Classifying Routine Clinical Electroencephalograms with Multivariate Iterative Filtering and Convolutional Neural Networks
IEEE TRANSACTIONS ON NEURAL SYSTEMS AND REHABILITATION ENGINEERING(2024)
摘要
Electroencephalogram (EEG) is widely used in basic and clinical neuroscience to explore neural states in various populations, and classifying these EEG recordings is a fundamental challenge. While machine learning shows promising results in classifying long multivariate time series, optimal prediction models and feature extraction methods for EEG classification remain elusive. Our study addressed the problem of EEG classification under the framework of brain age prediction, applying a deep learning model on EEG time series. We hypothesized that decomposing EEG signals into oscillatory modes would yield more accurate age predictions than using raw or canonically frequency-filtered EEG. Specifically, we employed multivariate intrinsic mode functions (MIMFs), an empirical mode decomposition (EMD) variant based on multivariate iterative filtering (MIF), with a convolutional neural network (CNN) model. Testing a large dataset of routine clinical EEG scans (n = 6540) from patients aged 1 to 103 years, we found that an ad-hoc CNN model without fine-tuning could reasonably predict brain age from EEGs. Crucially, MIMF decomposition significantly improved performance compared to canonical brain rhythms (from delta to lower gamma oscillations). Our approach achieved a mean absolute error (MAE) of 13.76 ± 0.33 and a correlation coefficient of 0.64 ± 0.01 in brain age prediction over the entire lifespan. Our findings indicate that CNN models applied to EEGs, preserving their original temporal structure, remains a promising framework for EEG classification, wherein the adaptive signal decompositions such as the MIF can enhance CNN models' performance in this task.
更多查看译文
关键词
Convolutional neural network,EEG,multivariate iterative filtering,brain-age,MIMF
AI 理解论文
溯源树
样例
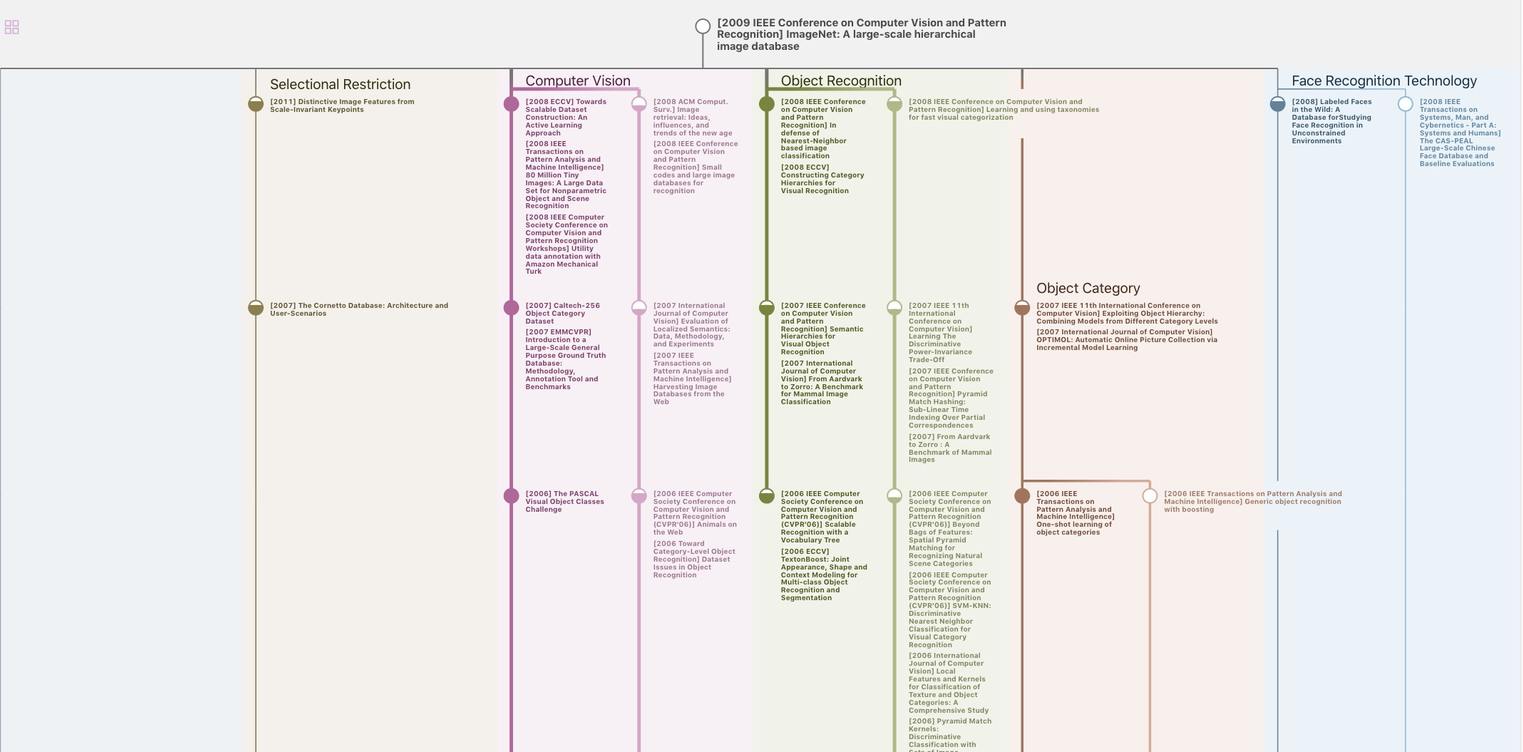
生成溯源树,研究论文发展脉络
Chat Paper
正在生成论文摘要