News Recommendation with Word-related Joint Topic Prediction
IEEE access(2024)
Abstract
As the problem of information overload becomes more severe, it has become increasingly difficult for users to browse news that they are interested in. News recommendation is an effective method to alleviate this problem. In news recommendation, accurately obtaining rich semantic news representations and modeling user’s historical interests are long-standing challenges. Based on these two issues, we propose a News recommendation approach with Word-related joint Topic prediction(NWT). The core of our approach is the topic perceptron and the news encoder that emphasizes word relevance. The topic information and word relevance information are fused through joint training, enhancing the abilities of topic prediction and news representation. In the news encoder, we employ multi-head self-attention network to emphasize word relevance, capturing the semantic relationships between any two words in the news title and enhancing the representation of the news. In the topic perceptron, we use the enhanced word relevance-based news representation as input and learn the topic information in the news by assigning weights to different topics. During joint training, the overall news recommendation module obtains the news topic information. Additionally, the user encoder learns the user’s topic preferences and utilizes attention networks to highlight news articles that are more representative of the user’s interests, thereby acquiring a more accurate user representation. We conduct extensive experiments on the MIND dataset, and the results demonstrate that NWT outperforms most existing baseline methods across various evaluation metrics.
MoreTranslated text
Key words
Semantics,Training,Recommender systems,Knowledge graphs,History,Media,Information integrity,Text processing,Text analysis,News recommendation,topic prediction,word-related,joint training
AI Read Science
Must-Reading Tree
Example
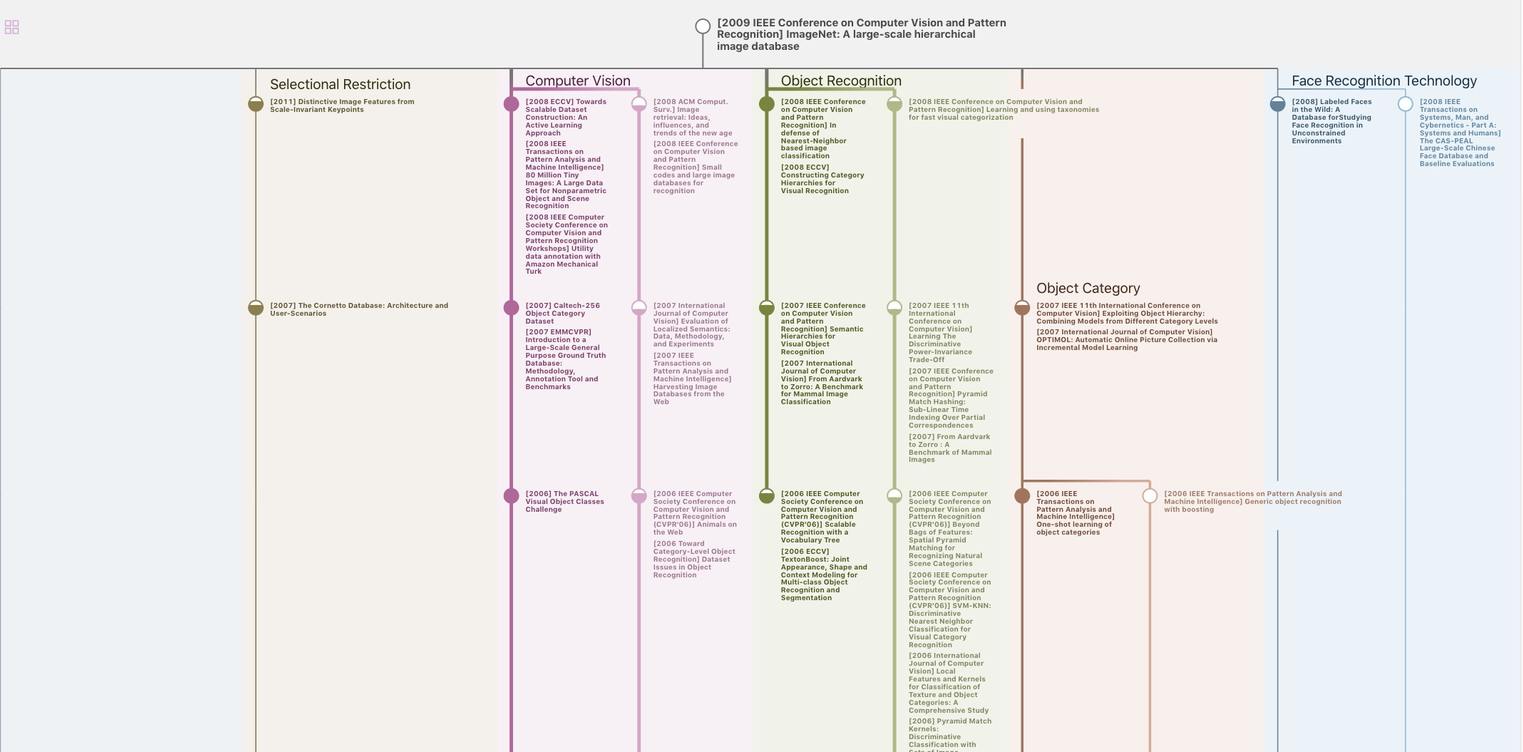
Generate MRT to find the research sequence of this paper
Chat Paper
Summary is being generated by the instructions you defined