Scalable Learning for Multi-Agent Route Planning: Adapting to Diverse Task Scales
IEEE Transactions on Artificial Intelligence(2024)
摘要
When utilizing end-to-end learn-to-construct methods to solve routing problems for multi-agent systems, the model is usually trained individually for different problem scales (i.e., the number of customers to be concurrently served within a map) to make the model adaptive to the corresponding scale, ensuring good solution quality. Otherwise, the model trained for one specific scale can lead to poor performance when applied to another different scale, and this situation can get worse when the scale discrepancy increases. Such a separate training strategy is inefficient and time-intensive. In this paper, we propose a Mix-scale learning framework that requires only a single training session, enabling the model to effectively plan high-quality routes for various problem scales. Based on the capacitated vehicle routing problem (CVRP), the test results reveal that: for problem scales which are no matter seen or unseen during training, our once-trained model can produce solution routes with performance comparable or even superior to those of individually trained models, and offer the highest average solution quality with improvement ratio ranging from 2.28% to 8.07%, which effectively spares the separate training session for each specific scale. Additionally, the extended comparison analysis with individually trained models on real-world benchmark dataset from CVRPLib further highlights our once-trained model’s generalization performance across various problem scales and diverse node distributions.
更多查看译文
关键词
Routing Problem,Hypernetworks,Reinforcement learning
AI 理解论文
溯源树
样例
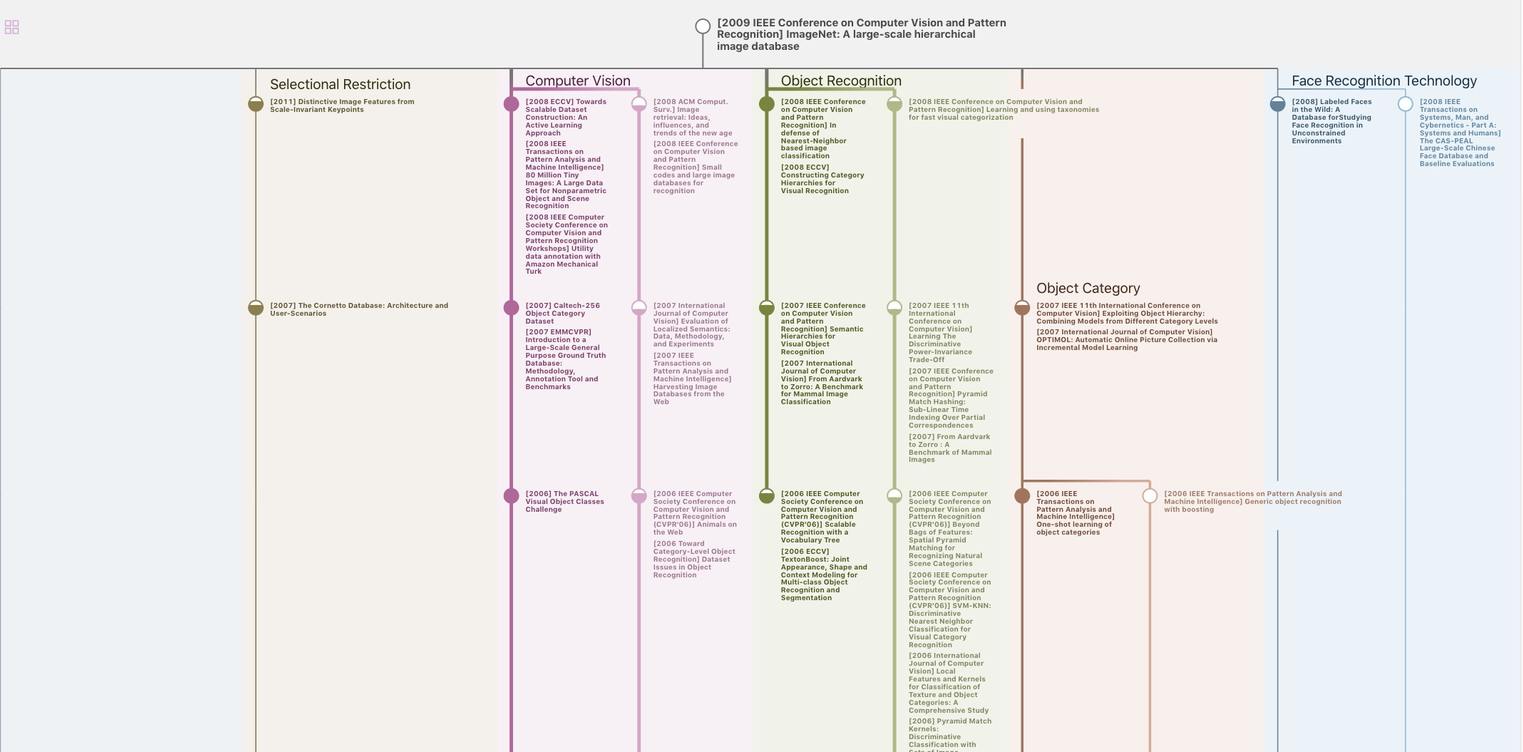
生成溯源树,研究论文发展脉络
Chat Paper
正在生成论文摘要