Problem-Specific Knowledge Based Multi-Objective Meta-Heuristics Combined Q-Learning for Scheduling Urban Traffic Lights with Carbon Emissions
IEEE TRANSACTIONS ON INTELLIGENT TRANSPORTATION SYSTEMS(2024)
关键词
Delays,Pedestrians,Metaheuristics,Carbon dioxide,Search problems,Job shop scheduling,Q-learning,Traffic light scheduling,delay,carbon emissions,meta-heuristics
AI 理解论文
溯源树
样例
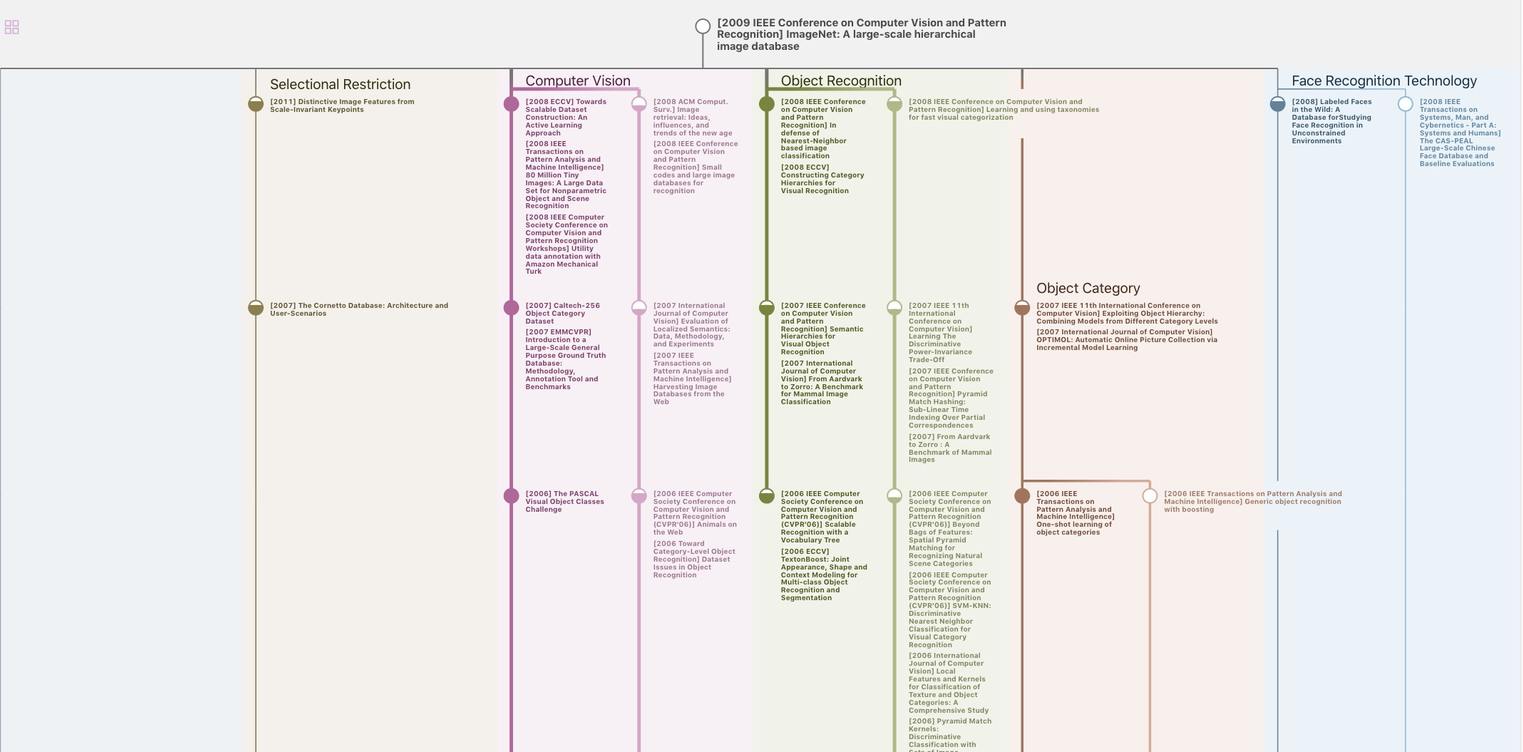
生成溯源树,研究论文发展脉络
Chat Paper
正在生成论文摘要