DeepFedKDAD: Deep Federated Knowledge Distillation Based Anomaly Detection in B5G Network Slicing
2023 International Conference on Networks, Communications and Intelligent Computing (NCIC)(2023)
摘要
In order to support the various needs of future applications and different services anticipated in Beyond 5G (B5G) networks, B5G networks will rely on network slicing (NS), a key technology that creates multiple virtual networks on a shared physical infrastructure. As the number of user equipment (UE) connections accessing resources from the B5G NS is expected to increase, security and privacy concerns may arise from abnormal behaviors of these UEs. These security and privacy concerns can disrupt the performance of the B5G NS and impact the slices' ability to effectively provide resources and services to the UEs. Therefore, we propose a novel deep-federated knowledge distillation-based anomaly detection framework, named DeepFedKDAD, to address the se-curity and privacy concerns arising from anomalous UEs. The results show that our proposed anomaly detection framework outperformed baseline studies regarding accuracy, precision, recall and f1-score performance metrics in detecting anoma-lies. Additionally, the results showed that our DeepFedKDAD converges faster as compared to the baseline studies.
更多查看译文
关键词
B5G networks,Network slicing,Anomaly detection,Federated knowledge distillation,Security,Privacy
AI 理解论文
溯源树
样例
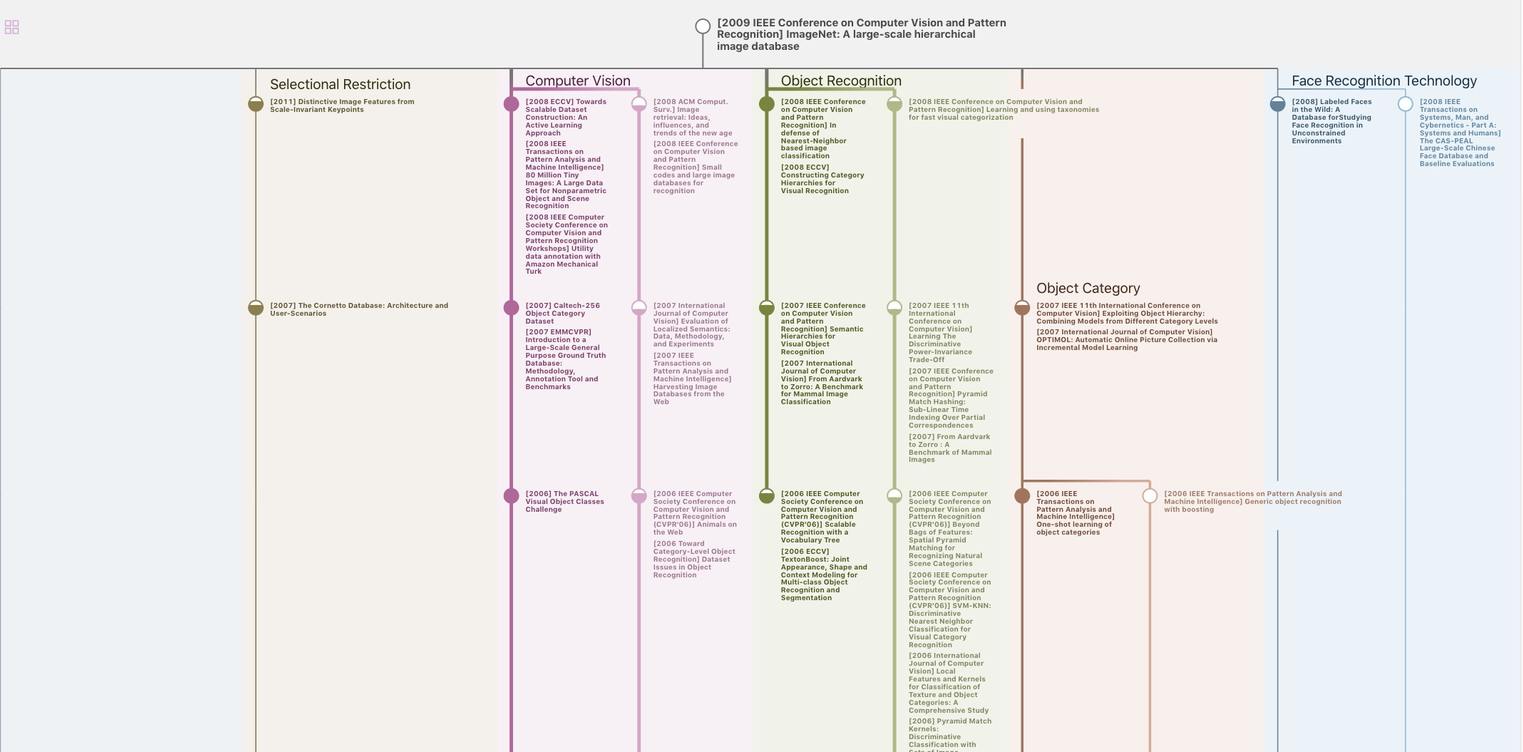
生成溯源树,研究论文发展脉络
Chat Paper
正在生成论文摘要