Two-Phase Sparsification with Secure Aggregation for Privacy-Aware Federated Learning
IEEE INTERNET OF THINGS JOURNAL(2024)
关键词
Servers,Computational modeling,Security,Training,Protocols,Machine learning algorithms,Aggregates,Communication-efficient,gradient sparsification,privacy-aware federated learning (FL),probabilistic subnetwork,secure aggregation (SA)
AI 理解论文
溯源树
样例
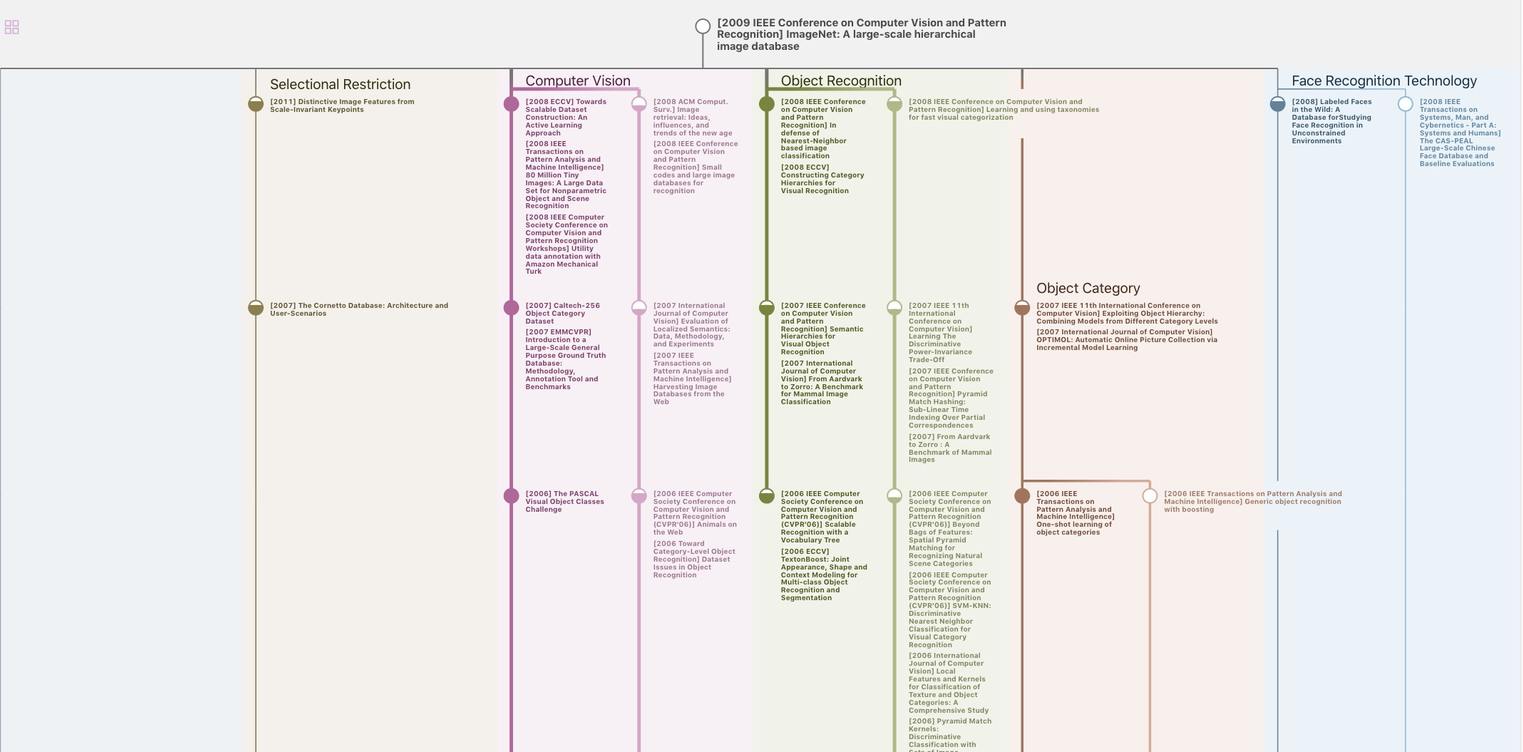
生成溯源树,研究论文发展脉络
Chat Paper
正在生成论文摘要