Exploring Innovative Approaches to Synthetic Tabular Data Generation
Electronics(2024)
摘要
The rapid advancement of data generation techniques has spurred innovation across multiple domains. This comprehensive review delves into the realm of data generation methodologies, with a keen focus on statistical and machine learning-based approaches. Notably, novel strategies like the divide-and-conquer (DC) approach and cutting-edge models such as GANBLR have emerged to tackle a spectrum of challenges, spanning from preserving intricate data relationships to enhancing interpretability. Furthermore, the integration of generative adversarial networks (GANs) has sparked a revolution in data generation across sectors like healthcare, cybersecurity, and retail. This review meticulously examines how these techniques mitigate issues such as class imbalance, data scarcity, and privacy concerns. Through a meticulous analysis of evaluation metrics and diverse applications, it underscores the efficacy and potential of synthetic data in refining predictive models and decision-making software. Concluding with insights into prospective research trajectories and the evolving role of synthetic data in propelling machine learning and data-driven solutions across disciplines, this work provides a holistic understanding of the transformative power of contemporary data generation methodologies.
更多查看译文
关键词
data generation,synthetic data,machine learning-based generation,statistical-based generation,healthcare,privacy preservation
AI 理解论文
溯源树
样例
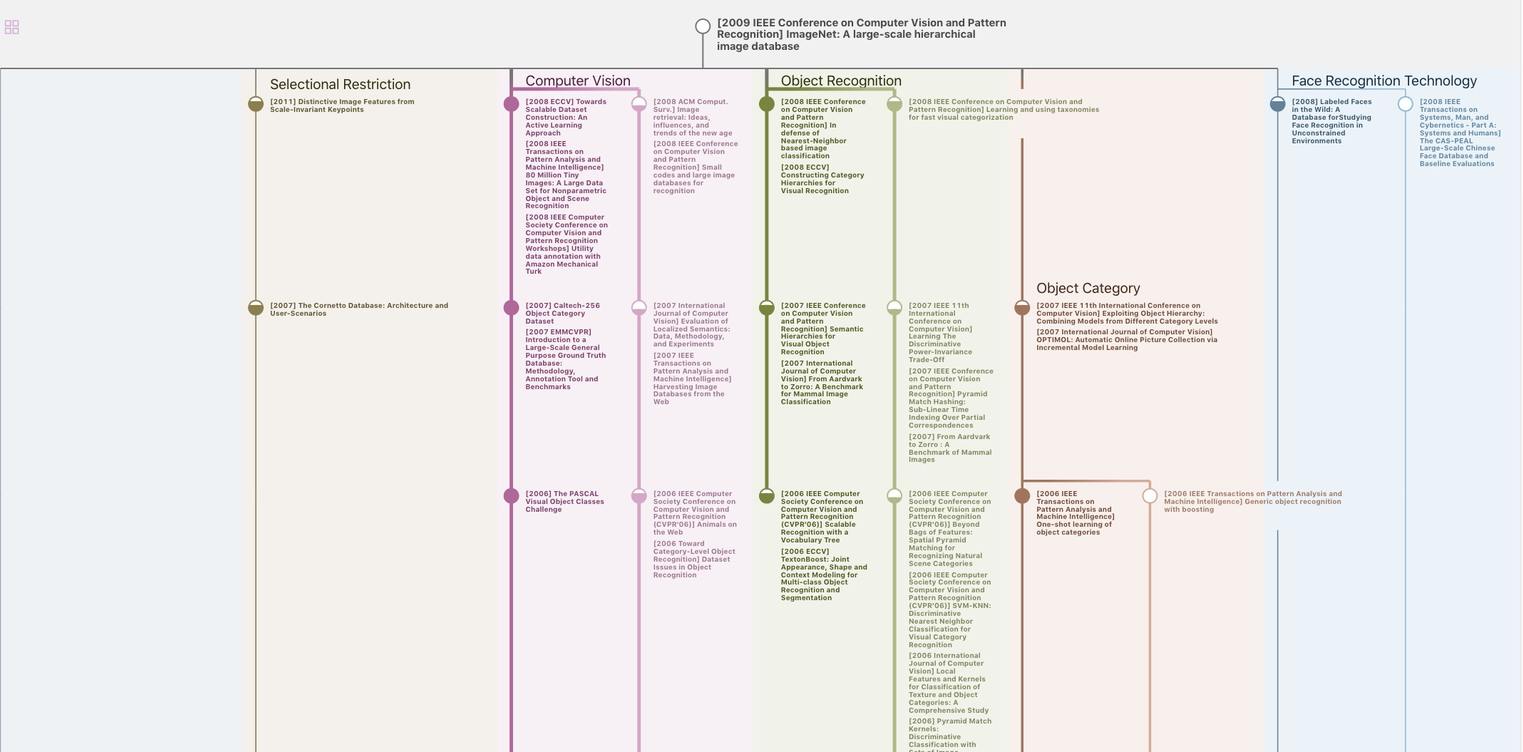
生成溯源树,研究论文发展脉络
Chat Paper
正在生成论文摘要