Toward a Machine Learning-Facilitated LES of Turbulent Flow Around a Solid Body
2024 IEEE/OES Thirteenth Current, Waves and Turbulence Measurement (CWTM)(2024)
摘要
In this research, we introduce a machine-learning framework aimed at refining the dynamic Smagorinsky model used in LES. This study analyzes two approaches: a multi-layer feedforward artificial neural network, which is applied in a point-wise fashion, and a convolutional neural network (CNN), which processes selectively chosen snapshots of fluid dynamics. The primary objective is to explore the potential of a data-driven methodology for the accurate determination of the Smagorinsky coefficient, which is crucial for effective turbulence modelling. Our machine learning models were rigorously tested by comparing their performance against the traditional dynamic Smagorinsky model, particularly in scenarios involving flow past a sphere at a Reynolds number of 10
3
. This comparison focused on evaluating different neural network architectures and their dependence on the volume of training data and the nature of the input variables used. We conducted a detailed analysis of the results, where the correlation between the true and predicted stress tensors was calculated to assess model accuracy. The cross-correlation coefficient demonstrated that the model achieves acceptable precision for simulations at a Reynolds number of 10
3
. Furthermore, our study highlights that the incorporation of velocity gradient information and resolved stress components into the convolutional neural networks significantly enhances the capability of the model to handle the LES of flows with massive separation.
更多查看译文
关键词
Subgrid-Scale (SGS),Machine Learning,Convolutional Neural Network (CNN),LES,Turbulent Flow
AI 理解论文
溯源树
样例
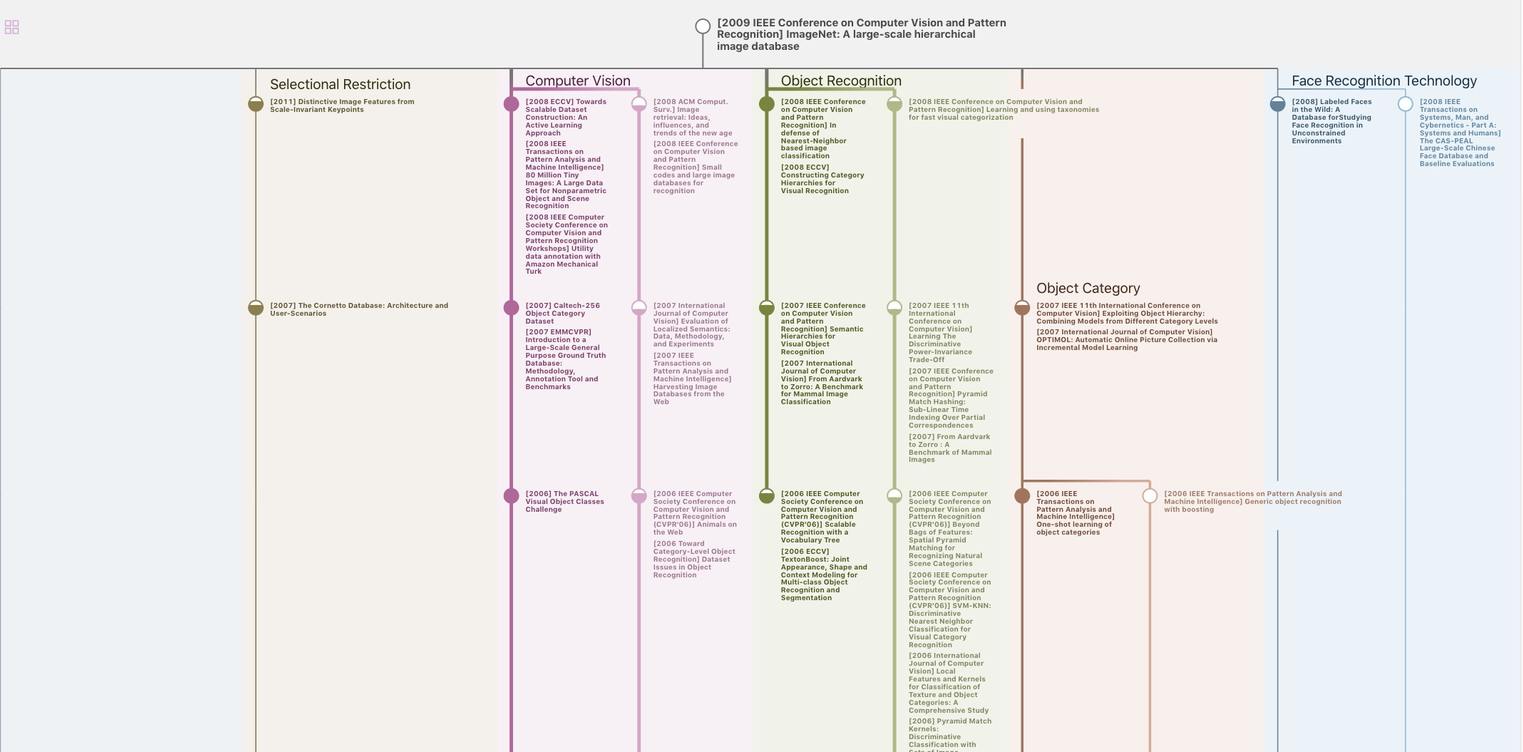
生成溯源树,研究论文发展脉络
Chat Paper
正在生成论文摘要