Decentralized Kernel Ridge Regression Based on Data-Dependent Random Feature
IEEE TRANSACTIONS ON NEURAL NETWORKS AND LEARNING SYSTEMS(2024)
Abstract
Random feature (RF) has been widely used for node consistency in decentralized kernel ridge regression (KRR). Currently, the consistency is guaranteed by imposing constraints on coefficients of features, necessitating that the RFs on different nodes are identical. However, in many applications, data on different nodes vary significantly on the number or distribution, which calls for adaptive and data-dependent methods that generate different RFs. To tackle the essential difficulty, we propose a new decentralized KRR algorithm that pursues consensus on decision functions, which allows great flexibility and well adapts data on nodes. The convergence is rigorously given, and the effectiveness is numerically verified: by capturing the characteristics of the data on each node, while maintaining the same communication costs as other methods, we achieved an average regression accuracy improvement of 25.5% across six real-world datasets.
MoreTranslated text
Key words
Radio frequency,Kernel,Convergence,Learning systems,Distributed databases,Costs,Approximation algorithms,Data-dependent algorithm,decentralized learning,kernel methods,random feature (RF)
AI Read Science
Must-Reading Tree
Example
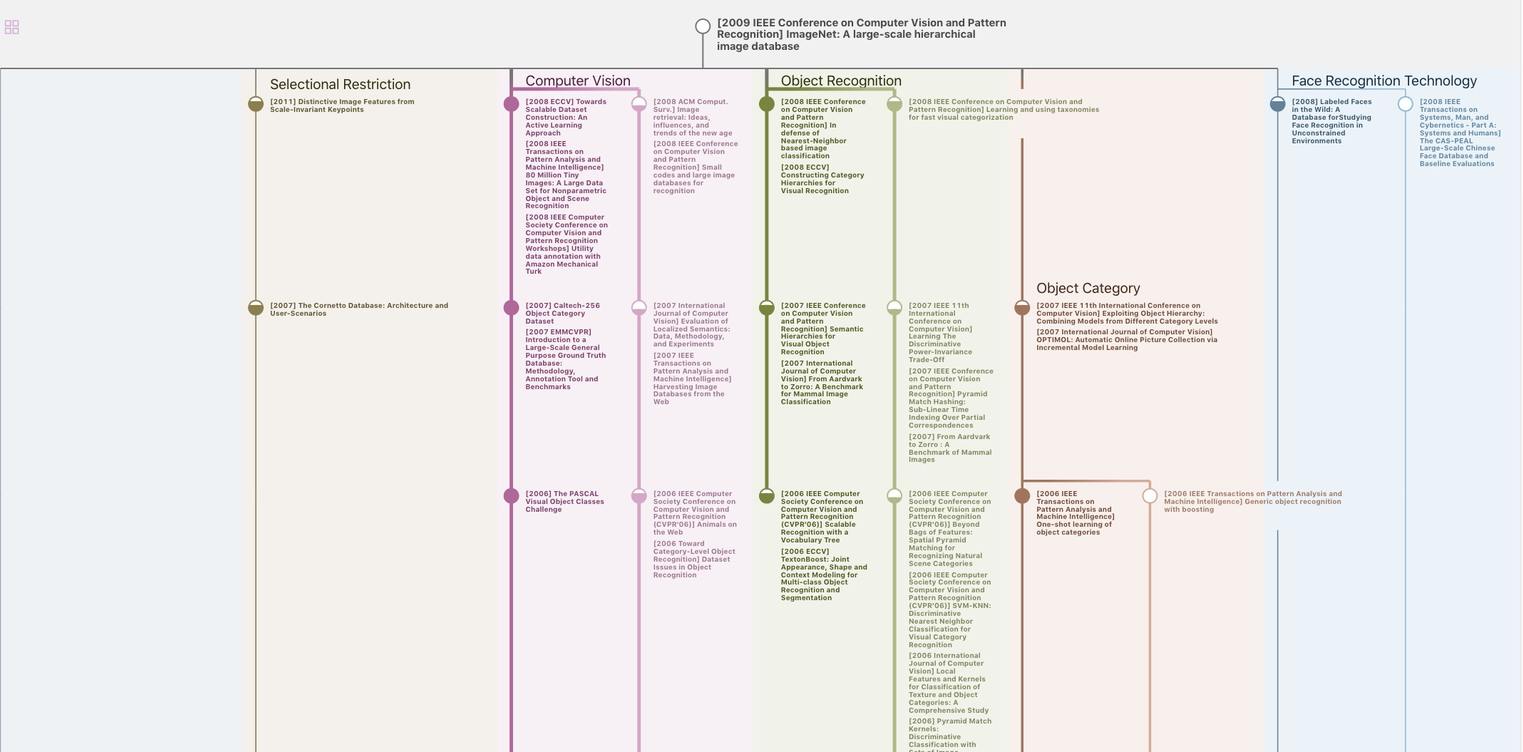
Generate MRT to find the research sequence of this paper
Chat Paper
Summary is being generated by the instructions you defined