Metabolomic Prediction of Severe Maternal and Newborn Complications in Preeclampsia
Metabolomics(2024)SCI 3区
Drexel College of Medicine | Beaumont Health System | Oakland University School of Medicine | Wayne State University-Detroit Medical Center | Depaul University
Abstract
Preeclampsia (PreE) remains a major source of maternal and newborn complications. Prenatal prediction of these complications could significantly improve pregnancy management. Using metabolomic analysis we investigated the prenatal prediction of maternal and newborn complications in early and late PreE and investigated the pathogenesis of such complications. Serum samples from 76 cases of PreE (36 early-onset and 40 late-onset), and 40 unaffected controls were collected. Direct Injection Liquid Chromatography–Mass Spectrometry combined with Nuclear Magnetic Resonance (NMR) spectroscopy was performed. Logistic regression analysis was used to generate models for prediction of adverse maternal and neonatal outcomes in patients with PreE. Metabolite set enrichment analysis (MSEA) was used to identify the most dysregulated metabolites and pathways in PreE. Forty-three metabolites were significantly altered (p < 0.05) in PreE cases with maternal complications and 162 metabolites were altered in PreE cases with newborn adverse outcomes. The top metabolite prediction model achieved an area under the receiver operating characteristic curve (AUC) = 0.806 (0.660–0.952) for predicting adverse maternal outcomes in early-onset PreE, while the AUC for late-onset PreE was 0.843 (0.712–0.974). For the prediction of adverse newborn outcomes, regression models achieved an AUC = 0.828 (0.674–0.982) in early-onset PreE and 0.911 (0.828–0.994) in late-onset PreE. Profound alterations of lipid metabolism were associated with adverse outcomes. Prenatal metabolomic markers achieved robust prediction, superior to conventional markers for the prediction of adverse maternal and newborn outcomes in patients with PreE. We report for the first-time the prediction and metabolomic basis of adverse maternal and newborn outcomes in patients with PreE.
MoreTranslated text
Key words
Metabolomics,Preeclampsia,Adverse outcomes,Nuclear magnetic resonance,Mass spectrometry
PDF
View via Publisher
AI Read Science
AI Summary
AI Summary is the key point extracted automatically understanding the full text of the paper, including the background, methods, results, conclusions, icons and other key content, so that you can get the outline of the paper at a glance.
Example
Background
Key content
Introduction
Methods
Results
Related work
Fund
Key content
- Pretraining has recently greatly promoted the development of natural language processing (NLP)
- We show that M6 outperforms the baselines in multimodal downstream tasks, and the large M6 with 10 parameters can reach a better performance
- We propose a method called M6 that is able to process information of multiple modalities and perform both single-modal and cross-modal understanding and generation
- The model is scaled to large model with 10 billion parameters with sophisticated deployment, and the 10 -parameter M6-large is the largest pretrained model in Chinese
- Experimental results show that our proposed M6 outperforms the baseline in a number of downstream tasks concerning both single modality and multiple modalities We will continue the pretraining of extremely large models by increasing data to explore the limit of its performance
Try using models to generate summary,it takes about 60s
Must-Reading Tree
Example
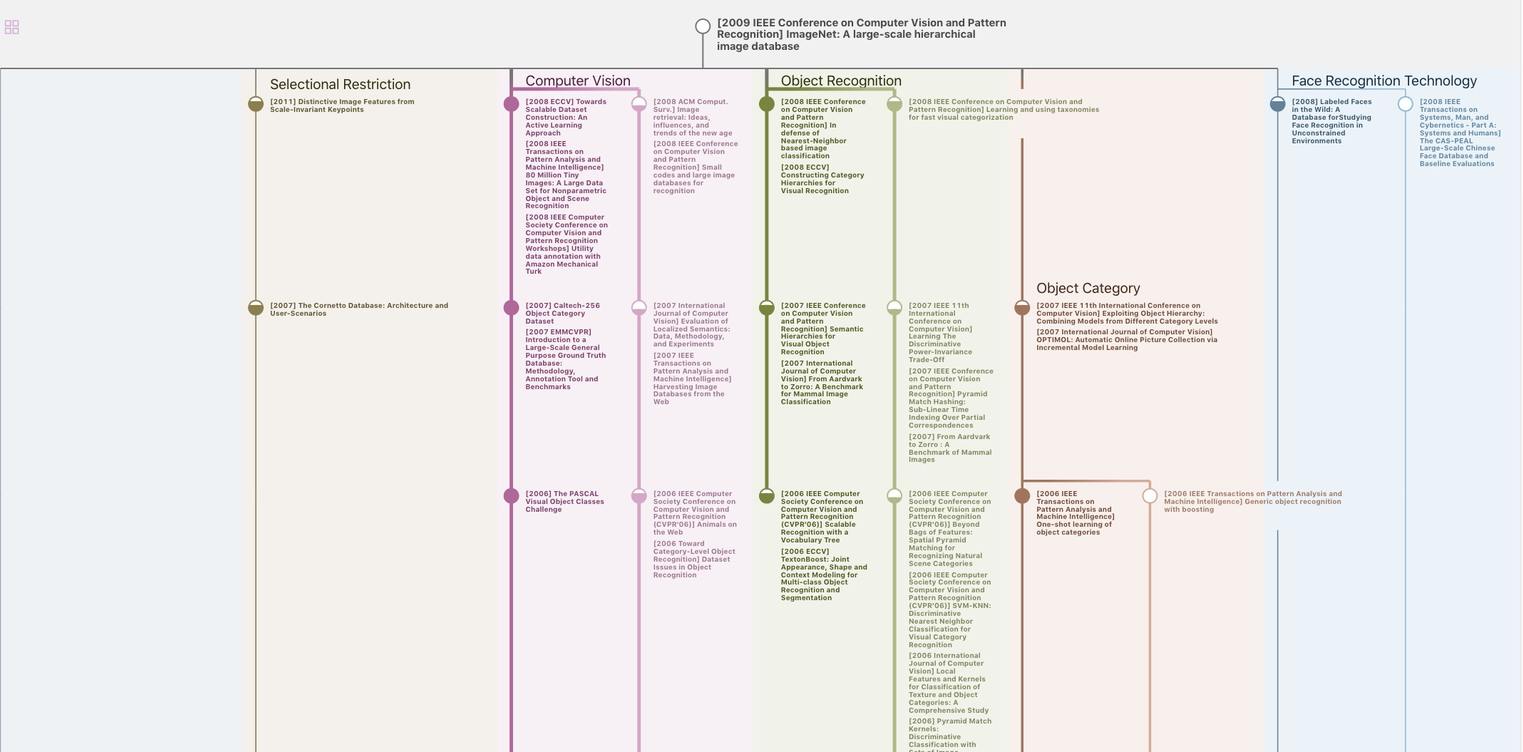
Generate MRT to find the research sequence of this paper
Related Papers
2007
被引用63 | 浏览
2001
被引用118 | 浏览
2011
被引用226 | 浏览
2009
被引用224 | 浏览
2013
被引用101 | 浏览
2014
被引用16 | 浏览
2014
被引用59 | 浏览
2015
被引用256 | 浏览
2016
被引用171 | 浏览
2017
被引用33 | 浏览
Applications of Metabolomics in the Study and Management of Preeclampsia: a Review of the Literature
2017
被引用35 | 浏览
2015
被引用135 | 浏览
2022
被引用12 | 浏览
2022
被引用6 | 浏览
2014
被引用15 | 浏览
Data Disclaimer
The page data are from open Internet sources, cooperative publishers and automatic analysis results through AI technology. We do not make any commitments and guarantees for the validity, accuracy, correctness, reliability, completeness and timeliness of the page data. If you have any questions, please contact us by email: report@aminer.cn
Chat Paper
去 AI 文献库 对话