Gait Activity Classification with Convolutional Neural Network Using Lower Limb Angle Measurement from Inertial Sensors
IEEE sensors journal(2024)
摘要
Human gait activity recognition can be crucial to adapt the assistance provided by lower limb exoskeletons, as well as for biomechanical analysis. With this purpose, Deep Learning techniques can be applied to develop a classifier based on the acquisition of the lower limb kinematics. In this paper, we present a one-dimensional Convolutional Neural Network (CNN) to classify different activities from the hip, knee, and ankle flexion/extension angles, measured with wearable inertial sensors. The proposed CNN classifier achieves 99.56% accuracy with users not involved in the learning process. In addition, the Gradient-weighted Class Activation-Map (Grad-CAM) and the t-Distributed Stochastic Neighbor Embedding (t-SNE) were used to understand the CNN model decision-making. Finally, how the accuracy of the CNN model is impacted by input reduction was analyzed to adapt the CNN model to multiple situations, and it can be concluded that the CNN maintains high accuracy with a single joint angle as input.
更多查看译文
关键词
Gait analysis,human activity recognition,magneto-inertial devices,Deep Learning
AI 理解论文
溯源树
样例
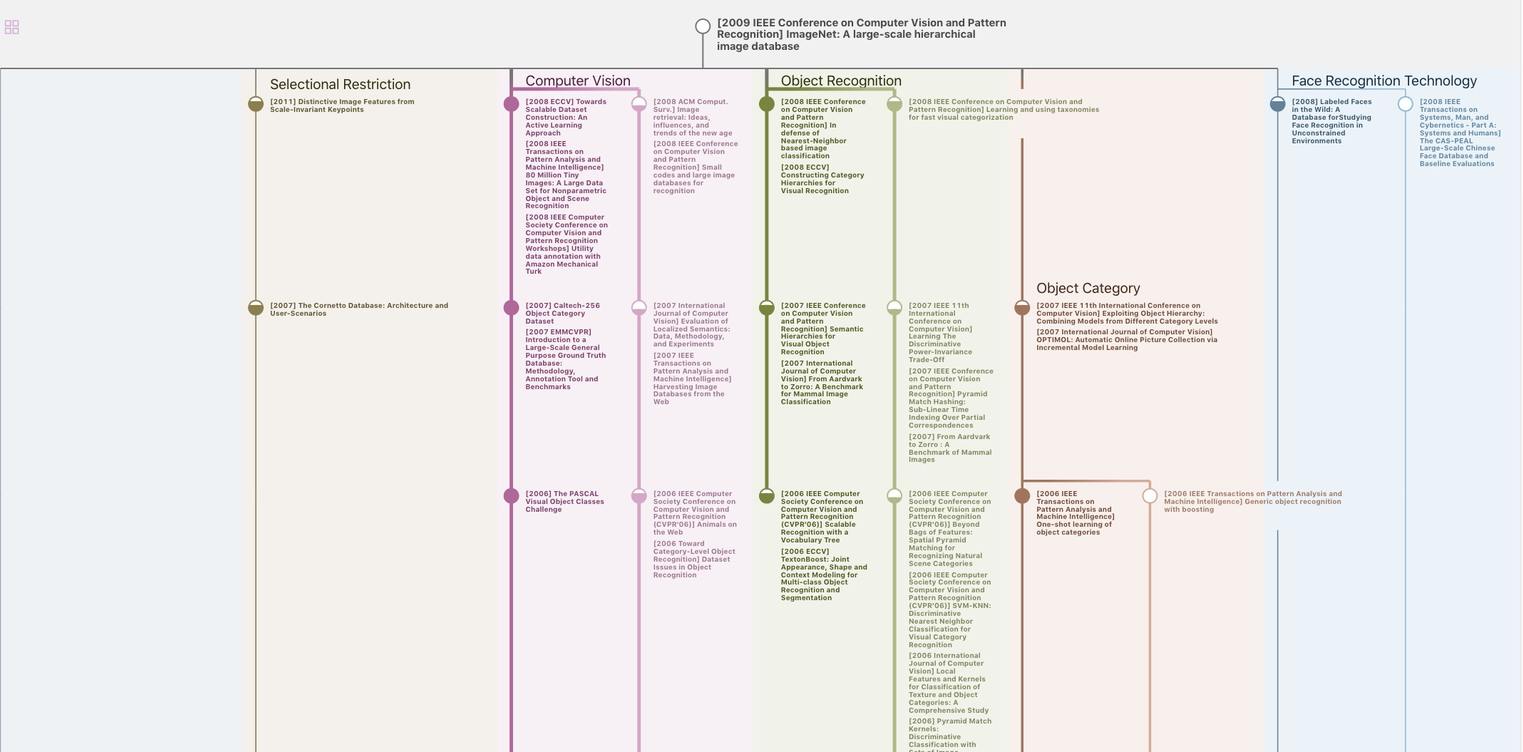
生成溯源树,研究论文发展脉络
Chat Paper
正在生成论文摘要