Metric Learning-Based Few-Shot Adversarial Domain Adaptation: A Cross-Machine Diagnosis Method for Ball Screws of Industrial Robots.
IEEE Trans. Instrum. Meas.(2024)
摘要
Due to the varying working conditions of SCARA (Selective Compliance Assembly Robot Arm) robots, there are significant differences in data distribution among different machines. As a result, it is challenging to apply unsupervised methods for cross-machine fault diagnosis. This paper proposes a method called Metric Learning-based Few-shot Adversarial Domain Adaptation (MLFADA) for cross-machine diagnosis of the SCARA robot’s ball screws. Firstly, MLFADA constructs data pairs by sampling a few shots of samples from the source domain (named SCARA A) and the target domain (named SCARA B). Subsequently, it integrates metric learning and adversarial learning theories to minimize the distance between data pairs that belong to the same class in both domains while maximizing the distance between data pairs from different classes. Secondly, to further enhance the performance of MLFADA, a strategy called Pseudo-label Self-correcting Maximum Mean Discrepancy (PSMMD) is proposed to reduce the conditional distribution differences between the two domains. Finally, a lightweight network is designed for feature extraction and fault classification to facilitate deployment on terminal devices. The experiment demonstrates that the challenge of cross-machine fault diagnosis for the ball screws of SCARA robots has been successfully resolved. This is a relatively understudied problem. Compared to mainstream domain adaptation and few-shot methods, the proposed method achieved the best diagnostic accuracy of 88.14%, even when there was only one labeled sample available in the target domain.
更多查看译文
关键词
Ball screw,cross-machine diagnosis,few-shot,adversarial domain adaptation,lightweight
AI 理解论文
溯源树
样例
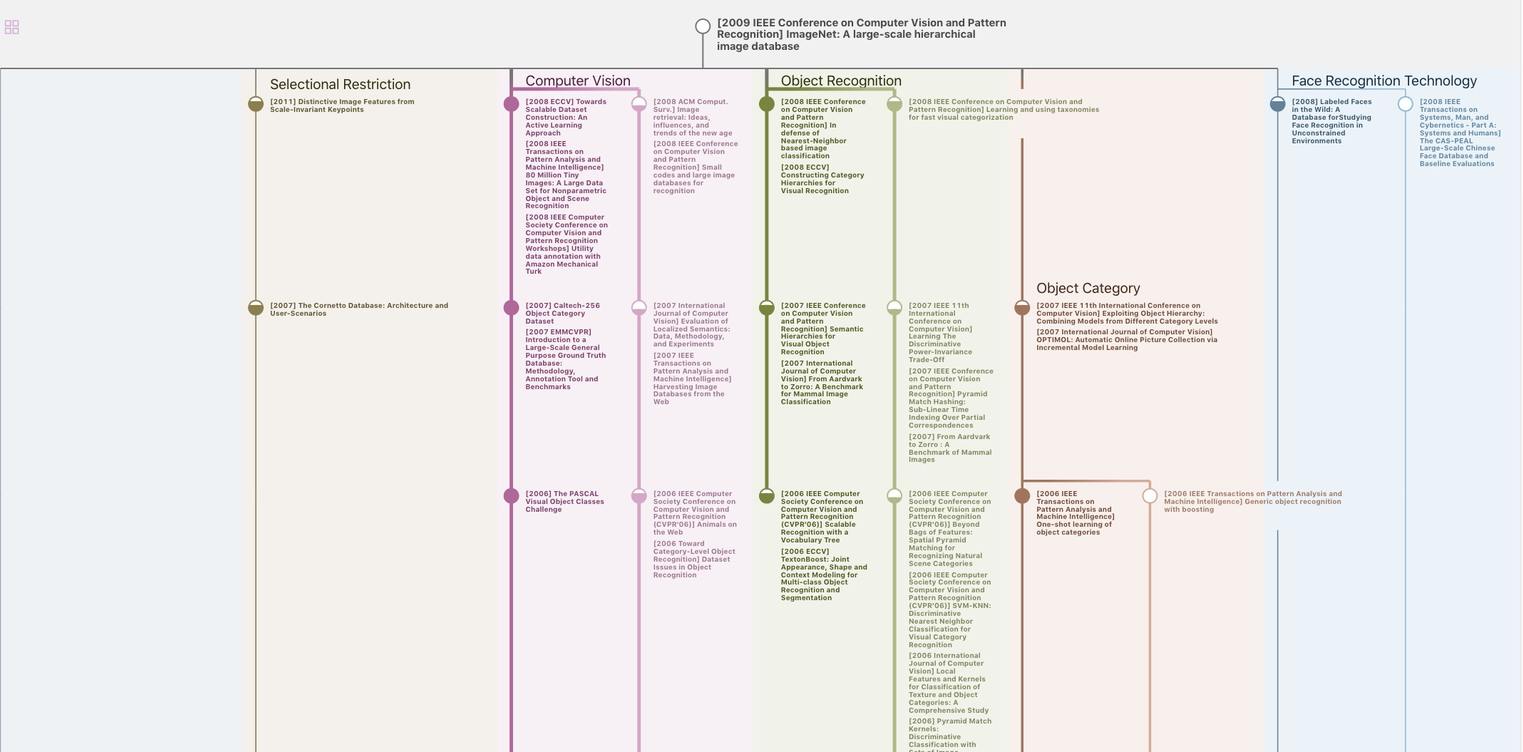
生成溯源树,研究论文发展脉络
Chat Paper
正在生成论文摘要