Leveraging Mobile NER for Real-Time Capture of Symptoms, Diagnoses, and Treatments from Clinical Dialogues
Informatics in medicine unlocked(2024)
摘要
In the dynamic world of healthcare technology, efficiently and accurately extracting medical data from physician-patient conversations is vital. This paper presents a new approach in healthcare technology, employing Natural Language Processing (NLP) to identify and extract critical information from doctor-patient conversations on mobile devices. Unlike traditional methods that rely on Electronic Health Records, our novel application enables the extraction of symptoms, diagnoses, and treatments directly on a mobile device during medical consultations, significantly enhancing patient privacy. We managed to integrate both Bidirectional Encoder Representations from Transformers (BERT) models and optimized Large Language Models (LLMs) on a mobile device without compromising performance significantly. Our findings reveal that the BERT model attained an F1-score of 85.1%, while FLERT and its compressed variant DistilFLERT showed superior performance. The FLAN-T5 model outperformed all models we tested with scores up to 92.7%. These results highlight the efficacy of leveraging advanced NLP and LLM technologies in healthcare environments on a mobile device, offering a promising direction for accessible and efficient patient care.
更多查看译文
关键词
Named Entity Recognition (NER),Medical data extraction,Distillation models for mobile,Electronic Health Records (EHR),Transformers,Large Language Models
AI 理解论文
溯源树
样例
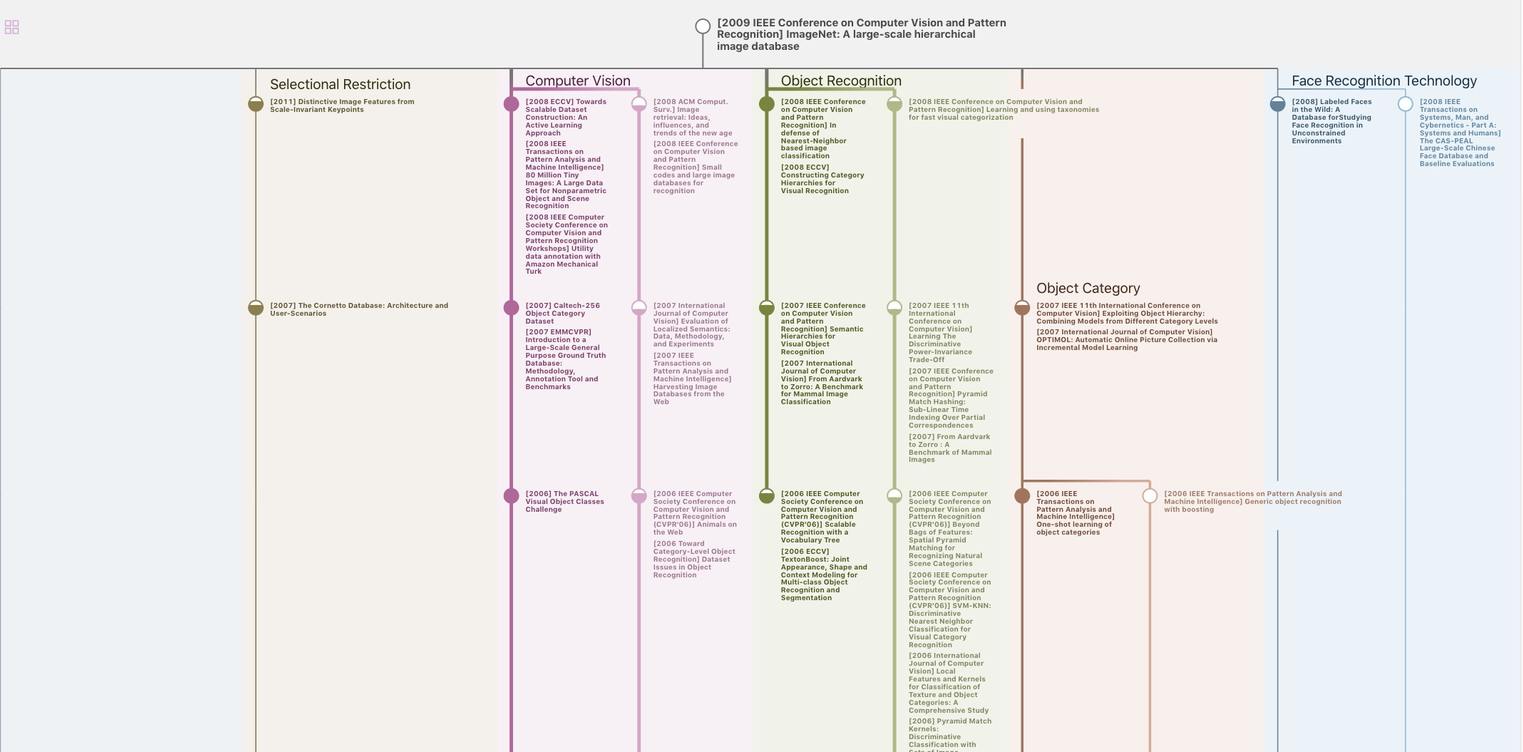
生成溯源树,研究论文发展脉络
Chat Paper
正在生成论文摘要