End-to-End Learning Framework for Space Optical Communications in Non-Differentiable Poisson Channel
IEEE WIRELESS COMMUNICATIONS LETTERS(2024)
Abstract
This letter studies an autoencoder (AE) model for point-to-point space optical communications (SOC) in the presence of an intensity modulation and direct detection setting wherein the optical link is modeled by a Poisson channel model. While the Poisson channel is a realistic channel model in SOC, its non-differentiable nature poses challenges when applied to deep learning. In this letter, a novel non-gradient-based optimization framework has been applied to estimating the channel gradient, addressing the non-differentiability of Poisson channels. Furthermore, our AE incorporates normalization layers in both encoders and decoders for input data standardization and efficient training convergence. The incorporation of the hyper-tuning optimization algorithm alongside the AE enhances the performance of the standard AE. This improvement stems from the effective minimization of the error cost function through gradient descent. The numerical results demonstrate that the proposed AE outperforms both state-of-the-art learning frameworks and model-based schemes in bit error rate (BER) performance within the Poisson channel.
MoreTranslated text
Key words
Optical transmitters,Photonics,Decoding,Channel estimation,Wireless communication,Training,Optimization,Free space optics,end-to-end learning,poisson channel,non-differentiable channels
AI Read Science
Must-Reading Tree
Example
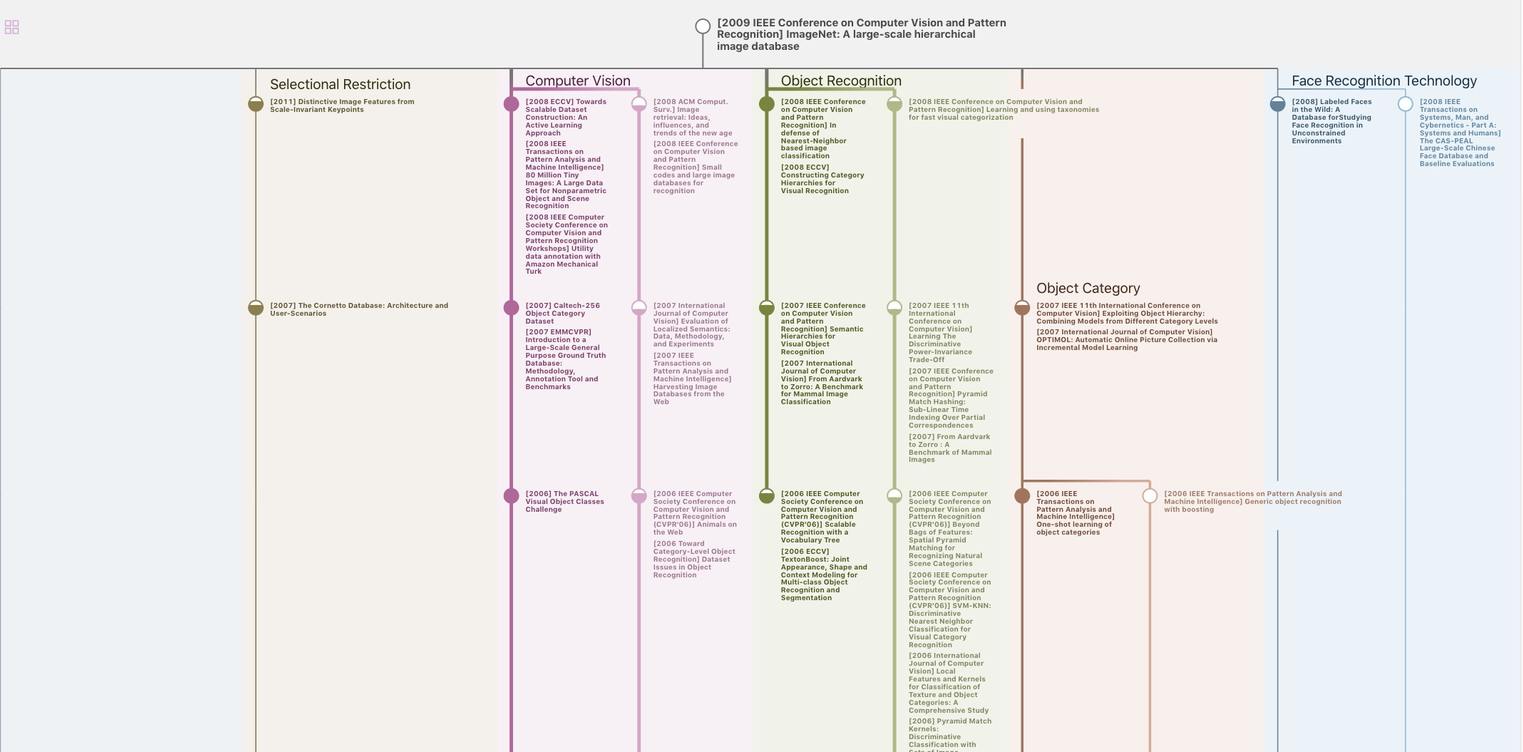
Generate MRT to find the research sequence of this paper
Chat Paper
Summary is being generated by the instructions you defined