Integrating Regular Expressions into Neural Networks for Relation Extraction
Expert Syst Appl(2024)
摘要
Relation extraction aims to identify semantic relationships between entities from the given sentences. The development of deep learning prompts a variety of neural-based relation extraction models. These models can learn features from sentences automatically, but controlling their learned patterns is equally difficult. Therefore, it is hard to take steps to improve their performance further. A possible way to modify the models is by utilizing human-defined rules to introduce rule-based knowledge and guiding the models to capture the desired features based on human intent. As an efficient human knowledge, regular expression (RE) is helpful in extracting particular targets from text, which is also logical and interpretable. A novel embedding method is proposed to transform REs into learnable representations available to neural networks (NNs). Then, the rule-based representations are investigated to be integrated into different levels of NNs. Three representative relation extraction models based on recurrent neural network (RNN), convolutional neural network (CNN), and Transformer encoder, respectively, are selected as baselines to incorporate our rule-based representations. The experimental results show that the models with rule-based information outperform their original ones on the F1-score by 3.75%, 4.06%, and 2.57%, and the identification accuracy of complex samples is also significantly improved, verifying the effectiveness of our approaches. Our work is easy to reproduce and can be generally applied to various neural networks, proving that data-driven relation extraction methods can take advantage of the rule-based knowledge provided by Res
更多查看译文
关键词
Relation extraction,Regular expression,Rule-based representation,Neural networks
AI 理解论文
溯源树
样例
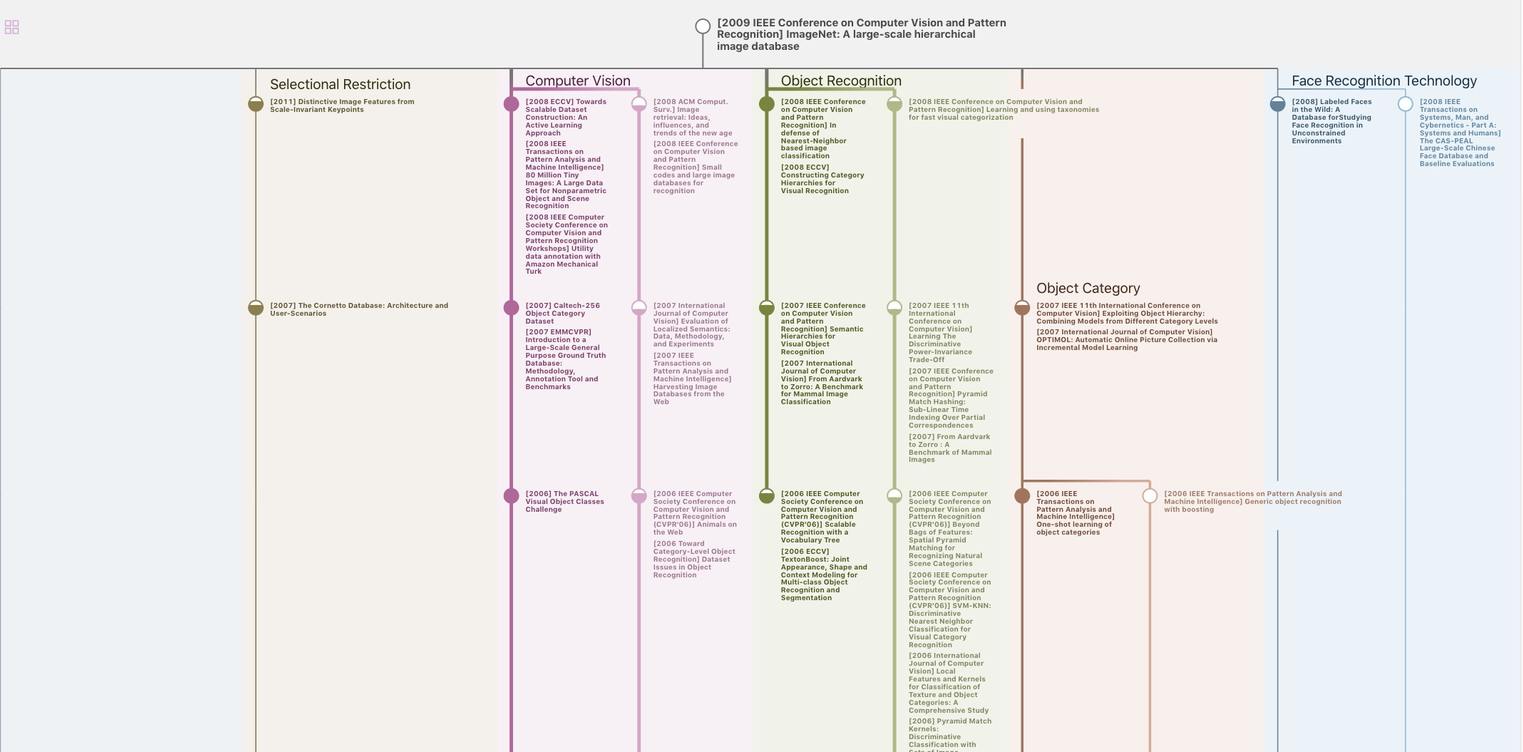
生成溯源树,研究论文发展脉络
Chat Paper
正在生成论文摘要