Bayesian Prediction of Afghanistan Annual Population Growth Using ARIMA and BSTS Models
crossref(2024)
摘要
Abstract This study analyzes Afghanistan’s annual population growth using historical time series data from 1950 to 2022. The objectives are modeling, comparison, and prediction. Two models are employed: the auto-regressive integrated moving average (ARIMA) and the Bayesian Structural Time Series (BSTS). These models are fitted to the data, and their accuracy is evaluated using the Leave-One-Out Information Criteria (LOOIC), a Bayesian model selection criterion. The ARIMA(2,1,2) model is identified as suitable based on its minimum LOOIC value. The analysis is conducted using R statistical software and Stan proba-bilistic programming language for Bayesian modeling. The model comparison indicates the ARIMA model outperforms the BSTS model for this population growth data, exhibiting lower LOOIC values, suggesting better model fit and pre-dictive ability. The key result is a next-decade prediction of annual population growth from 2023 to 2032, generated using the preferred ARIMA(2,1,2) model. This model is deemed most appropriate for forecasting Afghanistan’s population growth, as evidenced by its superior performance on the LOOIC evaluation metric. The study demonstrates the effectiveness of the ARIMA model in capturing population growth dynamics and providing accurate forecasts for the upcoming decade.
更多查看译文
AI 理解论文
溯源树
样例
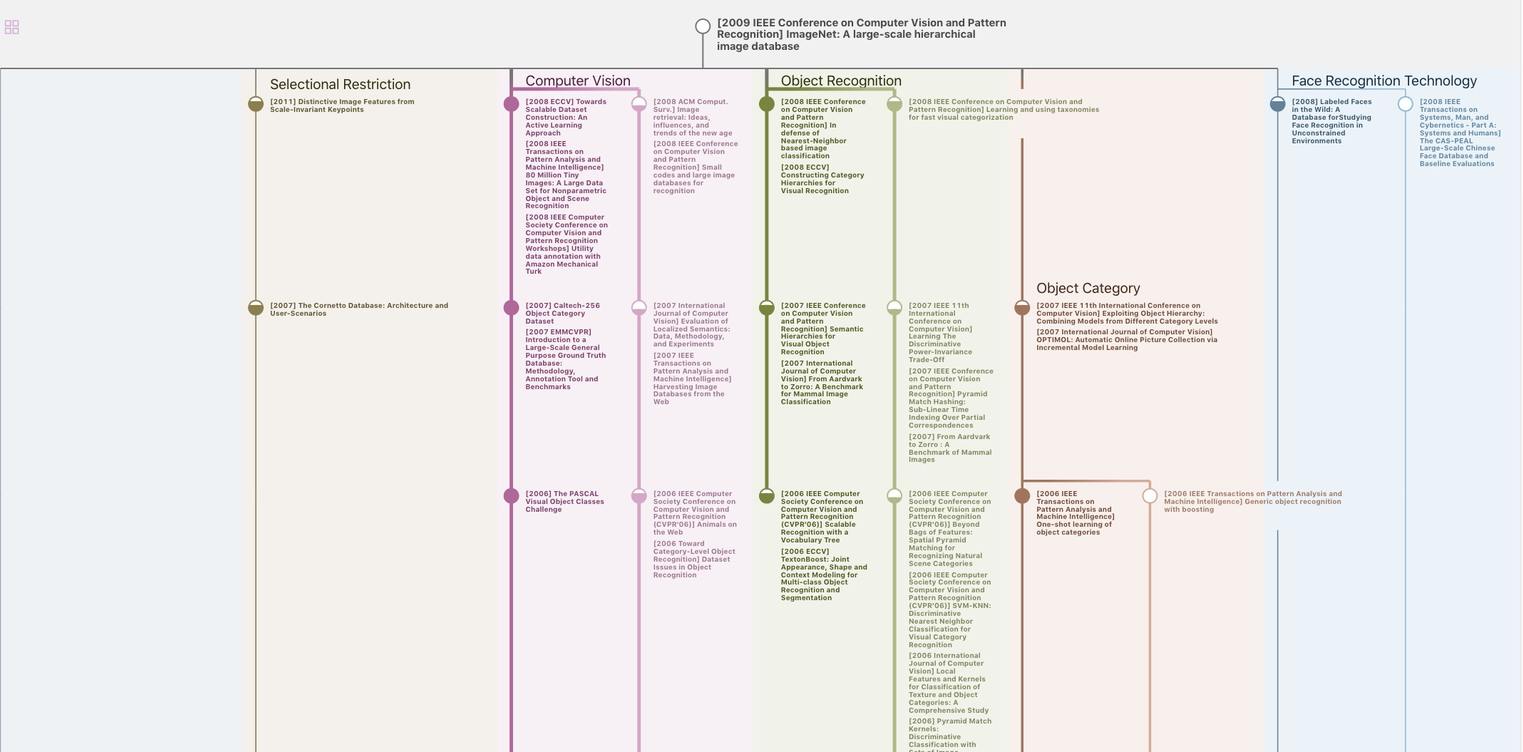
生成溯源树,研究论文发展脉络
Chat Paper
正在生成论文摘要