Multi-attention Associate Prediction Network for Visual Tracking
arXiv (Cornell University)(2024)
摘要
Classification-regression prediction networks have realized impressive success in several modern deep trackers. However, there is an inherent difference between classification and regression tasks, so they have diverse even opposite demands for feature matching. Existed models always ignore the key issue and only employ a unified matching block in two task branches, decaying the decision quality. Besides, these models also struggle with decision misalignment situation. In this paper, we propose a multi-attention associate prediction network (MAPNet) to tackle the above problems. Concretely, two novel matchers, i.e., category-aware matcher and spatial-aware matcher, are first designed for feature comparison by integrating self, cross, channel or spatial attentions organically. They are capable of fully capturing the category-related semantics for classification and the local spatial contexts for regression, respectively. Then, we present a dual alignment module to enhance the correspondences between two branches, which is useful to find the optimal tracking solution. Finally, we describe a Siamese tracker built upon the proposed prediction network, which achieves the leading performance on five tracking benchmarks, consisting of LaSOT, TrackingNet, GOT-10k, TNL2k and UAV123, and surpasses other state-of-the-art approaches.
更多查看译文
关键词
Multiple Object Tracking,Object Tracking,Visual Tracking,Real-time Tracking,Foreground Segmentation
AI 理解论文
溯源树
样例
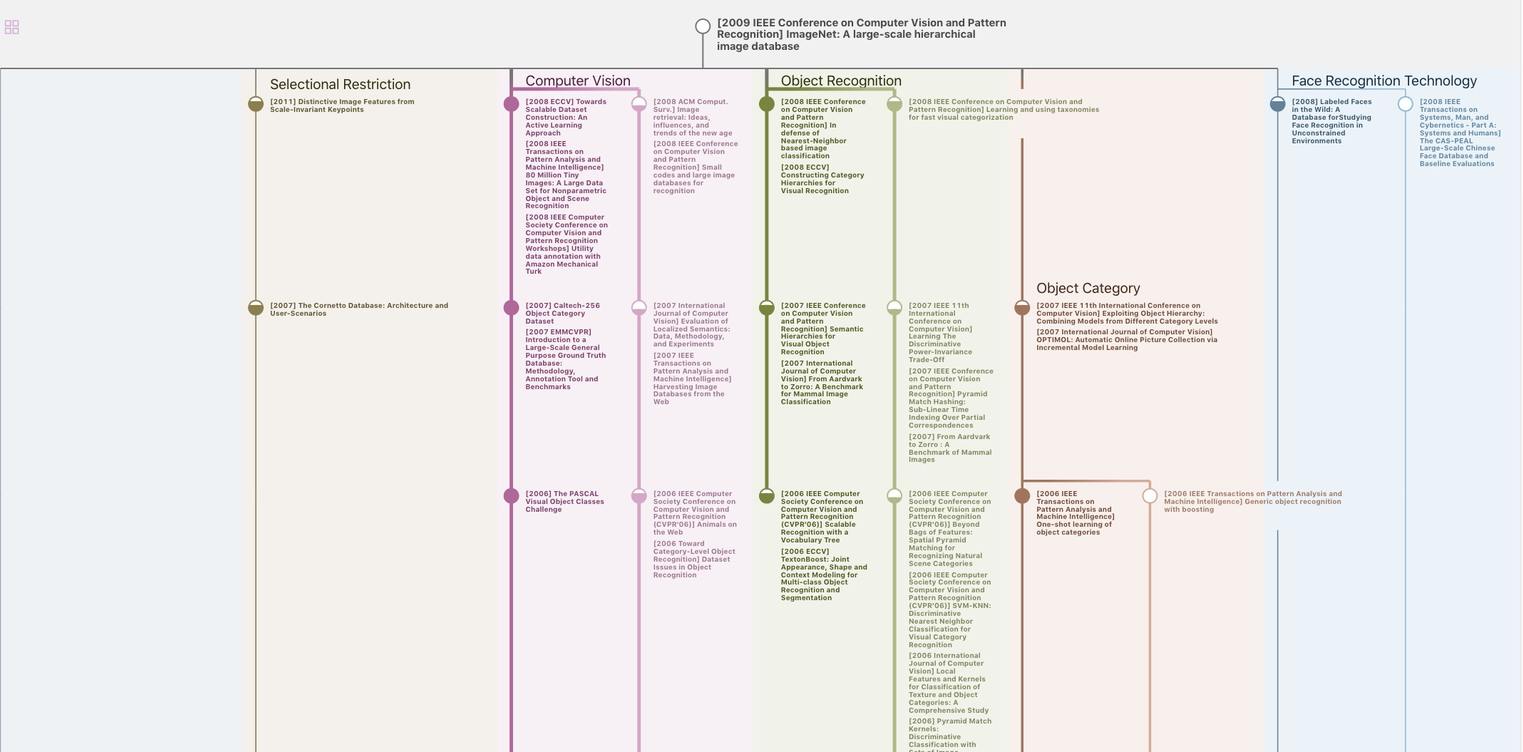
生成溯源树,研究论文发展脉络
Chat Paper
正在生成论文摘要