Self-distillation with Augmentation in Feature Space
IEEE Transactions on Circuits and Systems for Video Technology(2024)
摘要
Compared with traditional knowledge distillation, self-distillation does not require a pre-trained teacher network, which is more concise. Among them, data augmentation-based methods provide an elegant solution without modifying the network structure or additional memory consumption. However, when employing data augmentation in the input space, the forward propagations for augmented data bring additional computation costs and the augmentation methods need be adaptive to the modality of input data. Meanwhile, we note that from a generalization perspective, under the condition of being able to distinguish from other classes, a dispersed intra-class feature distribution is superior to compact intra-class feature distribution, especially for categories with larger sample differences. Based on the above considerations, this paper proposes a feature augmentation based self-distillation method (FASD) based on the idea of feature extrapolation. For each source feature, two augmentations are generated by subtraction between features. The one is subtracting the temporary class center computed with samples belonging to the same category, and another one is subtracting a sample feature belonging to other categories with the closest distance. Then, the predicted outputs of the augmented features are constrained to be consistent with that of the source feature. The consistent constraint on the previous augmented feature expands the learned class feature distribution, leading to greater overlap with the unknown feature distribution of test samples, thereby improving the generalization performance of the network. The consistent constraint on the latter augmented feature increases the distance between samples from different categories, which enhances the distinguishability between categories. Experimental results on image classification task demonstrate the effectiveness and efficiency of the proposed method. Meanwhile, experiments on text and audio tasks prove the universality of the method for classification tasks with different modalities.
更多查看译文
关键词
Knowledge distillation,Self-distillation,Classification task,Feature augmentation,Generalization performance
AI 理解论文
溯源树
样例
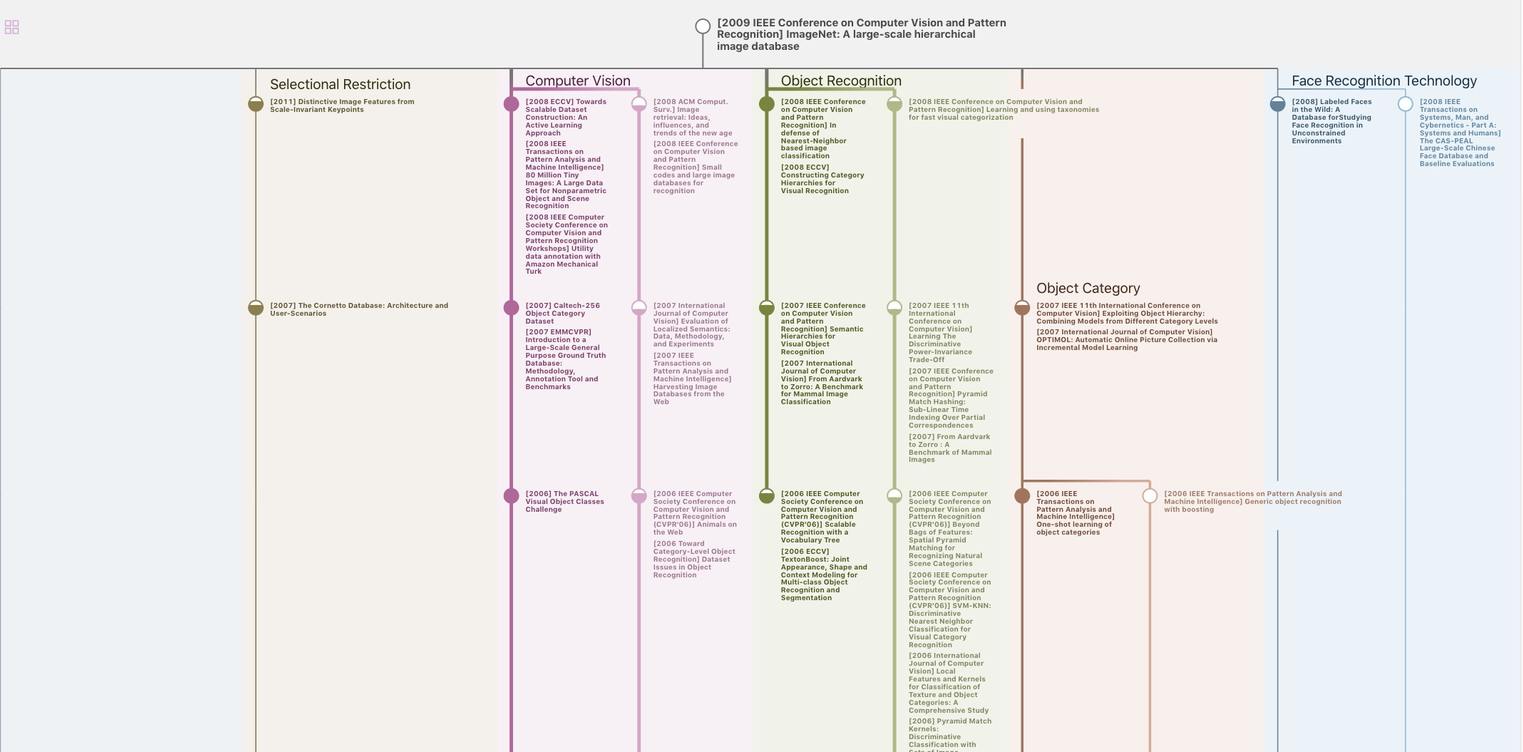
生成溯源树,研究论文发展脉络
Chat Paper
正在生成论文摘要