The phobic brain: morphometric features correctly classify individuals with small animal phobia
crossref(2024)
摘要
Specific phobia represents an anxiety disorder category characterized by intense fear generated by distinct stimuli or situational triggers. Among specific phobias, small animal phobia (SAP) denotes a particular condition that has been poorly investigated in the neuroscientific literature. Moreover, the few previous studies on this topic have mostly employed univariate analyses, with limited and unbalanced samples, often leading to inconsistent results. To overcome these limitations, and to characterize the neural underpinnings of SAP, this study aims to rely on a machine learning method known as Binary Support Vector Machine (BSVM), to develop a classification model of individuals with small animal phobia based on grey matter features. Moreover, the contribution of specific structural macro-networks, such as the Default Mode, the Salience, the Executive, and the Affective networks, in separating phobic subjects from controls was assessed. Thirty-two subjects with SAP and ninety matched healthy controls were tested to this aim. At a whole-brain level, we found a significant predictive model including brain structures related to emotional regulation, cognitive control, and sensory integration, such as the cerebellum, the temporal pole, the frontal cortex, temporal lobes, the amygdala and the thalamus. Instead, when considering macro-networks analysis, we found the Default Mode, the Affective, the Central Executive and the Sensorimotor networks to significantly outperform the other networks in classifying SAP individuals. In conclusion, this study expands knowledge about the neural basis of small animal phobia, proposing new research directions and potential diagnostic strategies using advanced machine learning methods applied to neuroimaging data.
更多查看译文
AI 理解论文
溯源树
样例
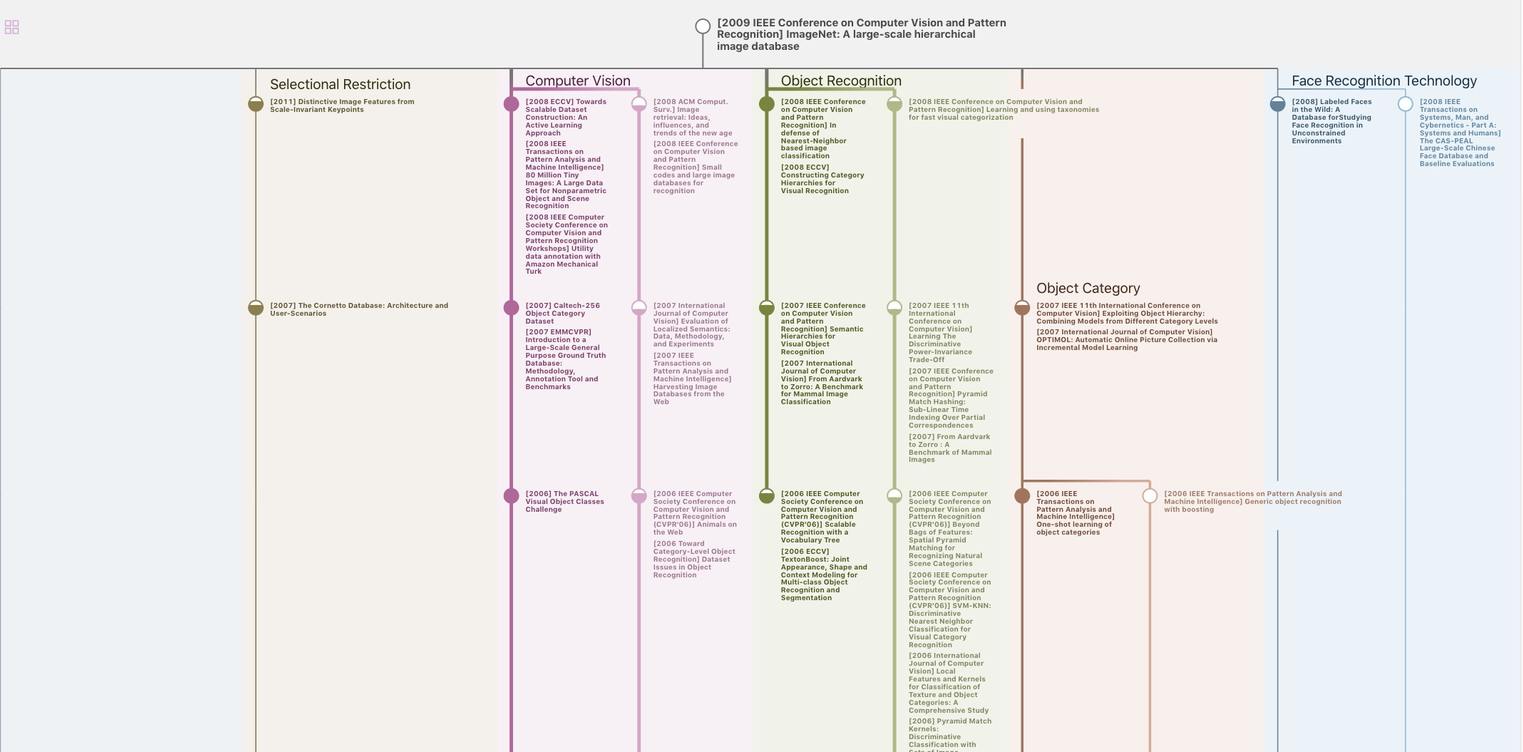
生成溯源树,研究论文发展脉络
Chat Paper
正在生成论文摘要