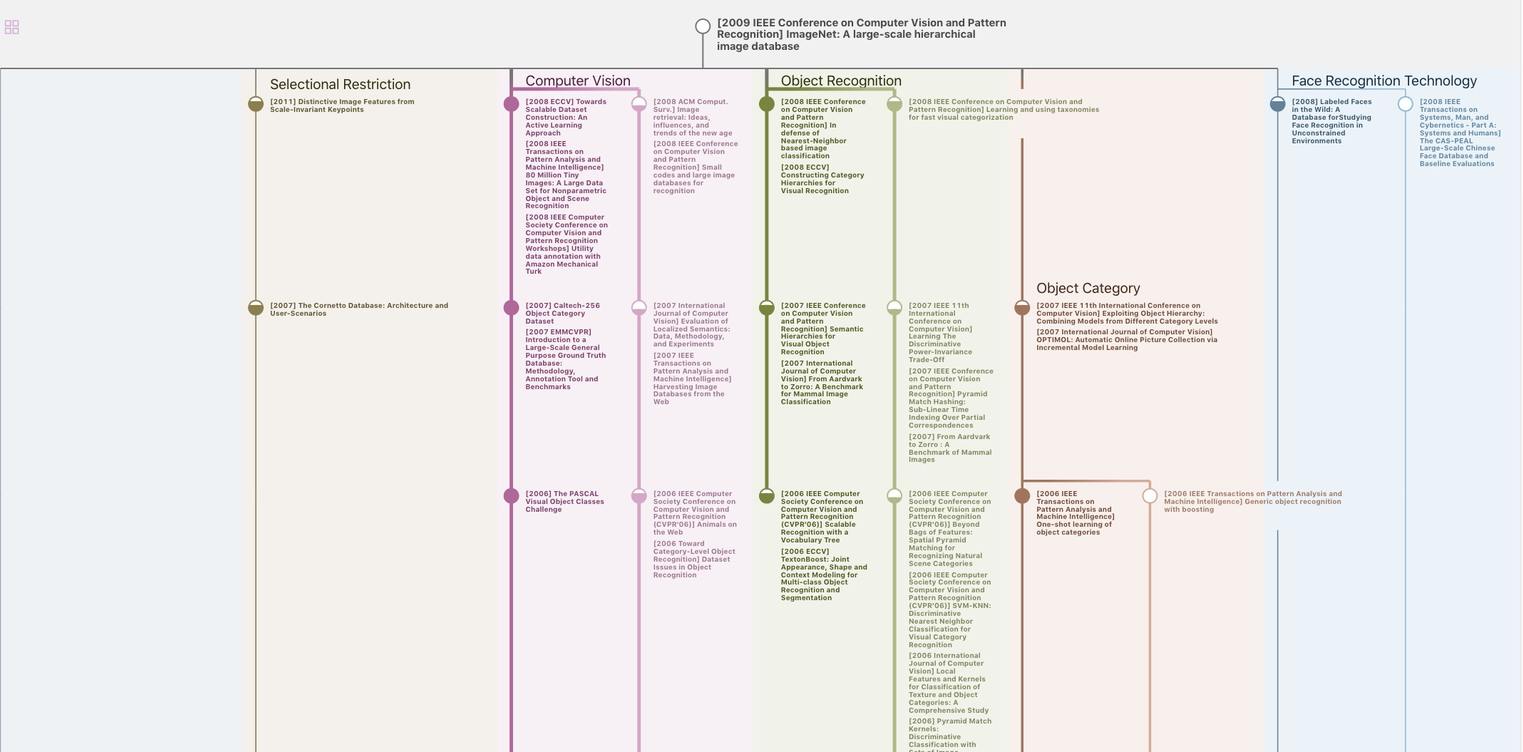
Generate MRT to find the research sequence of this paper
Data Disclaimer
The page data are from open Internet sources, cooperative publishers and automatic analysis results through AI technology. We do not make any commitments and guarantees for the validity, accuracy, correctness, reliability, completeness and timeliness of the page data. If you have any questions, please contact us by email: report@aminer.cn
Chat Paper
GPU is busy, summary generation fails
Rerequest