A Novel Deep Learning Approach for Myocardial Infarction Detection and Multi-Label Classification
IEEE access(2024)
摘要
One of the main causes of death from cardiovascular diseases is Myocardial Infarction (MI), which is brought on by coronary artery problems. Myocardial infarction is a pathological condition resulting from an anatomical issue with the Left Ventricle (LV). MI is a potentially fatal cardiac disease for which prompt medical attention can lower the fatality risk. This paper proposes a Deep Learning (DL) based approach to robustly detect binary and multiclass myocardial infarction (MI) in two environments, i.e., with and without data balancing. We employed a Myocardial infarction dataset that contains 1700 MI patients’ medical records. The data preprocessing step is performed, during which data balancing and normalization are carried out. In many real-world medical datasets, class imbalance is a serious problem since it often causes the proposed algorithms to predict the majority class. We apply a class imbalance handling technique to solve the imbalance issue and create a balanced and trustworthy prediction approach. To ensure the generalizability and performance comparison, we employ various deep learning algorithms such as Recurrent Neural Network (RNN), Convolutional Neural Network (CNN), Deep Neural Network (DNN), Long Short-Term Memory (LSTM) without data balancing first, and then after that, with data balancing to enhance model performance. Experiments reveal that the DNN model outperformed others by applying the class imbalance handling technique compared to the method without balancing data. The DNN model attained a maximum test accuracy of 99.39% and a test loss of 0.0252 for the binary class and achieved a maximum test accuracy of 99.74% and a test loss of 0.0115 for multiclass categorization.
更多查看译文
关键词
Myocardial infraction,cardiovascular disease,deep learning,convolutional neural network,recurrent neural network,deep neural network,long short term memory,healthcare
AI 理解论文
溯源树
样例
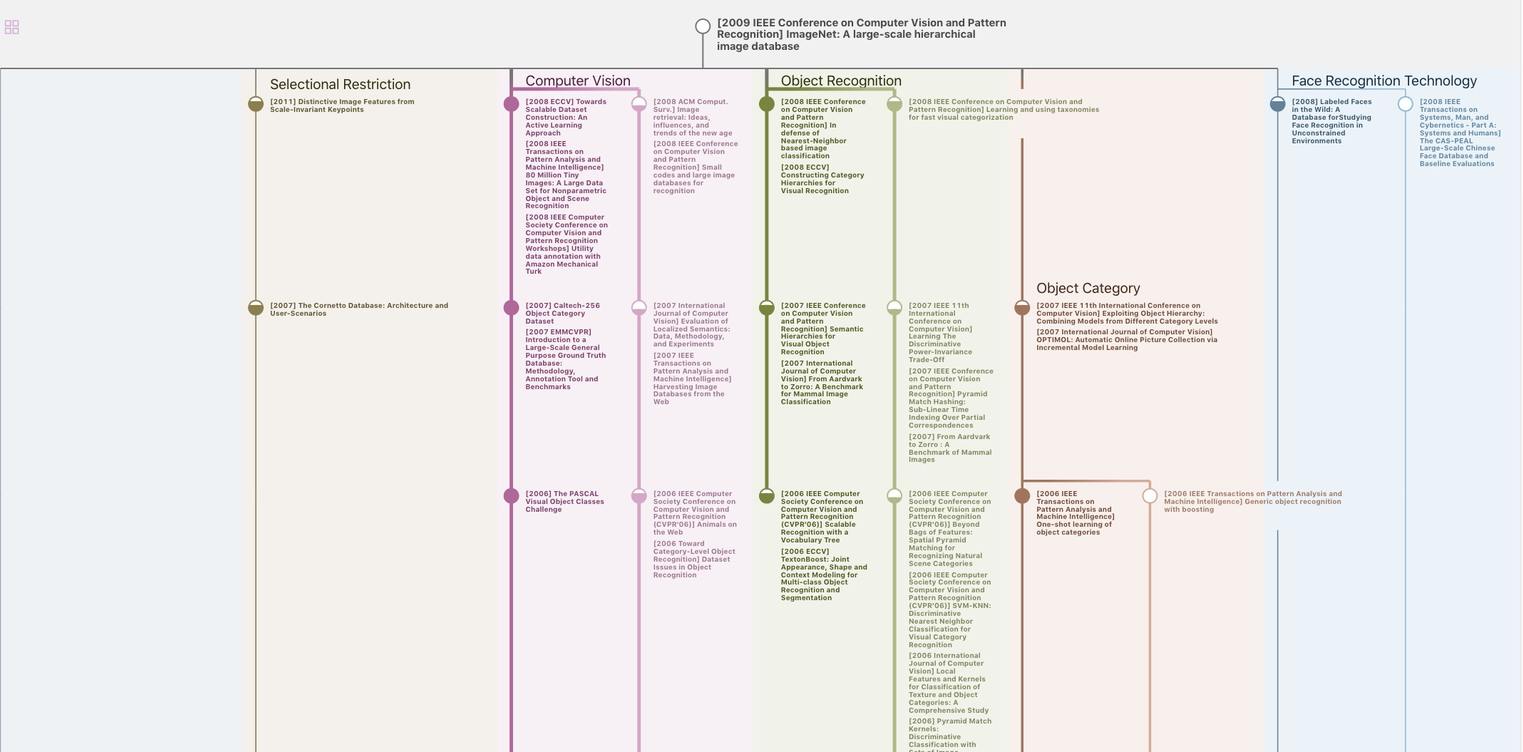
生成溯源树,研究论文发展脉络
Chat Paper
正在生成论文摘要