Unrolled Optimization Via Physics-assisted Convolutional Neural Network for MR-based Electrical Properties Tomography: a Numerical Investigation
IEEE open journal of engineering in medicine and biology(2024)
摘要
Magnetic Resonance imaging based Electrical Properties Tomography (MR-EPT) is a non-invasive technique that measures the electrical properties (EPs) of biological tissues. In this work, we present and numerically investigate the performance of an unrolled, physics-assisted method for 2D MR-EPT reconstructions, where a cascade of Convolutional Neural Networks is used to compute the contrast update. Each network takes in input the EPs and the gradient descent direction (encoding the physics underlying the adopted scattering model) and returns as output the updated contrast function. The network is trained and tested in silico using 2D slices of realistic brain models at 128 MHz. Results show the capability of the proposed procedure to reconstruct EPs maps with quality comparable to that of the popular Contrast Source Inversion-EPT, while significantly reducing the computational time.
更多查看译文
关键词
Convolutional neural network,electrical properties,inverse scattering problems,learning methods,magnetic resonance imaging
AI 理解论文
溯源树
样例
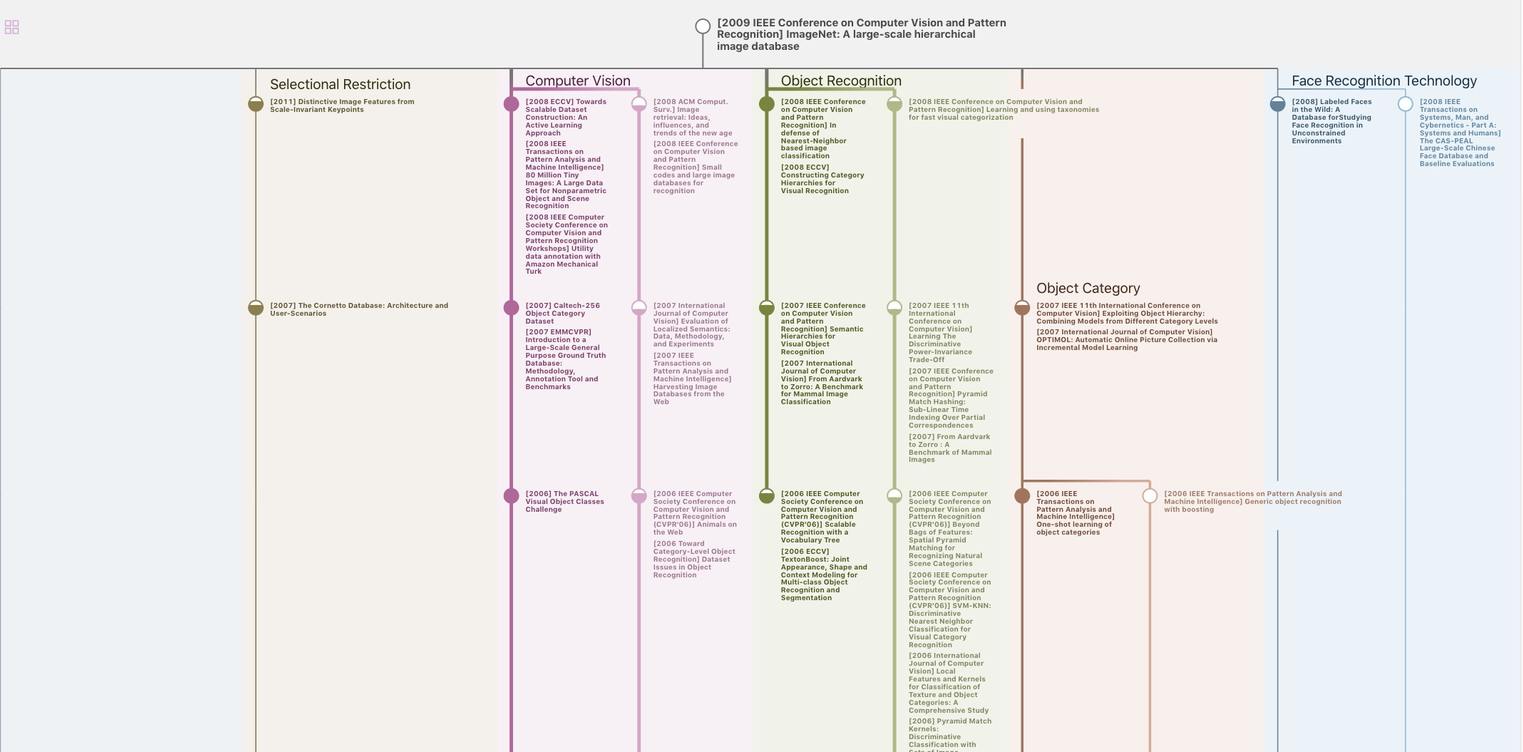
生成溯源树,研究论文发展脉络
Chat Paper
正在生成论文摘要