Hyperspectral Image Super-Resolution with ConvLSTM Skip-Connections
IEEE TRANSACTIONS ON GEOSCIENCE AND REMOTE SENSING(2024)
摘要
Hyperspectral image super-resolution has been extensively studied, and significant development has been made based on deep convolutional neural networks. Particularly, residual networks that fuse features from multiple layers have achieved high accuracy hyperspectral image super-resolution. However, most residual networks tend to straightforwardly add features from one layer to another through skip-connections that may cause confusion to feature fusion. To tackle this issue, we develop a ConvLSTM skip-connection strategy that characterizes features from consecutive layers by ConvLSTMs and renders feature fusion in a more principal manner. Accordingly, we develop a super-resolution framework that consists of three modules. The first module, i.e., spatial feature reconstruction, employs the ConvLSTM skip-connections to comprehensively fuse spatial features from different layers. The second module, i.e., edge refinement, involves the ConvLSTM skip-connections to enhance the edge information from intermediate results. The third module, i.e., spectral information reconstruction, refines spectral features by capturing interactions between different spectral bands through the ConvLSTM skip-connections. The three complementary modules cooperate such that both spatial resolution and spectral fidelity are well maintained. Extensive experimental results on the Chikusei, Houston, and QUST-1 datasets demonstrate that our framework outperforms state-of-the-art methods in terms of quantitative evaluation and visual quality across a variety of scenarios. We release our source code at https://gitee.com/xu yinghao/CLSCNet for public evaluations.
更多查看译文
关键词
Super-resolution,hyperspectral image,ConvLSTM,feature fusion,edge enhancement
AI 理解论文
溯源树
样例
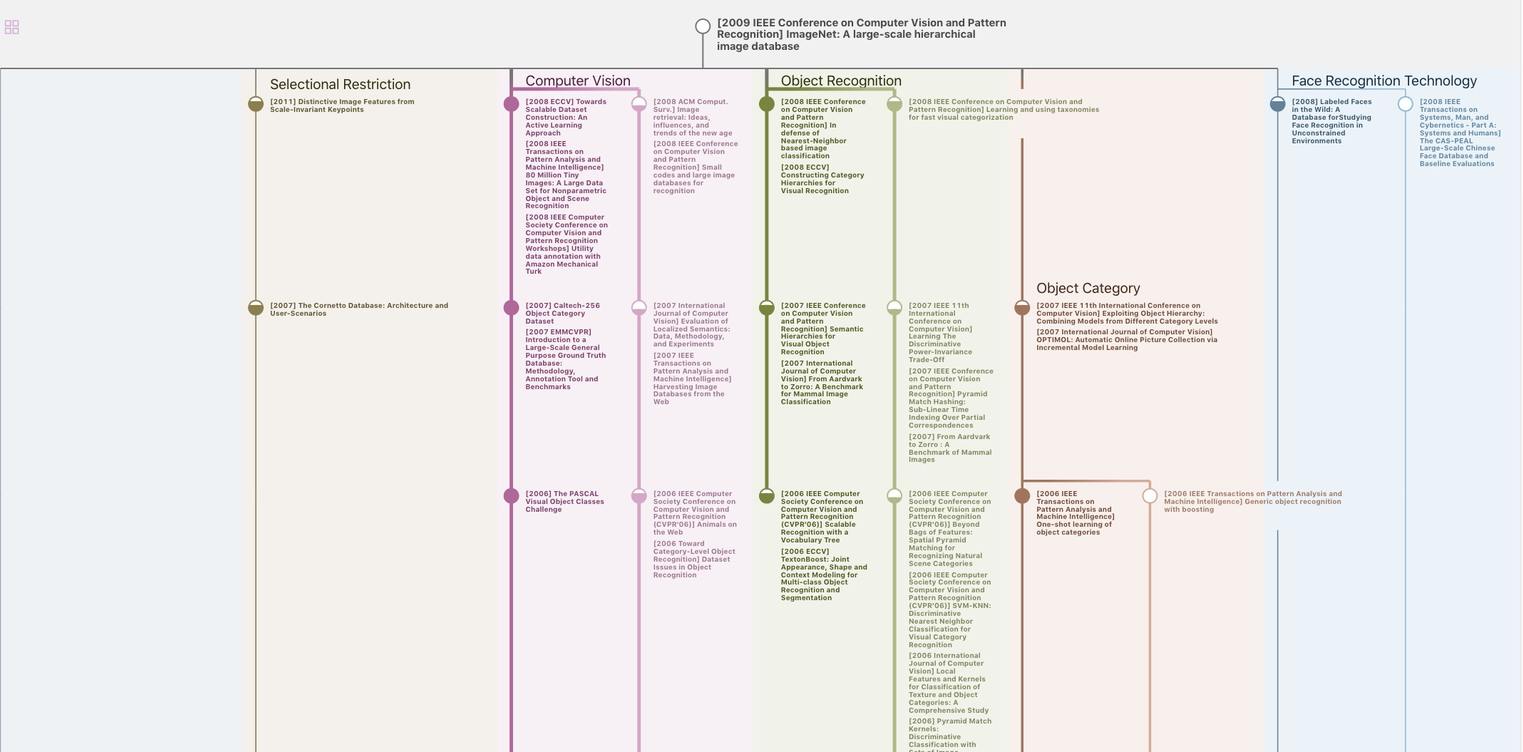
生成溯源树,研究论文发展脉络
Chat Paper
正在生成论文摘要