How Machine Learning Approaches Are Useful in Computing the Optical Properties of Non-Spherical Particles Across a Broad Range of Size Parameters?
Journal of Quantitative Spectroscopy & Radiative Transfer/Journal of quantitative spectroscopy & radiative transfer(2024)
Abstract
This study investigates the use of machine learning techniques, specifically deep neural networks (DNN), to compute the optical properties of non-spherical particles across a wide range of size parameters. The approach involves training a DNN using a T-matrix database of super-spheroids with size parameters below 50. The DNN is then able to predict optical properties for size parameters beyond this limit, with exceptional accuracy observed for size parameters between 50-100. These predictions outperform the improved geometric optics method (IGOM). To further enhance accuracy and broaden the range of size parameters, databases are merged, combining the T-matrix results for small (0.1-50) and some moderate size parameters (50-100), the DNN predicted values for moderate size parameters (50-100), and the IGOM results for large size parameters (>100). This merged database is used to train a new DNN. This comprehensive training enables the neural networks to calculate the optical properties for super-spheroids across the entire size parameter spectrum, considering various shape parameters and refractive indices. This technique effectively bridges the gap between full-electromagnetic wave results and geometric-optics approximations, providing an efficient method for computing the optical properties of non-spherical particles from Rayleigh to geometric optics domains. Notably, the DNNs automatically consider edge effects, eliminating the need for deriving complex analytical formulas for non-spherical particles.
MoreTranslated text
Key words
Light scattering,Non-spherical particles,Machine learning,Neural networks
AI Read Science
Must-Reading Tree
Example
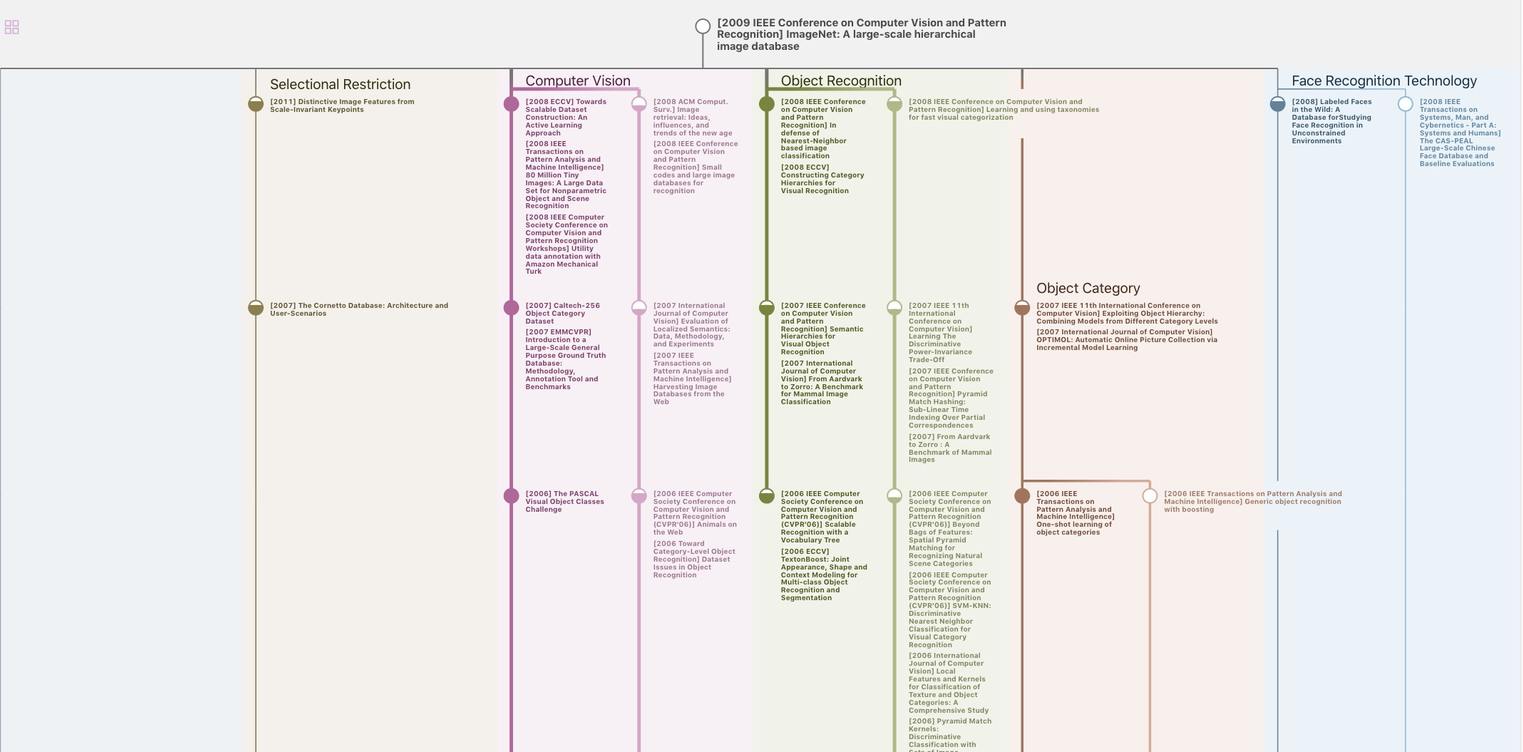
Generate MRT to find the research sequence of this paper
Chat Paper
Summary is being generated by the instructions you defined