BO-STA-LSTM: Building Energy Prediction Based on a Bayesian Optimized Spatial-Temporal Attention Enhanced LSTM Method
DEVELOPMENTS IN THE BUILT ENVIRONMENT(2024)
Abstract
In predicting building energy (affected by seasons), there are issues like inefficient hyperparameter optimization and inaccurate predictions, it is unclear whether spatial and temporal attention improves performance. This study proposes a method based on Bayesian Optimization (BO), Spatial-Temporal Attention (STA), and Long Short-Term Memory (LSTM). Seven improved LSTM models (BO-LSTM, SA-LSTM, TA-LSTM, STA-LSTM, BO-SA-LSTM, BO-TA-LSTM, and BO-STA-LSTM) are compared with LSTM and the impacts of seasonal variations on BO-STA-LSTM are analysed using different sample types and time domain analysis. To further demonstrate the efficiency of the proposed method, comparisons with convolutional neural network (CNN) and temporal convolutional network (TCN) are performed, and followed by validation with new datasets. The findings indicate that adding STA and BO to LSTM enhances the average R2 of prediction performance by 0.0885. BO alone contributes 0.0717, while adding attention improves 0.0560. BO-STA-LSTM achieves higher prediction accuracy for similar test and training samples or a test sample size of 14,016, effectively capturing seasonal, trend, and peak energy patterns. Additionally, BO-STA-LSTM outperforms CNN and TCN, demonstrating superior prediction accuracy.
MoreTranslated text
Key words
Building energy predictions,Bayesian optimization (BO),Spatial and temporal attention mechanisms,Long short-term memory (LSTM),Seasonal variations
AI Read Science
Must-Reading Tree
Example
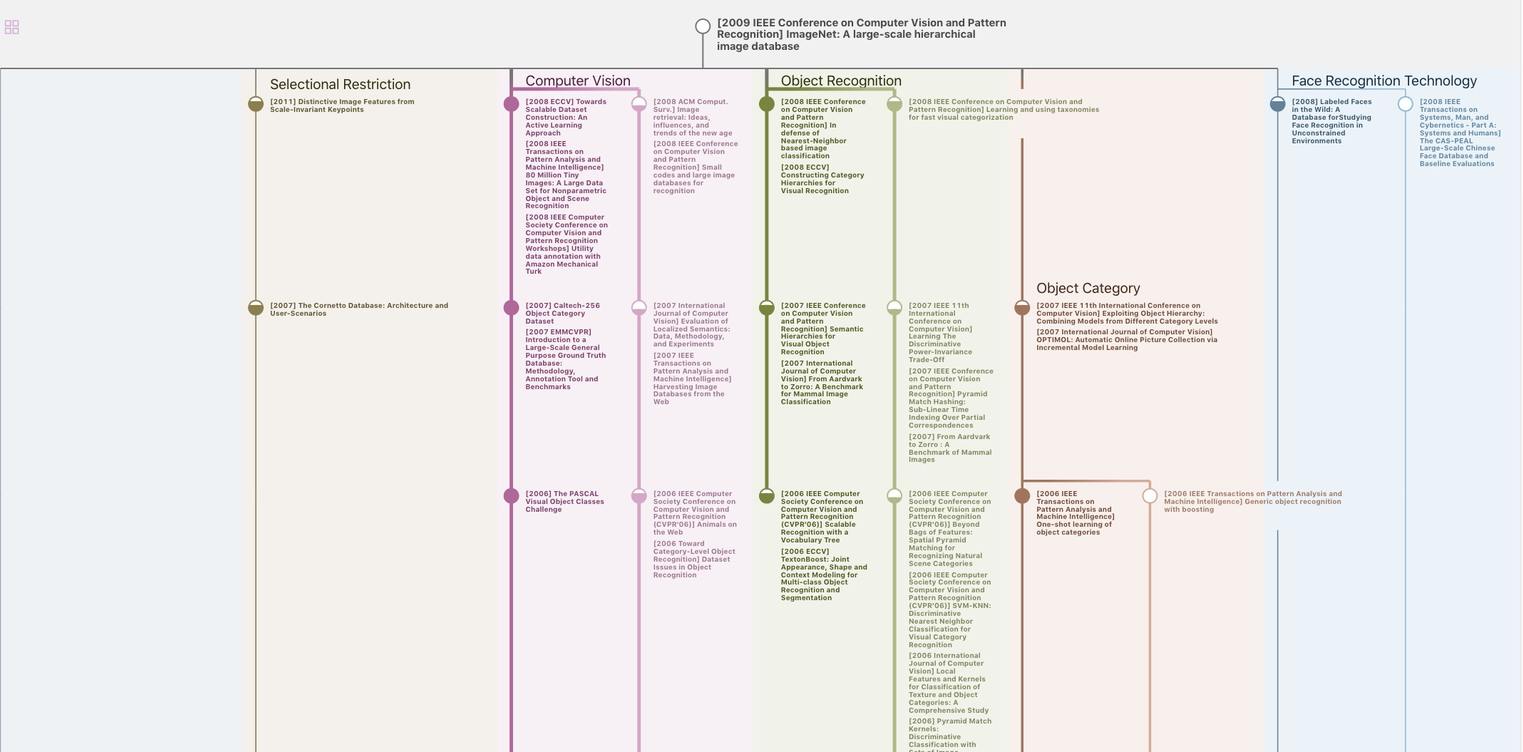
Generate MRT to find the research sequence of this paper
Chat Paper
Summary is being generated by the instructions you defined