RUL Prediction of Lithium-ion Batteries Using a Federated and Homomorphically Encrypted Learning Method
39TH ANNUAL ACM SYMPOSIUM ON APPLIED COMPUTING, SAC 2024(2024)
摘要
The increasing demand for lithium-ion batteries (LIB) across various industries has accentuated the importance of accurately predicting the Remaining Useful Life (RUL) of these energy storage devices. This article introduces a novel approach to RUL prediction by leveraging a federated learning (FL) and homomorphic encryption (HE) model, called FedHEONN. Traditional RUL prediction models often face challenges not only related to the accuracy and reliability of estimations but also to data privacy and security when dealing with sensitive information in Internet of Things (IoT) environments. In response, our approach employs FL, allowing multiple distributed nodes to collaboratively train a predictive model without sharing private data. This ensures data privacy and security while harnessing the collective knowledge from diverse edge computing devices. Furthermore, to address the issue of secure computation over encrypted data, FedHEONN has the capacity to incorporate HE into the learning process. This enables the model to operate directly on encrypted data, providing an additional layer of protection to that of the federated model itself. Our experimental results test the efficacy of this FL method in accurately predicting the RUL of LIB real data against benchmark models, including linear regression with regularisation techniques such as Lasso, Ridge and Elastic-net, and non-linear models such as multilayer perceptron and support vector machine for regression. The experiment demonstrates the preservation of prediction outcomes, upholding an additional level of data safety through encrypted transmission of model information.
更多查看译文
关键词
Lithium-ion Batteries,Remaining Useful Life,Federated Learning,Homomorphic Encryption
AI 理解论文
溯源树
样例
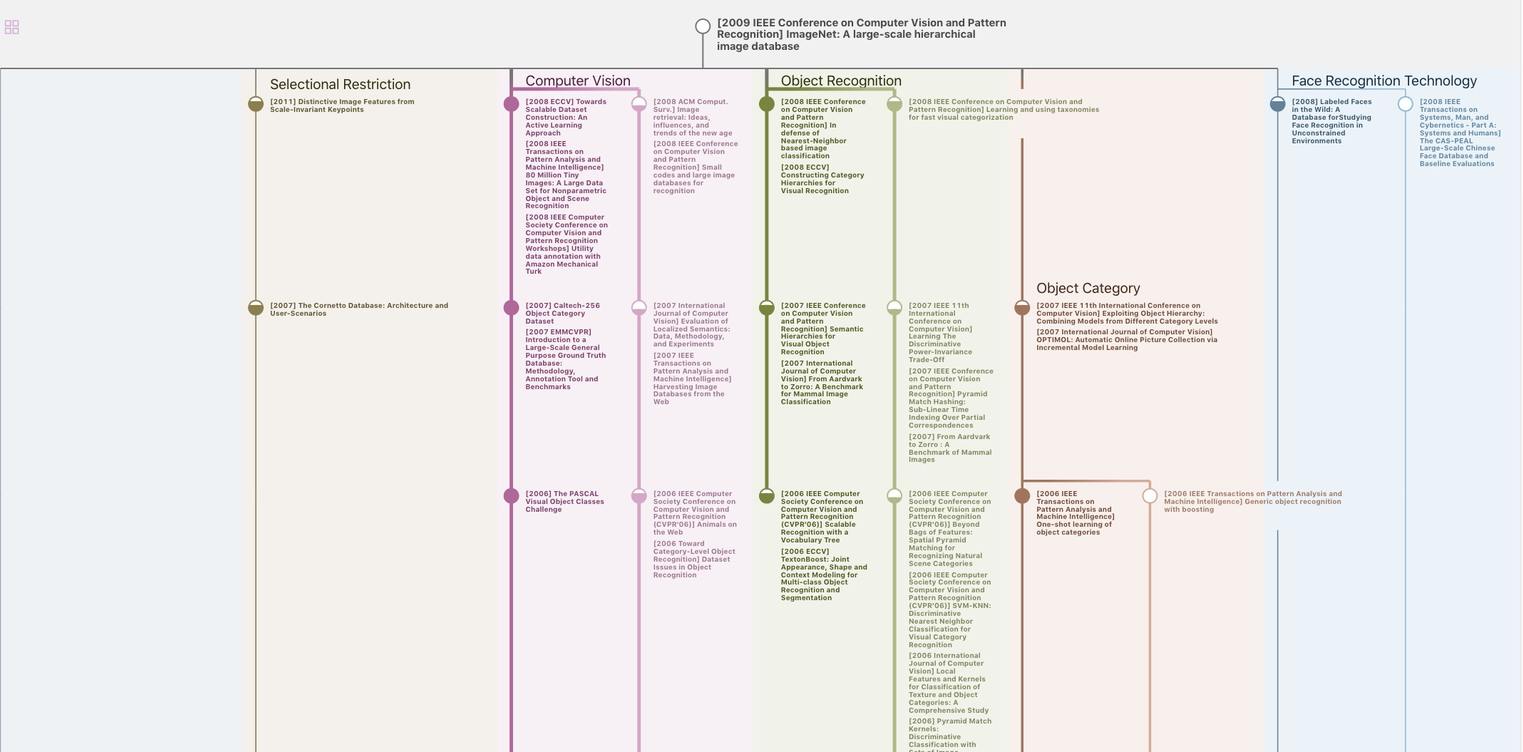
生成溯源树,研究论文发展脉络
Chat Paper
正在生成论文摘要