Development of a Machine Learning Model for Predicting Pneumothorax Risk in Coaxial Core Needle Biopsy (≤3 Cm)
European journal of radiology(2024)
摘要
Purpose: The aim is to devise a machine learning algorithm exploiting preoperative clinical data to forecast the hazard of pneumothorax post-coaxial needle lung biopsy (CCNB), thereby informing clinical decision-making and enhancing perioperative care. Method: This retrospective analysis aggregated clinical and imaging data from patients with lung nodules (<= 3 cm) biopsies. Variable selection was done using univariate analysis and LASSO regression, with the dataset subsequently divided into training (80 %) and validation (20 %) subsets. Various machine learning (ML) classifiers were employed in a consolidated approach to ascertain the paramount model, which was followed by individualized risk profiling showcased through Shapley Additive eXplanations (SHAP). Results: Out of the 325 patients included in the study, 19.6% (64/325) experienced postoperative pneumothorax. High-risk factors determined were Cancer, Lesion_type, GOLD, Size, and Depth. The Gaussian Naive Bayes (GNB) classifier demonstrated superior prediction with an Area Under the Curve (AUC) of 0.82 (95% CI 0.71-0.94), complemented by an accuracy rate of 0.8, sensitivity of 0.71, specificity of 0.84, and an F1 score of 0.61 in the test cohort. Conclusion: The formulated prognostic algorithm exhibited commendable efficacy in preoperatively prognosticating CCNB-induced pneumothorax, harboring the potential to refine personalized risk appraisals, steer clinical judgment, and ameliorate perioperative patient stewardship.
更多查看译文
关键词
Pneumothorax,Machine learning (ML),Coaxial core needle lung biopsy (CCNB),Prediction model,Risk factor
AI 理解论文
溯源树
样例
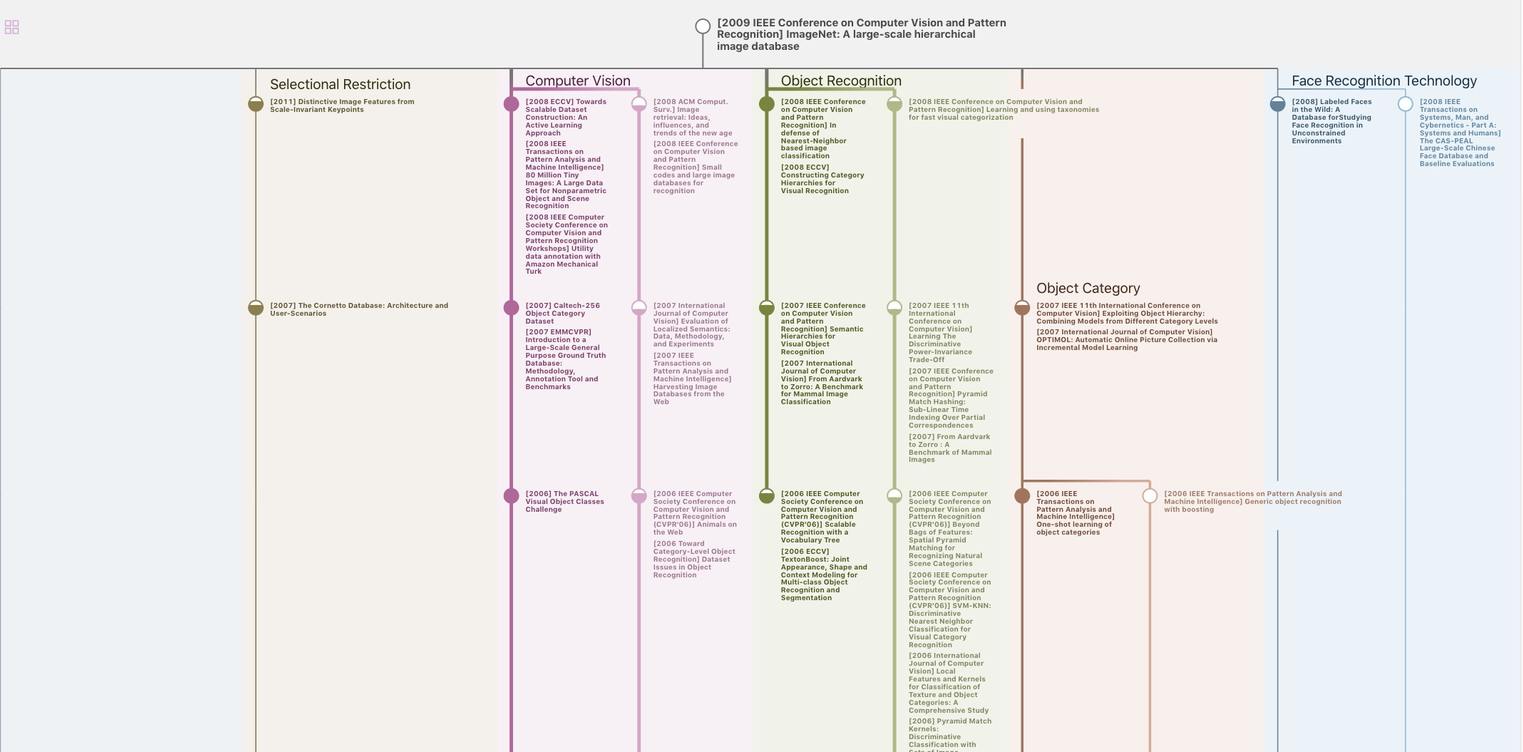
生成溯源树,研究论文发展脉络
Chat Paper
正在生成论文摘要