Expanding the Defect Image Dataset of Composite Material Coating with Enhanced Image-to-image Translation
Engineering Applications of Artificial Intelligence(2024)
摘要
In the defect detection based on machine vision, the limited datasets have consistently impeded the efficacy of deep learning in defect identification. Restricted datasets lead to inadequate model generalization and reduced detection accuracy. Creating simulated images is one proposed solution to address this challenge. However, existing defect image generation methods have limitations in diversity and realism. An enhanced Image-to-image translation method, named E-Pix2Pix, is proposed for enhancing defect image datasets of composite material coating on solid motors to expand the current dataset and enhance the precision of classification models. This method not only improves the quality of generated images, but also has strong generalization ability. This study examines how different convolution kernel sizes and the coefficient of the L1 loss function affect the generation performance. The results of the experiments show that the defect images generated by E-Pix2Pix have realistic visual effects and better capture the essential features of defects. The structure similarity index measurement (SSIM) value reaches approximately 0.9 under appropriate parameters. Moreover, this study intends to investigate how the content of generated images impacts the classification model. By doubling the dataset size using the generated images, the accuracy of ResNet50 model recognition can be improved by 7%. The accuracy of ResNet101 model recognition can be improved by 5% when the dataset is expanded to 1.5 times. The generated images have harmful effects on the weaker models. Our method has also been validated on two public datasets, and satisfactory results have been obtained.
更多查看译文
关键词
Generative adversarial networks,Image-to-image translation,Composite material,Defect detection
AI 理解论文
溯源树
样例
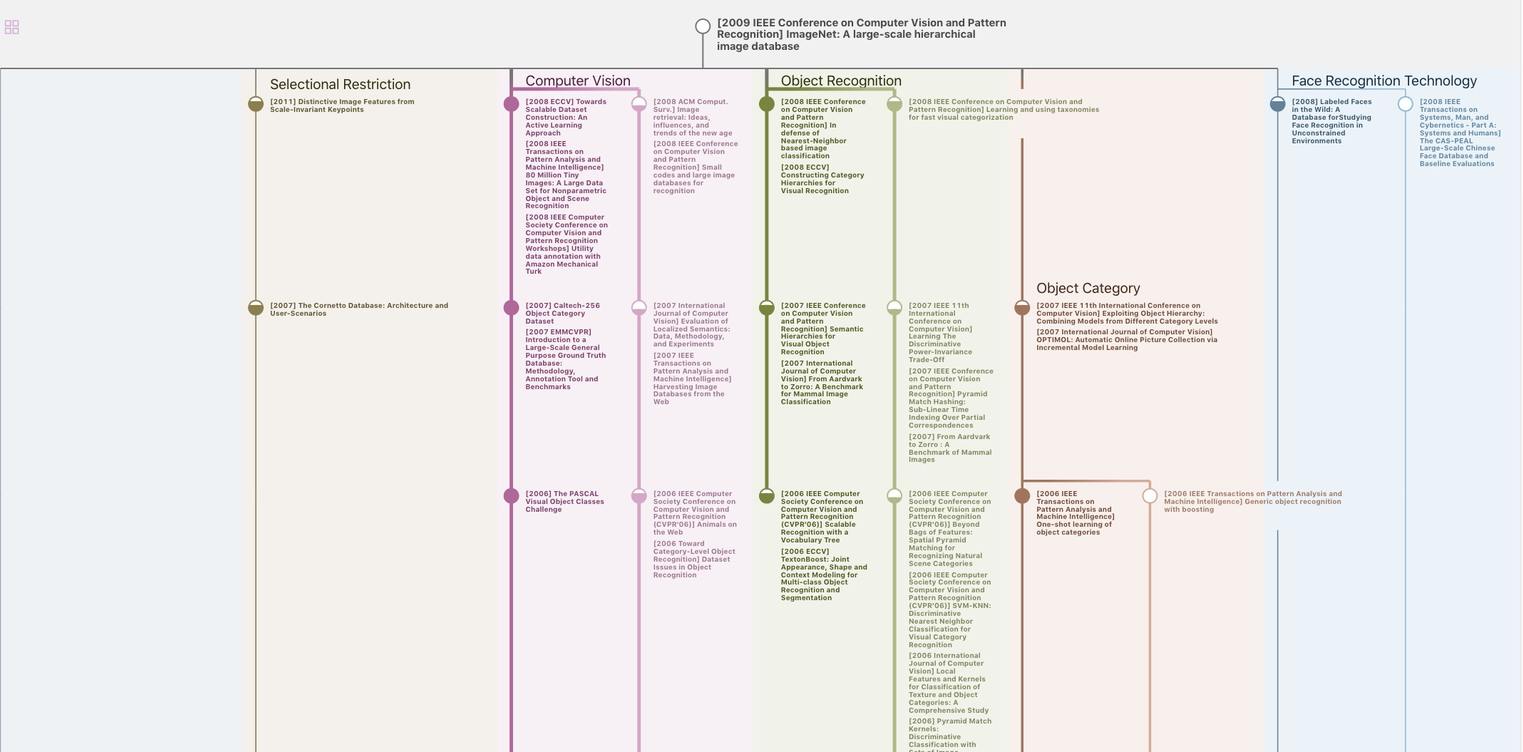
生成溯源树,研究论文发展脉络
Chat Paper
正在生成论文摘要