Improved Intrusion Detection System Using Machine Learning Techniques
2024 International Conference on Cognitive Robotics and Intelligent Systems (ICC - ROBINS)(2024)
摘要
The continuous demand for robust Intrusion Detection Systems (IDS) remains a constant in the ever-changing field of cybersecurity. This study aims to leverage the data obtained from Wireshark in order to build a sophisticated IDS. To achieve this goal, a specialized tool is utilized to generate network traffic and create a new dataset. By employing Machine Learning (ML) algorithms such as K-Nearest Neighbors, Random Forest Classifier and XGBoosts, study endeavors to classify network traffic and enhance network security. The performance evaluation metrics such as Recall, Precision, and F1 Score are employed to comprehensively analyze the system's performance. The novelty of this research lies in the utilization of a combination of machine learning algorithms to develop a comprehensive understanding of network behavior. Ultimately, this research not only expands knowledge within the field of intrusion detection but introduces an innovative solution using machine learning algorithms, advanced dataset, and thorough performance evaluation to enhance network security, this research establishes a resilient foundation for IDS by effectively safeguarding network infrastructures from evolving cyber threats.
更多查看译文
关键词
Cyber security,network traffic,XGboost,random forest classifier and knn
AI 理解论文
溯源树
样例
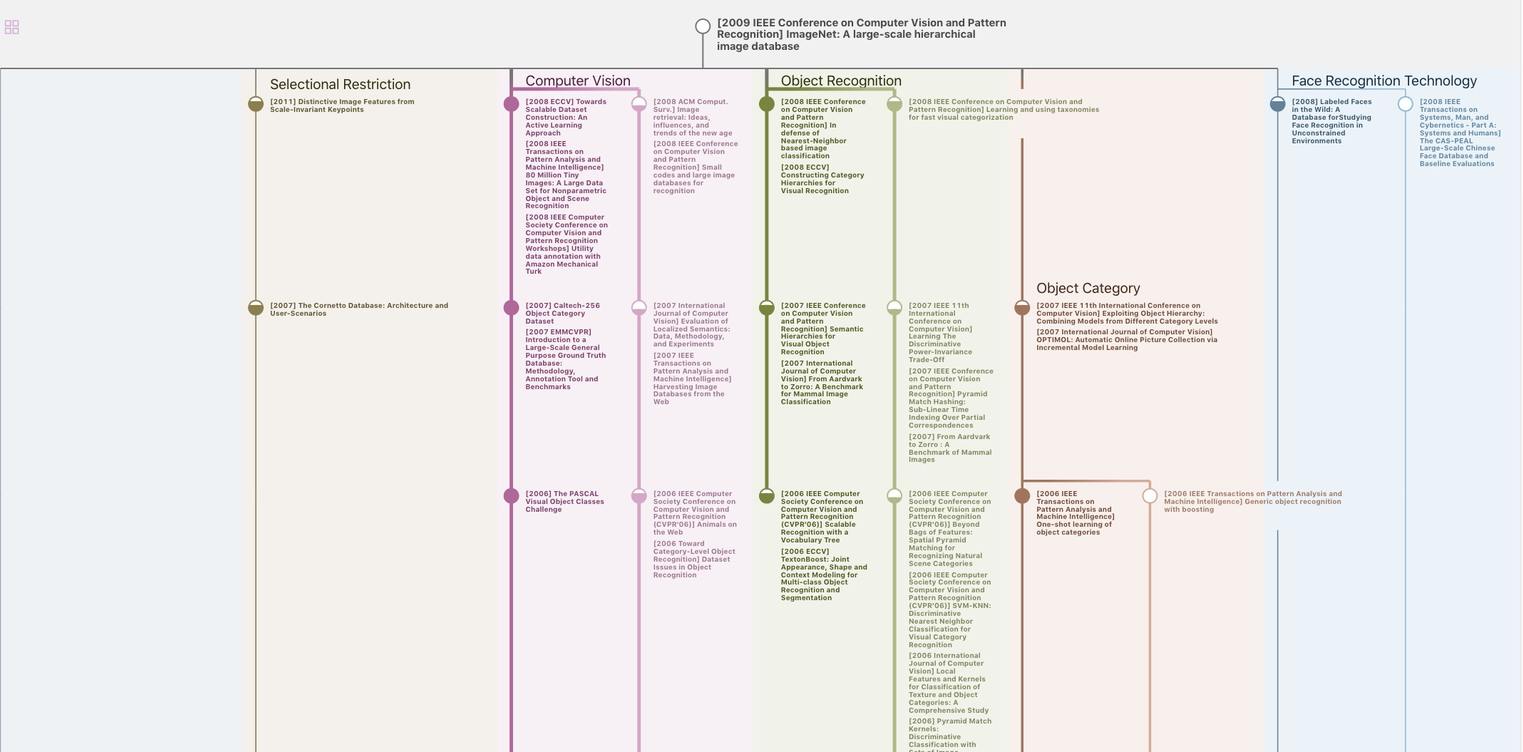
生成溯源树,研究论文发展脉络
Chat Paper
正在生成论文摘要